FLSys: Toward an Open Ecosystem for Federated Learning Mobile Apps
IEEE TRANSACTIONS ON MOBILE COMPUTING(2024)
摘要
This article presents the design, implementation, and evaluation of FLSys, a mobile-cloud federated learning (FL) system, which can be a key component for an open ecosystem of FL models and apps. FLSys is designed to work on smart phones with mobile sensing data. It balances model performance with resource consumption, tolerates communication failures, and achieves scalability. In FLSys, different DL models with different FL aggregation methods can be trained and accessed concurrently by different apps. Furthermore, FLSys provides advanced privacy preserving mechanisms and a common API for third-party app developers to access FL models. FLSys adopts a modular design and is implemented in Android and AWS cloud. We co-designed FLSys with a human activity recognition (HAR) model. HAR sensing data was collected in the wild from 100+ college students during a 4-month period. We implemented HAR-Wild, a CNN model tailored to mobile devices, with a data augmentation mechanism to mitigate the problem of non-Independent and Identically Distributed data. A sentiment analysis model is also used to demonstrate that FLSys effectively supports concurrent models. This article reports our experience and lessons learned from conducting extensive experiments using simulations, Android/Linux emulations, and Android phones that demonstrate FLSys achieves good model utility and practical system performance.
更多查看译文
关键词
Data models,Training,Smart phones,Biological system modeling,Sensors,Servers,Computational modeling,Federated learning,mobile sensing,smart phones
AI 理解论文
溯源树
样例
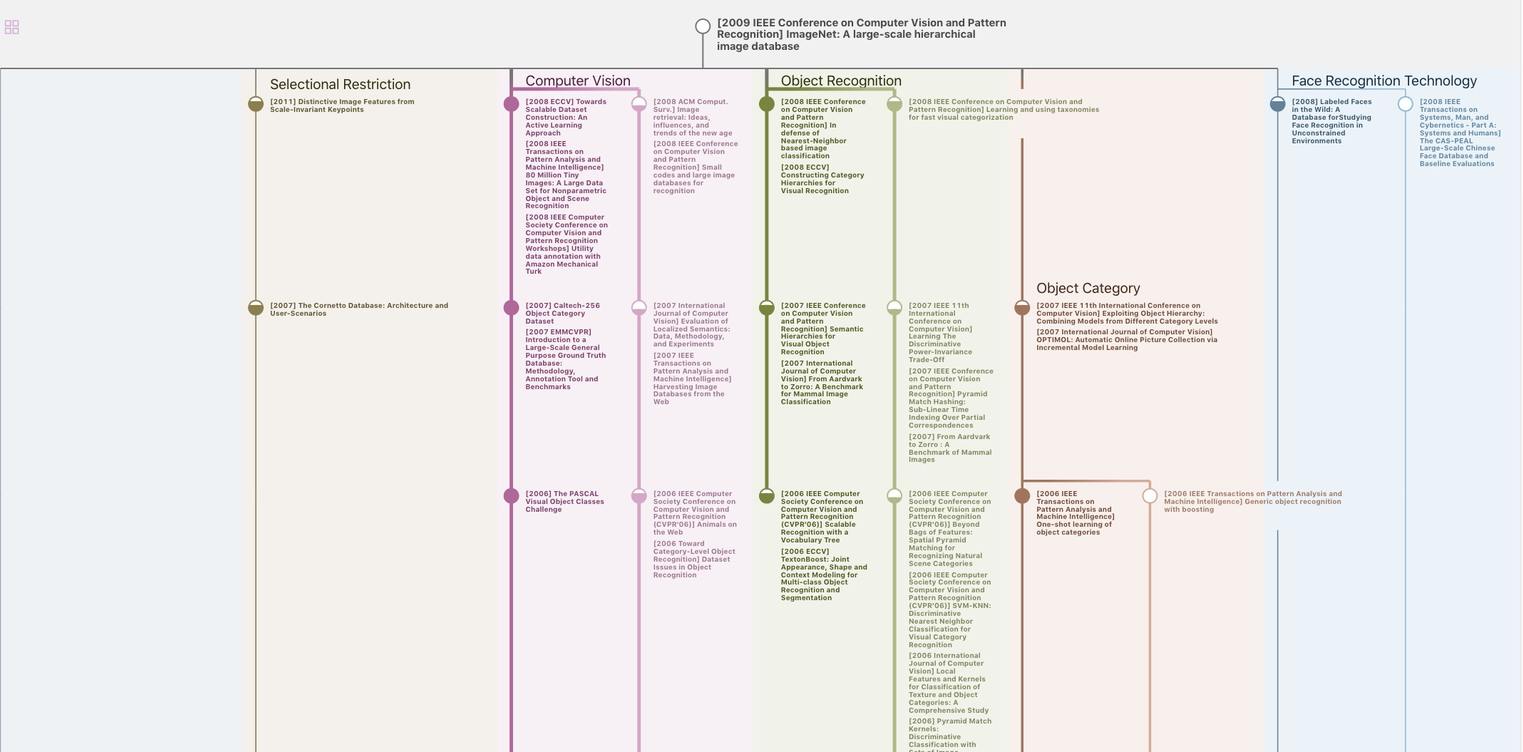
生成溯源树,研究论文发展脉络
Chat Paper
正在生成论文摘要