HieRFIT: Hierarchical Random Forest for Information Transfer
biorxiv(2020)
Abstract
The emergence of single-cell RNA sequencing (scRNA-seq) has led to an explosion in novel methods to study biological variation among individual cells, and to classify cells into functional and biologically meaningful categories. Here, we present a new cell type projection tool, HieRFIT ( Hie rarchical R andom F orest for I nformation T ransfer), based on hierarchical random forests. HieRFIT uses a priori information about cell type relationships to improve classification accuracy, taking as input a hierarchical tree structure representing the class relationships, along with the reference data. We use an ensemble approach combining multiple random forest models, organized in a hierarchical decision tree structure. We show that our hierarchical classification approach improves accuracy and reduces incorrect predictions especially for inter-dataset tasks which reflect real life applications. We use a scoring scheme that adjusts probability distributions for candidate class labels and resolves uncertainties while avoiding the assignment of cells to incorrect types by labeling cells at internal nodes of the hierarchy when necessary. Using HieRFIT, we re-analyzed publicly available scRNA-seq datasets showing its effectiveness in cell type cross-projections with inter/intra-species examples. HieRFIT is implemented as an R package and it is available at ( )
### Competing Interest Statement
The authors have declared no competing interest.
MoreTranslated text
Key words
hierarchical random forest,information transfer
AI Read Science
Must-Reading Tree
Example
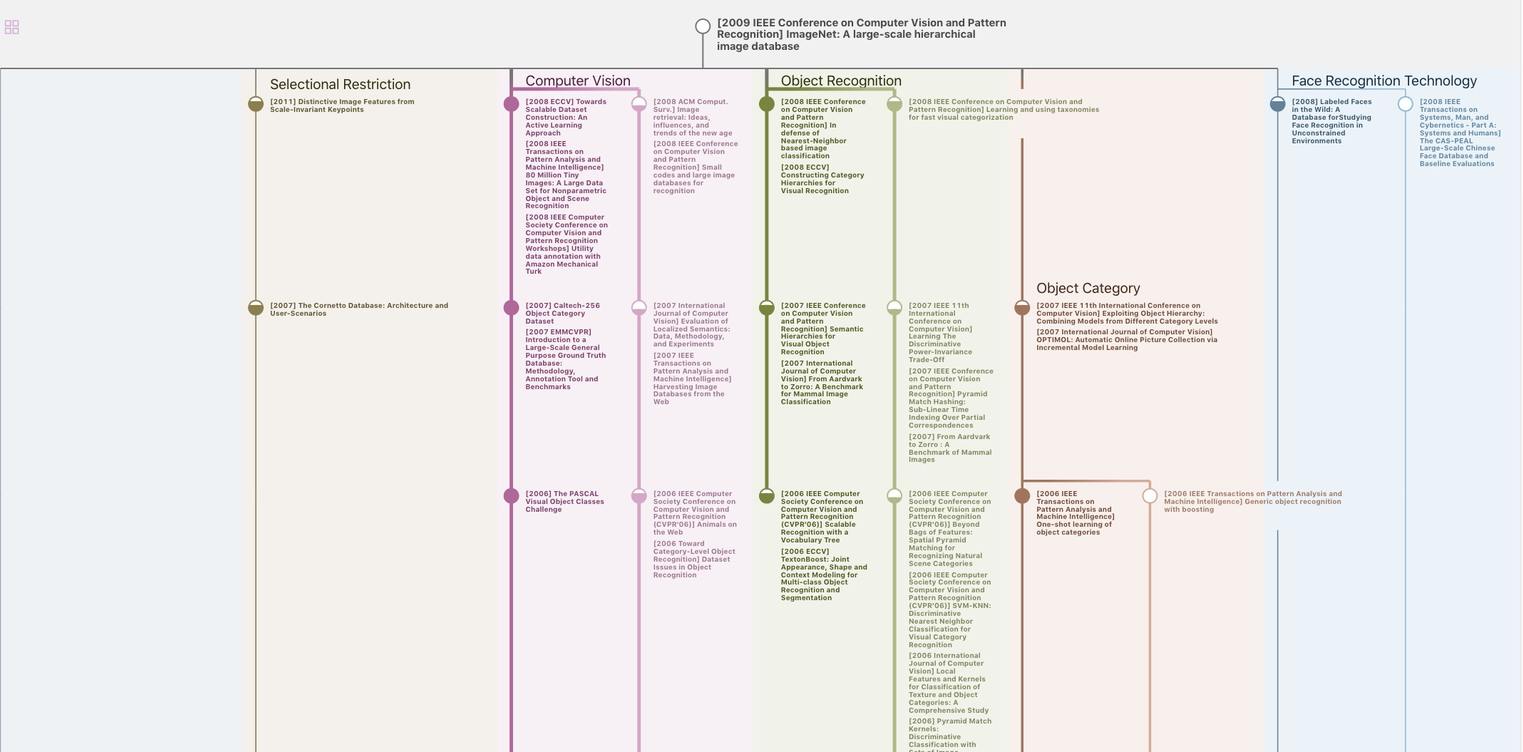
Generate MRT to find the research sequence of this paper
Chat Paper
Summary is being generated by the instructions you defined