High performance machine learning models can fully automate labeling of camera trap images for ecological analyses
bioRxiv (Cold Spring Harbor Laboratory)(2020)
摘要
1. Ecological data are increasingly collected over vast geographic areas using arrays of digital sensors. Camera trap arrays have become the ‘gold standard’ method for surveying many terrestrial mammals and birds, but these arrays often generate millions of images that are challenging to process. This causes significant latency between data collection and subsequent inference, which can impede conservation at a time of ecological crisis. Machine learning algorithms have been developed to improve camera trap data processing speeds, but these models are not considered accurate enough for fully automated labeling of images.
2. Here, we present a new approach to building and testing a high performance machine learning model for fully automated labeling of camera trap images. As a case-study, the model classifies 26 Central African forest mammal and bird species (or groups). The model was trained on a relatively small dataset ( c .300,000 images) but generalizes to fully independent data and outperforms humans in several respects (e.g. detecting ‘invisible’ animals). We show how the model’s precision and accuracy can be evaluated in an ecological modeling context by comparing species richness, activity patterns ( n = 4 species tested) and occupancy ( n = 4 species tested) derived from machine learning labels with the same estimates derived from expert labels.
3. Results show that fully automated labels can be equivalent to expert labels when calculating species richness, activity patterns ( n = 4 species tested) and estimating occupancy ( n = 3 of 4 species tested) in completely out-of-sample test data ( n = 227 camera stations, n = 23868 images). Simple thresholding (discarding uncertain labels) improved the model’s performance when calculating activity patterns and estimating occupancy, but did not improve estimates of species richness.
4. We provide the user-community with a multi-platform, multi-language user interface for running the model offline, and conclude that high performance machine learning models can fully automate labeling of camera trap data.
### Competing Interest Statement
The authors have declared no competing interest.
更多查看译文
关键词
camera trap images,machine learning,labeling
AI 理解论文
溯源树
样例
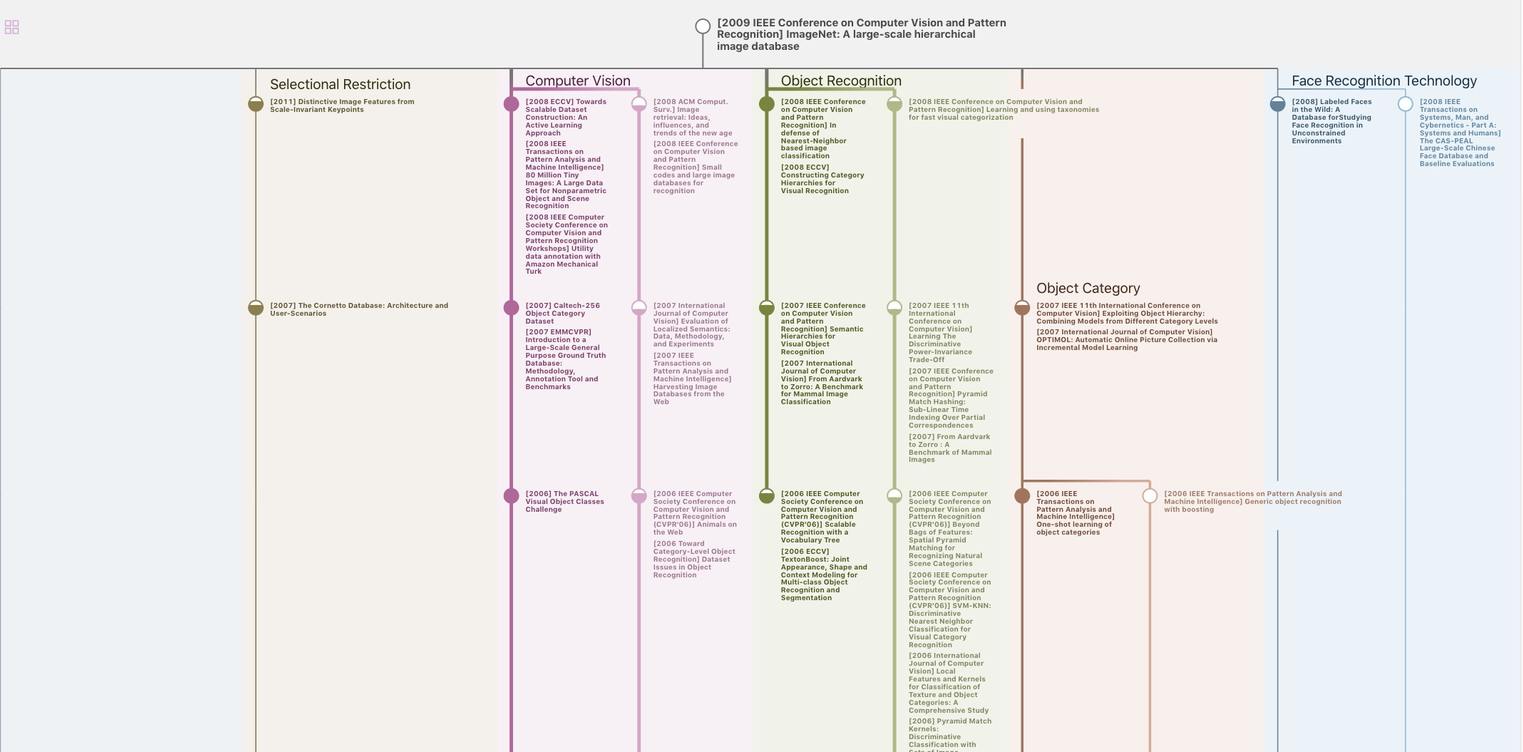
生成溯源树,研究论文发展脉络
Chat Paper
正在生成论文摘要