Bayesian Encoding and Decoding as Distinct Perspectives on Neural Coding
biorxiv(2021)
摘要
One of the most influential, and controversial, ideas in neuroscience has been to understand the brain in terms of Bayesian computations. Unstated differences in how Bayesian ideas are operationalized across different models make it difficult to ascertain both which empirical data support which models, and how Bayesian computations might be implemented by neural circuits. In this paper, we make one such difference explicit by identifying two distinct philosophies that underlie existing neural models of Bayesian inference: one in which the brain recovers experimenter-defined structures in the world from sensory neural activity (Decoding), and another in which the brain represents latent quantities in an internal model (Encoding). These philosophies require profoundly different assumptions about the nature of inference in the brain, and lead to different interpretations of empirical data. Here, we characterize and contrast both philosophies in terms of motivations, empirical support, and relationship to neural responses. We also show that this implicit difference in philosophy underlies some of the debate on whether neural activity is better described as a sampling-based, or a parametric, distributional code. Using a simple model of primary visual cortex as an example, we show mathematically that probabilistic inference by neural sampling in the encoding framework is, in fact, indistinguishable from a linear probabilistic population code (PPC) in the decoding framework. In sum, Bayesian Encoding and Bayesian Decoding are distinct, non-exclusive philosophies, and appreciating their similarities and differences will help organize future work on the nature of inference in the brain.
### Competing Interest Statement
The authors have declared no competing interest.
更多查看译文
关键词
decoding,encoding
AI 理解论文
溯源树
样例
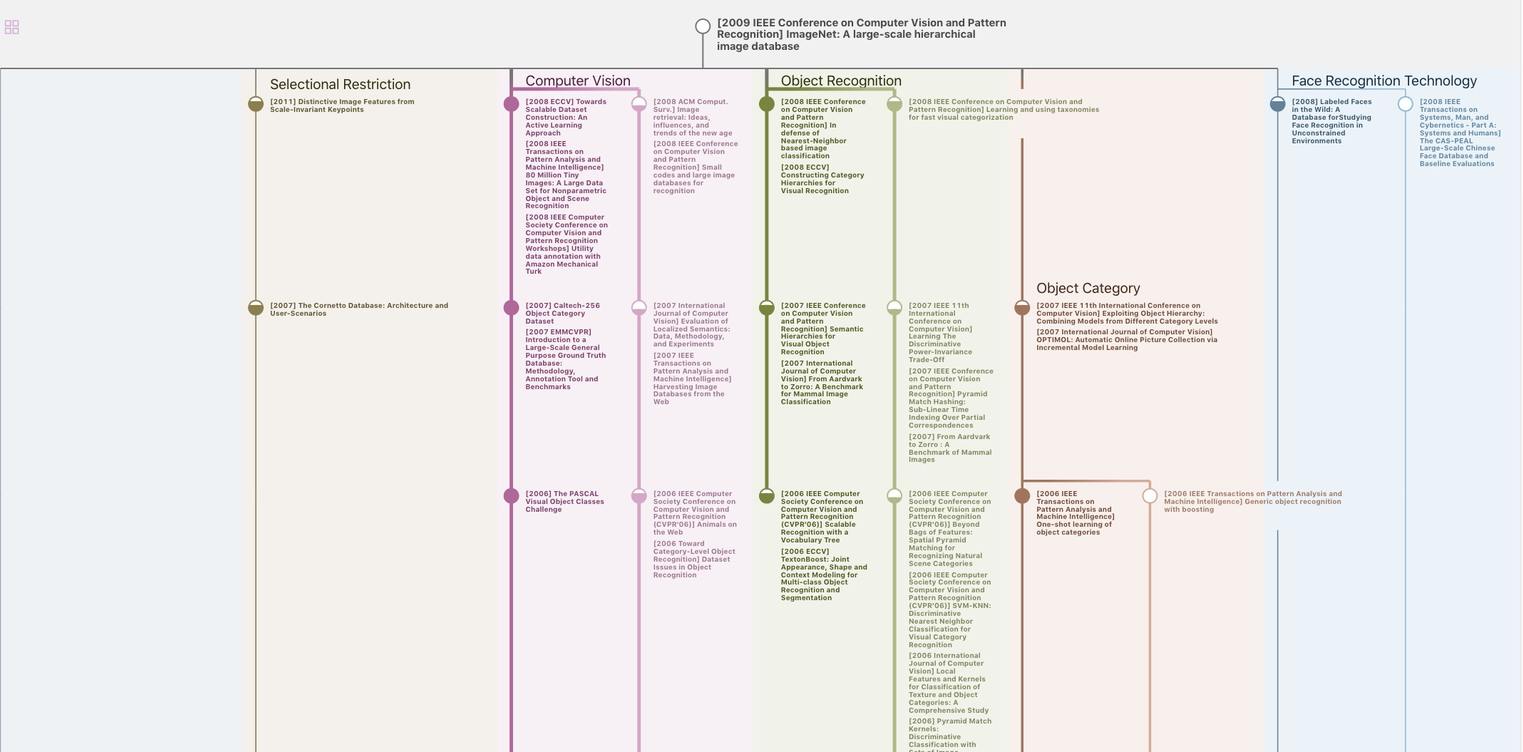
生成溯源树,研究论文发展脉络
Chat Paper
正在生成论文摘要