A Fault Diagnosis And Prognosis Method For Lithium-Ion Batteries Based On A Nonlinear Autoregressive Exogenous Neural Network And Boxplot
SYMMETRY-BASEL(2021)
Abstract
The frequent occurrence of electric vehicle fire accidents reveals the safety hazards of batteries. When a battery fails, its symmetry is broken, which results in a rapid degradation of its safety performance and poses a great threat to electric vehicles. Therefore, accurate battery fault diagnoses and prognoses are the key to ensuring the safe and durable operation of electric vehicles. Thus, in this paper, we propose a new fault diagnosis and prognosis method for lithium-ion batteries based on a nonlinear autoregressive exogenous (NARX) neural network and boxplot for the first time. Firstly, experiments are conducted under different temperature conditions to guarantee the diversity of the data of lithium-ion batteries and then to ensure the accuracy of the fault diagnosis and prognosis at different working temperatures. Based on the collected voltage and current data, the NARX neural network is then used to accurately predict the future battery voltage. A boxplot is then used for the battery fault diagnosis and early warning based on the predicted voltage. Finally, the experimental results (in a new dataset) and a comparative study with a back propagation (BP) neural network not only validate the high precision, all-climate applicability, strong robustness and superiority of the proposed NARX model but also verify the fault diagnosis and early warning ability of the boxplot. In summary, the proposed fault diagnosis and prognosis approach is promising in real electric vehicle applications.
MoreTranslated text
Key words
electric vehicles, lithium-ion batteries, fault diagnosis and prognosis, nonlinear autoregressive exogenous neural network, boxplot
AI Read Science
Must-Reading Tree
Example
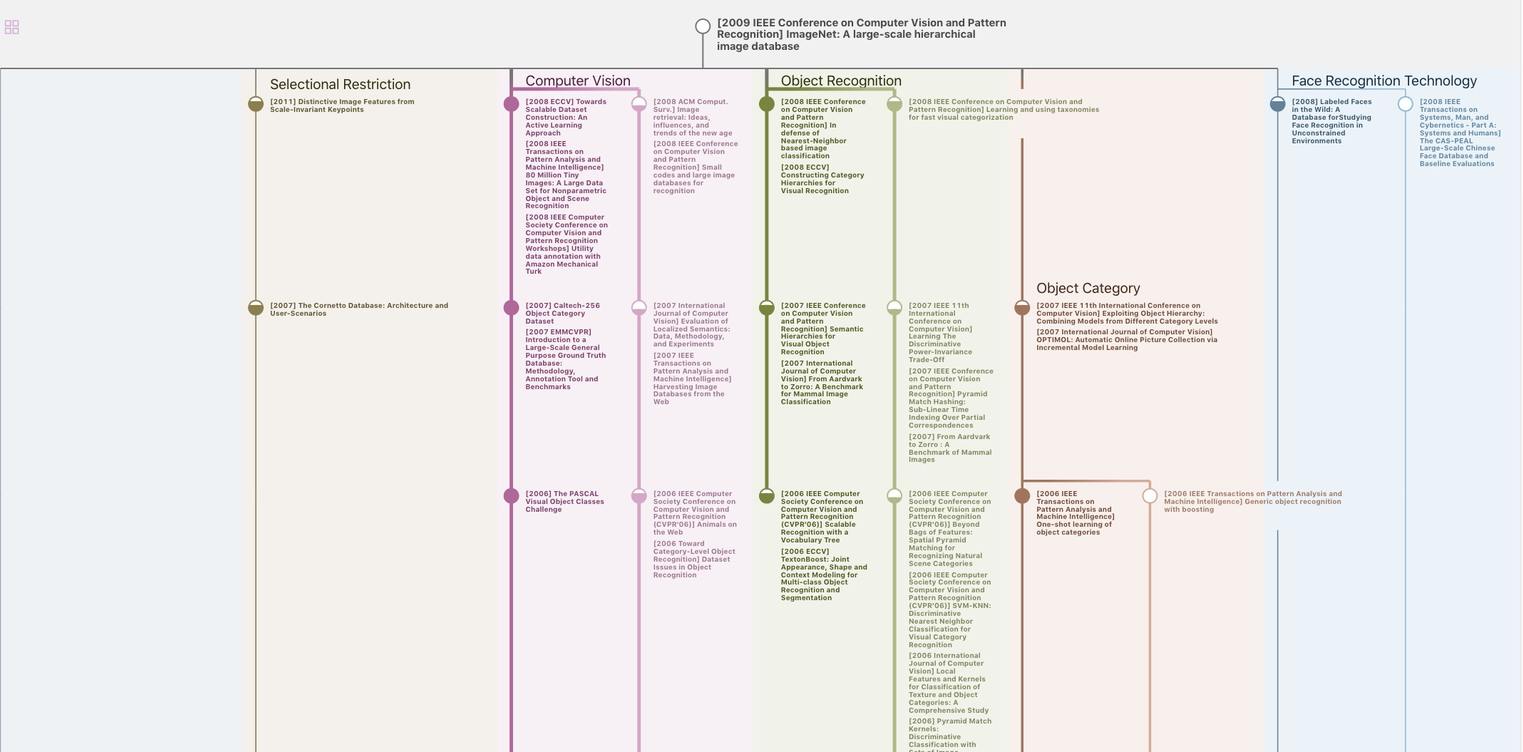
Generate MRT to find the research sequence of this paper
Chat Paper
Summary is being generated by the instructions you defined