For Honor, for Toxicity: Detecting Toxic Behavior through Gameplay
Proceedings of the ACM on Human-Computer Interaction(2021)
摘要
AbstractIs it possible to detect toxicity in games just by observing in-game behavior? If so, what are the behavioral factors that will help machine learning to discover the unknown relationship between gameplay and toxic behavior? In this initial study, we examine whether it is possible to predict toxicity in the MOBA gameFor Honor by observing in-game behavior for players that have been labeled as toxic (i.e. players that have been sanctioned by Ubisoft community managers). We test our hypothesis of detecting toxicity through gameplay with a dataset of almost 1,800 sanctioned players, and comparing these sanctioned players with unsanctioned players. Sanctioned players are defined by their toxic action type (offensive behavior vs. unfair advantage) and degree of severity (warned vs. banned). Our findings, based on supervised learning with random forests, suggest that it is not only possible to behaviorally distinguish sanctioned from unsanctioned players based on selected features of gameplay; it is also possible to predict both the sanction severity (warned vs. banned) and the sanction type (offensive behavior vs. unfair advantage). In particular, all random forest models predict toxicity, its severity, and type, with an accuracy of at least 82%, on average, on unseen players. This research shows that observing in-game behavior can support the work of community managers in moderating and possibly containing the burden of toxic behavior.
更多查看译文
关键词
labeled dataset,machine learning,random forest,toxicity,video games
AI 理解论文
溯源树
样例
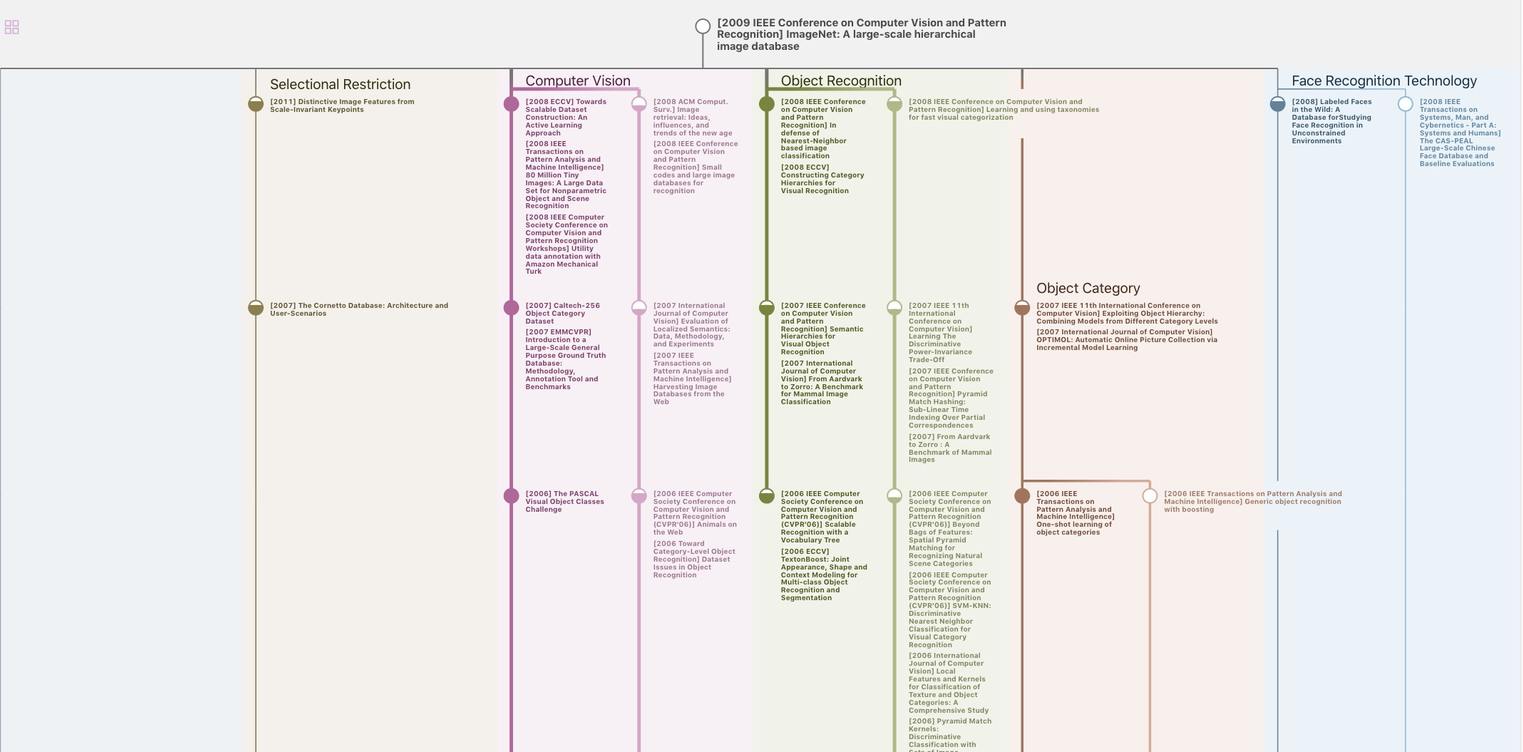
生成溯源树,研究论文发展脉络
Chat Paper
正在生成论文摘要