DeepDrug: A general graph-based deep learning framework for drug relation prediction
biorxiv(2020)
摘要
Computational approaches for accurate predictions of drug-related interactions such as drug-drug interactions (DDIs) and drug-target interactions (DTIs) are highly demanding for biochemical researchers due to their efficiency and cost-effectiveness. Despite the fact that many methods have been proposed and developed to predict DDIs and DTIs respectively, their success is still limited due to a lack of systematic evaluation of the intrinsic properties embedded in their structure. In this paper, we develop a deep learning framework, named DeepDrug, to overcome these shortcomings by using graph convolutional networks to learn the graphical representations of drugs and proteins such as molecular fingerprints and residual structures in order to boost the prediction accuracy. We benchmark our methods in binary-class DDIs, multi-class DDIs and binary-class DTIs classification tasks using several datasets. We then demonstrate that DeepDrug outperforms other state-of-the-art published methods both in terms of accuracy and robustness in predicting DDIs and DTIs with varying ratios of positive to negative training data. Ultimately, we visualize the structural features learned by DeepDrug, which display compatible and accordant patterns in chemical properties, providing additional evidence to support the strong predictive power of DeepDrug. We believe that DeepDrug is an efficient tool in accurate prediction of DDIs and DTIs and provides a promising path in understanding the underlying mechanism of these biochemical relations. The source code of the DeepDrug can be downloaded from .
### Competing Interest Statement
The authors have declared no competing interest.
更多查看译文
AI 理解论文
溯源树
样例
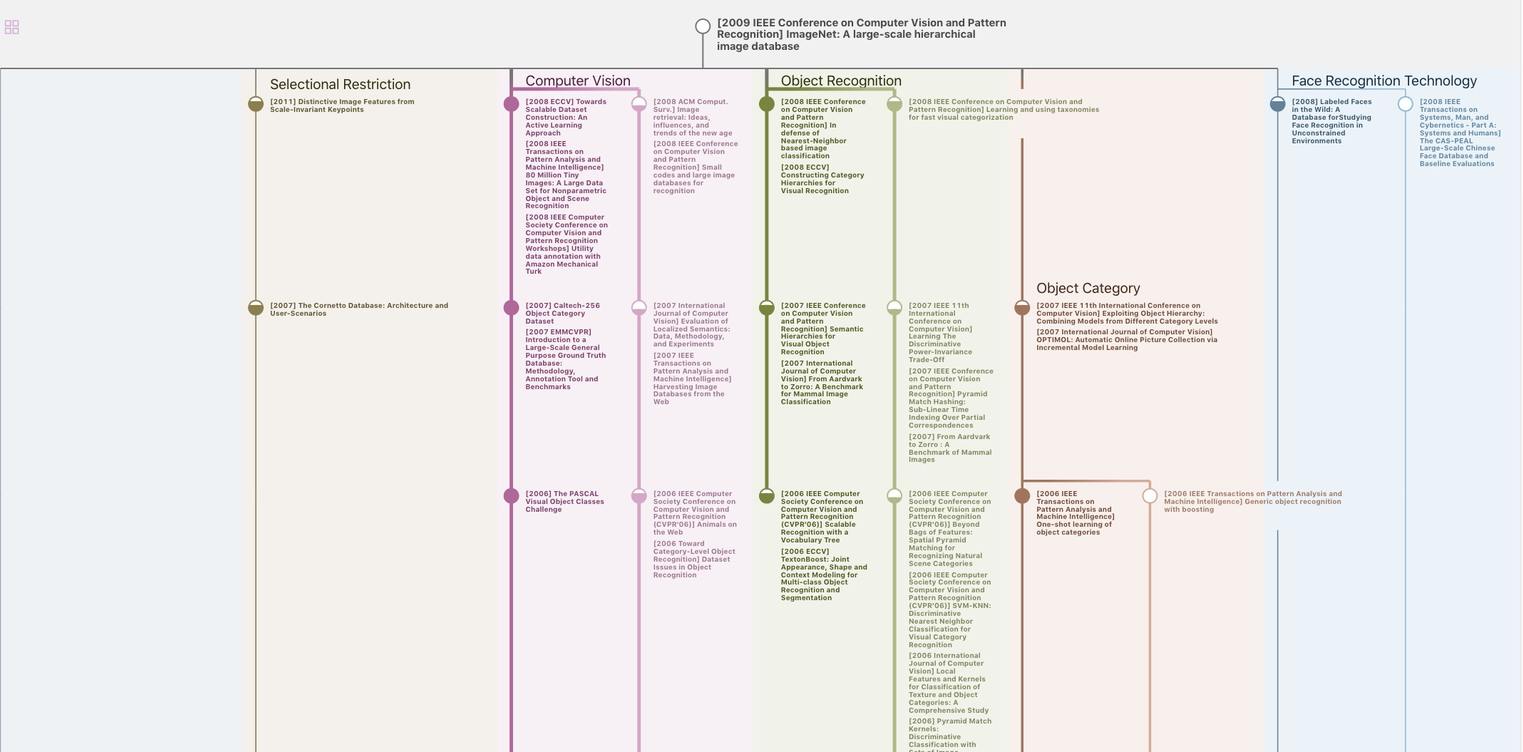
生成溯源树,研究论文发展脉络
Chat Paper
正在生成论文摘要