EMBEDR: Distinguishing signal from noise in single-cell omics data
Patterns(2022)
摘要
Single-cell “omics”-based measurements are often high dimensional so that dimensionality reduction (DR) algorithms are necessary for data visualization and analysis. The lack of methods for separating signal from noise in DR outputs has limited their utility in generating data-driven discoveries in single-cell data. In this work we present EMBEDR, which assesses the output of any DR algorithm to distinguish evidence of structure from algorithm-induced noise in DR outputs. We apply EMBEDR to DR-generated representations of single-cell omics data of several modalities to show where they visually show real—not spurious—structure. EMBEDR generates a “p” value for each sample, allowing for direct comparisons of DR algorithms and facilitating optimization of algorithm hyperparameters. We show that the scale of a sample’s neighborhood can thus be determined and used to generate a novel “cell-wise optimal” embedding. EMBEDR is available as a Python package for immediate use.
更多查看译文
关键词
dimensionality reduction,single-cell analysis,data visualization,quality assessment,clustering,t-SNE,UMAP,single-cell RNA sequencing,ATAC-seq,cell-type identification
AI 理解论文
溯源树
样例
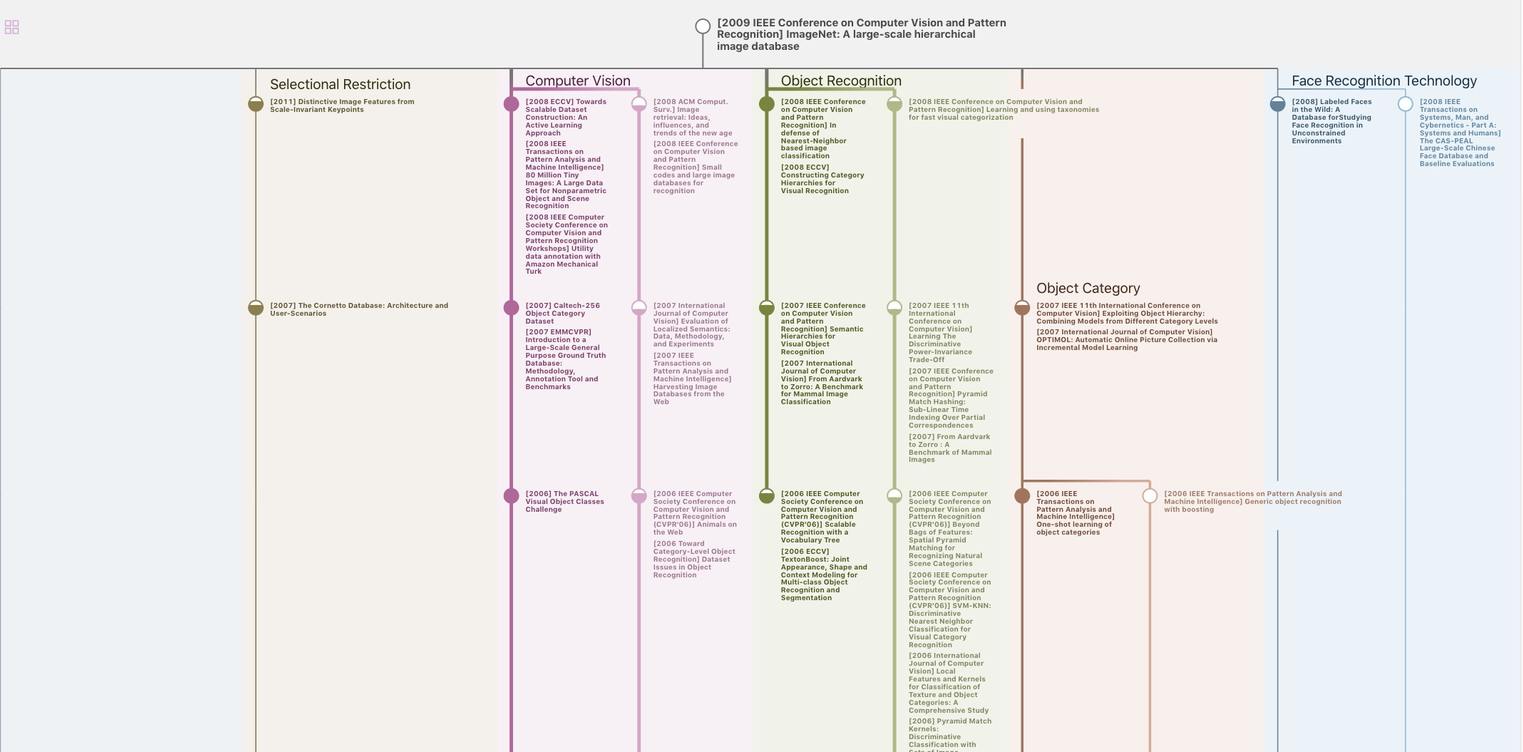
生成溯源树,研究论文发展脉络
Chat Paper
正在生成论文摘要