Green CWS: Extreme Distillation and Efficient Decode Method Towards Industrial Application
CoRR(2021)
摘要
Benefiting from the strong ability of the pre-trained model, the research on Chinese Word Segmentation (CWS) has made great progress in recent years. However, due to massive computation, large and complex models are incapable of empowering their ability for industrial use. On the other hand, for low-resource scenarios, the prevalent decode method, such as Conditional Random Field (CRF), fails to exploit the full information of the training data. This work proposes a fast and accurate CWS framework that incorporates a light-weighted model and an upgraded decode method (PCRF) towards industrially low-resource CWS scenarios. First, we distill a Transformer-based student model as an encoder, which not only accelerates the inference speed but also combines open knowledge and domain-specific knowledge. Second, the perplexity score to evaluate the language model is fused into the CRF module to better identify the word boundaries. Experiments show that our work obtains relatively high performance on multiple datasets with as low as 14\% of time consumption compared with the original BERT-based model. Moreover, under the low-resource setting, we get superior results in comparison with the traditional decoding methods.
更多查看译文
关键词
extreme distillation,efficient decode method
AI 理解论文
溯源树
样例
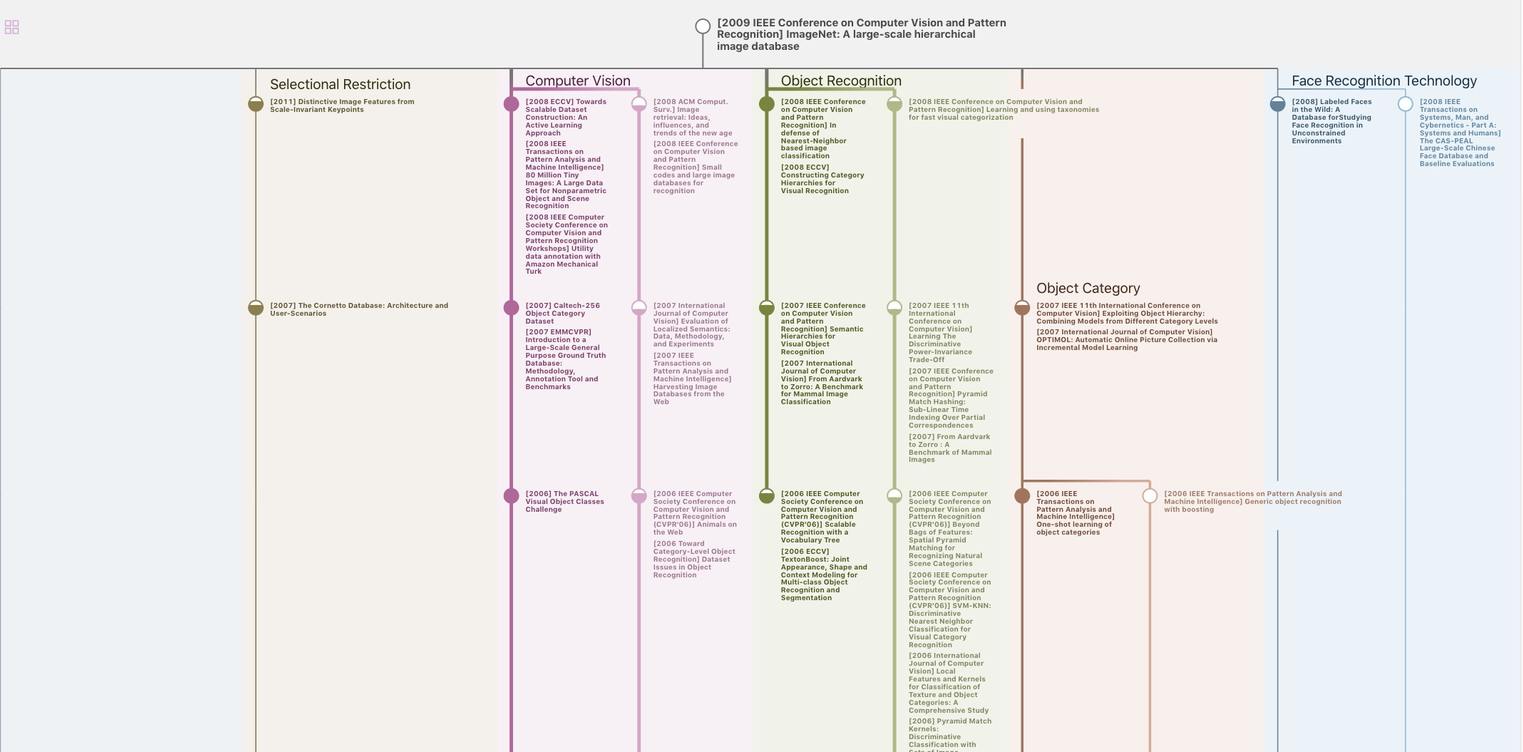
生成溯源树,研究论文发展脉络
Chat Paper
正在生成论文摘要