Mitigating Intensity Bias in Shadow Detection via Feature Decomposition and Reweighting.
ICCV(2021)
摘要
While CNNs achieved remarkable progress in shadow detection, they tend to make mistakes in dark non-shadow regions and relatively bright shadow regions. They are also susceptible to brightness change. These two phenomenons reveal that deep shadow detectors heavily depend on the intensity cue, which we refer to as intensity bias. In this paper, we propose a novel feature decomposition and reweighting scheme to mitigate this intensity bias, in which multi-level integrated features are decomposed into intensity-variant and intensity-invariant components through self-supervision. By reweighting these two types of features, our method can reallocate the attention to the corresponding latent semantics and achieves balanced exploitation of them. Extensive experiments on three popular datasets show that the proposed method outperforms state-of-the-art shadow detectors.
更多查看译文
关键词
Low-level and physics-based vision
AI 理解论文
溯源树
样例
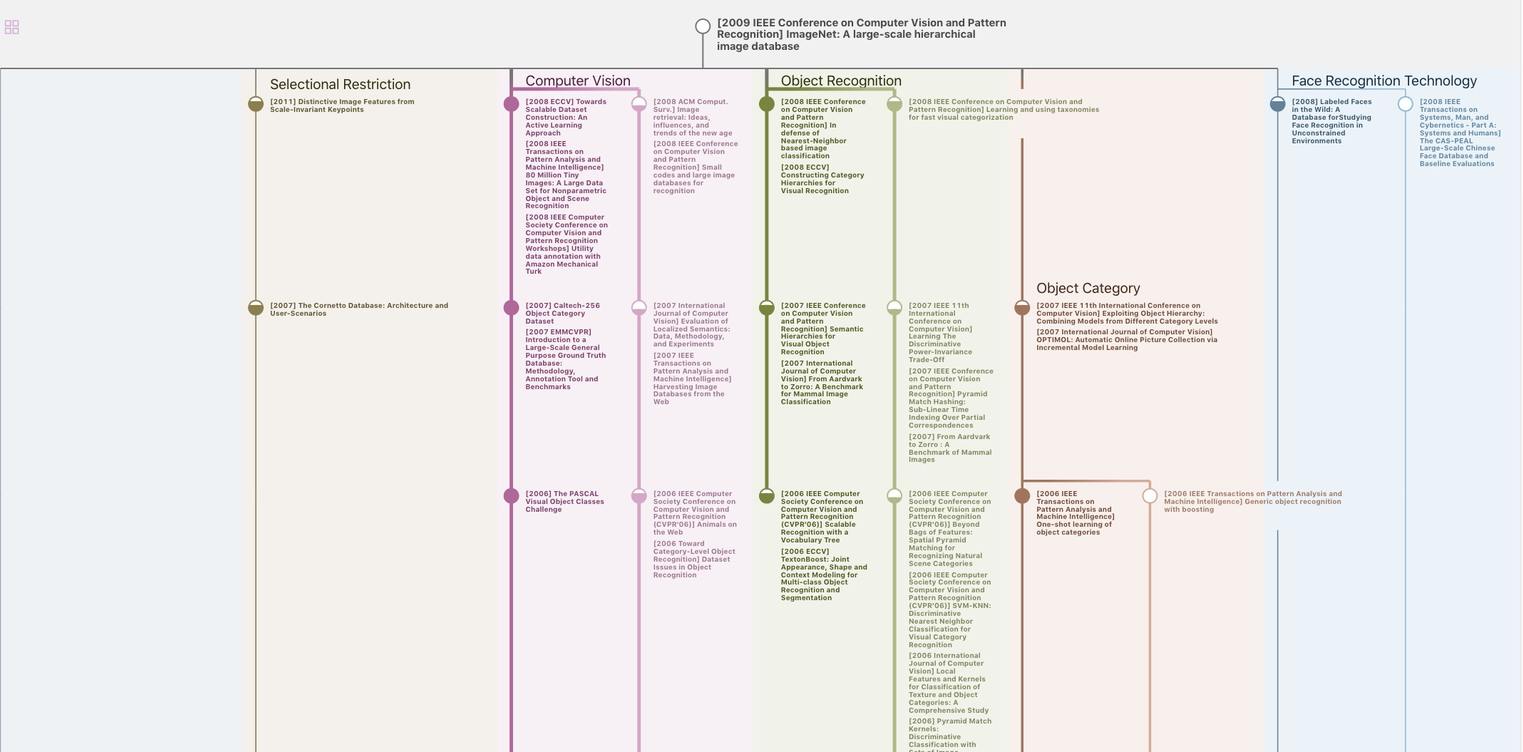
生成溯源树,研究论文发展脉络
Chat Paper
正在生成论文摘要