A robust two-step adversarial debiasing with partial learning - medical image case-studies
MEDICAL IMAGING 2023(2023)
摘要
The use of artificial intelligence (AI) in healthcare has become a very active research area in the last few years. While significant progress has been made in image classification tasks, only a few AI methods are actually deployed in clinical settings. A major hurdle in actively using clinical AI models is the trustworthiness of these models. Often, these complex models are utilized as black boxes in which promising results are generated. However, when scrutinized, these models reveal implicit biases during decision-making, such as having an unintended bias towards particular ethnic groups and sub-populations. In our study, we develop a two-step adversarial debiasing approach with partial learning that can reduce the disparity while preserving the performance of the targeted diagnosis/classification task. The methodology has been evaluated on two independent medical image case studies - chest X-rays and mammograms and showed promises in bias reduction while preserving the targeted performance on both internal and external datasets.
更多查看译文
关键词
Adversarial Debiasing, Fairness, X-ray, Mammography
AI 理解论文
溯源树
样例
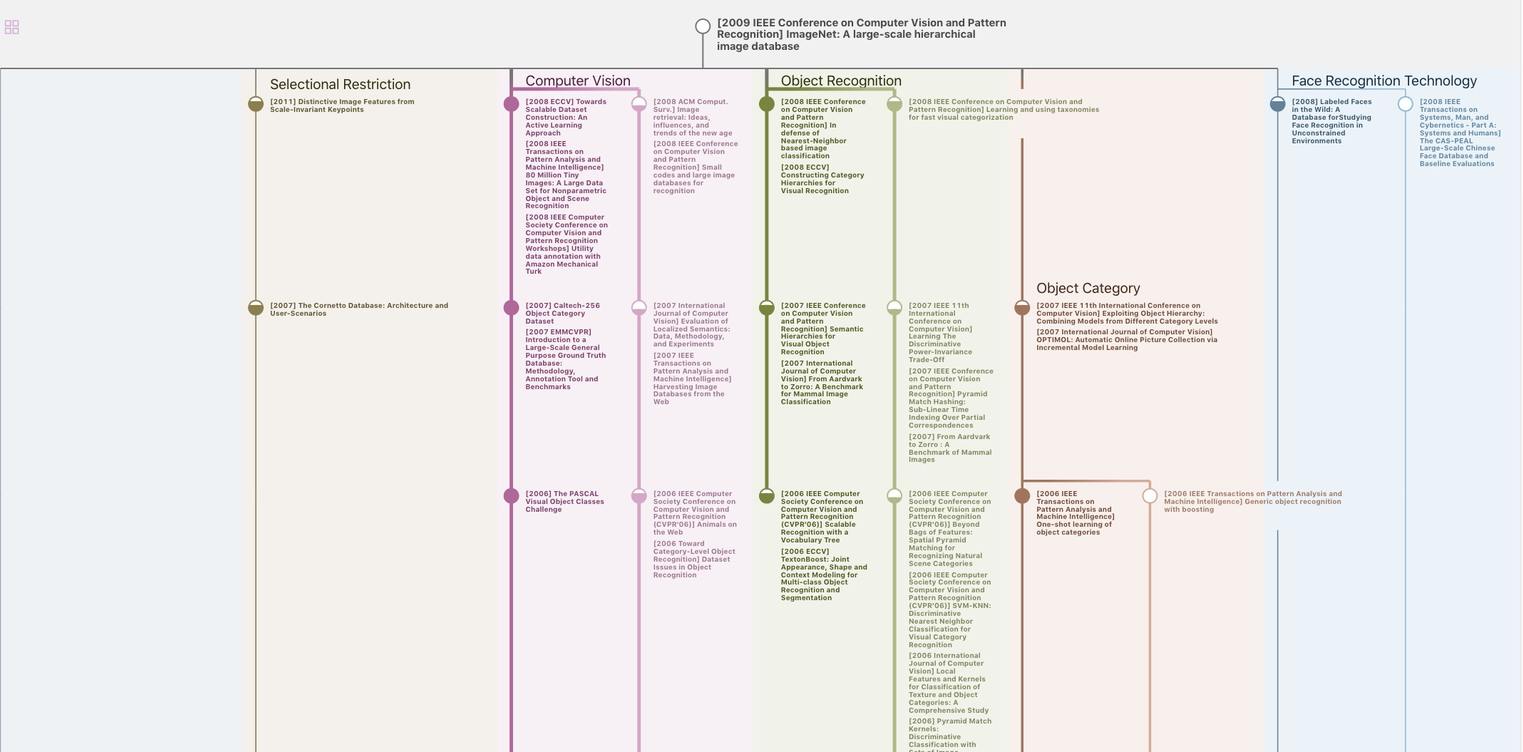
生成溯源树,研究论文发展脉络
Chat Paper
正在生成论文摘要