Forecasting adverse surgical events using self-supervised transfer learning for physiological signals
NPJ DIGITAL MEDICINE(2021)
摘要
Hundreds of millions of surgical procedures take place annually across the world, which generate a prevalent type of electronic health record (EHR) data comprising time series physiological signals. Here, we present a transferable embedding method (i.e., a method to transform time series signals into input features for predictive machine learning models) named PHASE (PHysiologicAl Signal Embeddings) that enables us to more accurately forecast adverse surgical outcomes based on physiological signals. We evaluate PHASE on minute-by-minute EHR data of more than 50,000 surgeries from two operating room (OR) datasets and patient stays in an intensive care unit (ICU) dataset. PHASE outperforms other state-of-the-art approaches, such as long-short term memory networks trained on raw data and gradient boosted trees trained on handcrafted features, in predicting six distinct outcomes: hypoxemia, hypocapnia, hypotension, hypertension, phenylephrine, and epinephrine. In a transfer learning setting where we train embedding models in one dataset then embed signals and predict adverse events in unseen data, PHASE achieves significantly higher prediction accuracy at lower computational cost compared to conventional approaches. Finally, given the importance of understanding models in clinical applications we demonstrate that PHASE is explainable and validate our predictive models using local feature attribution methods.
更多查看译文
关键词
Transfer of learning,Feature (machine learning),Machine learning,Raw data,Computer science,SIGNAL (programming language),Embedding,Intensive care unit,Hypoxemia,Artificial intelligence,Electronic health record,Surgical procedures
AI 理解论文
溯源树
样例
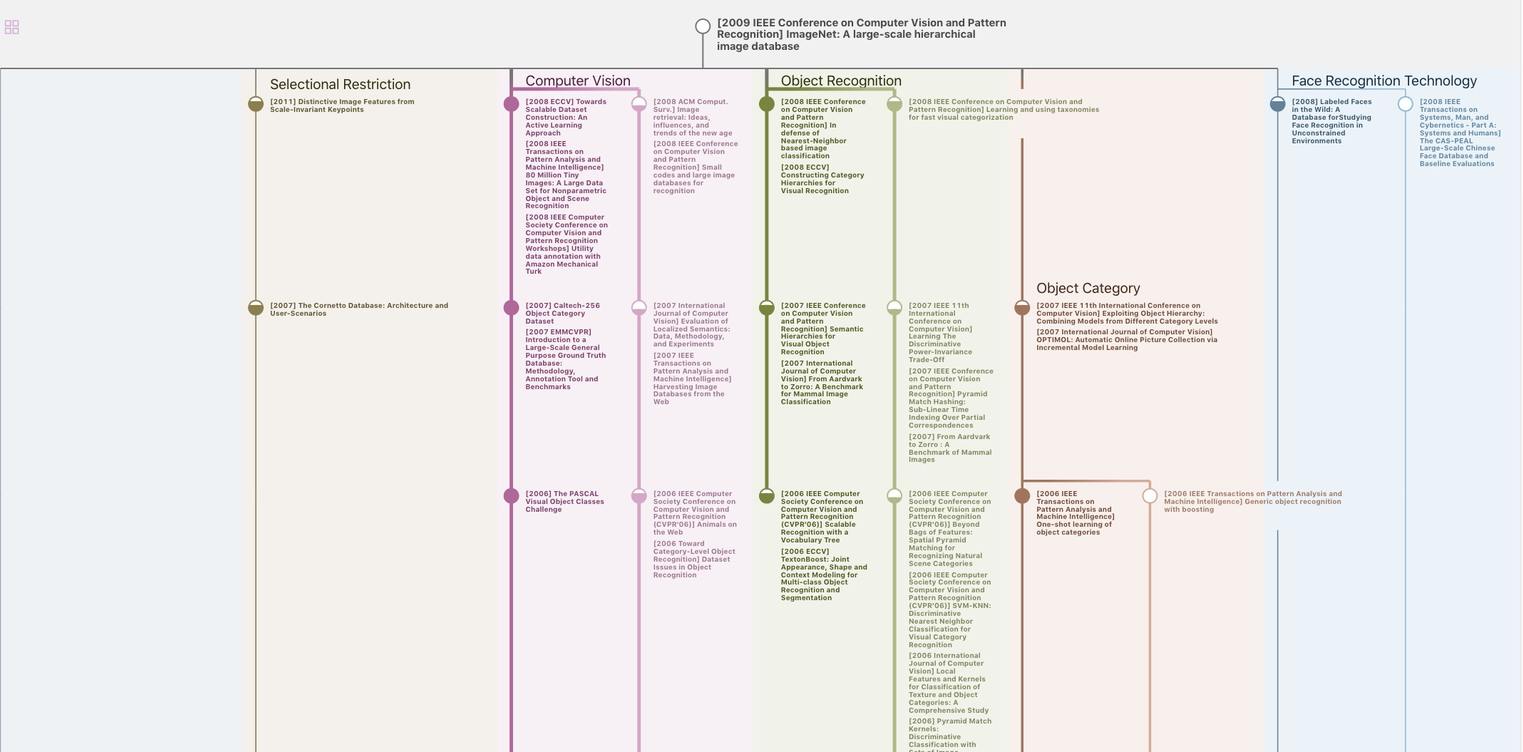
生成溯源树,研究论文发展脉络
Chat Paper
正在生成论文摘要