Learning Attribute-driven Disentangled Representations for Interactive Fashion Retrieval.
ICCV(2021)
摘要
Interactive retrieval for online fashion shopping provides the ability of changing image retrieval results according to the user feedback. One common problem in interactive retrieval is that a specific user interaction (e.g., changing the color of a T-shirt) causes other aspects to change inadvertently (e.g., the results have a sleeve type different from that of the query). This is a consequence of existing methods learning visual representations that are entangled in the embedding space, which limits the controllability of the retrieved results. We propose to leverage on the semantics of visual attributes to train convolutional networks that learn attribute-specific subspaces for each attribute type to obtain disentangled representations. Operations, such as swapping out a particular attribute value for another, impact the attribute at hand and leave others untouched. We show that our model can be tailored to deal with different retrieval tasks while maintaining its disentanglement property. We obtained state-of-the-art performance on three interactive fashion retrieval tasks: attribute manipulation retrieval, conditional similarity retrieval, and outfit complementary item retrieval. We will make code and models publicly available.
更多查看译文
关键词
Image and video retrieval,Representation learning,Vision applications and systems
AI 理解论文
溯源树
样例
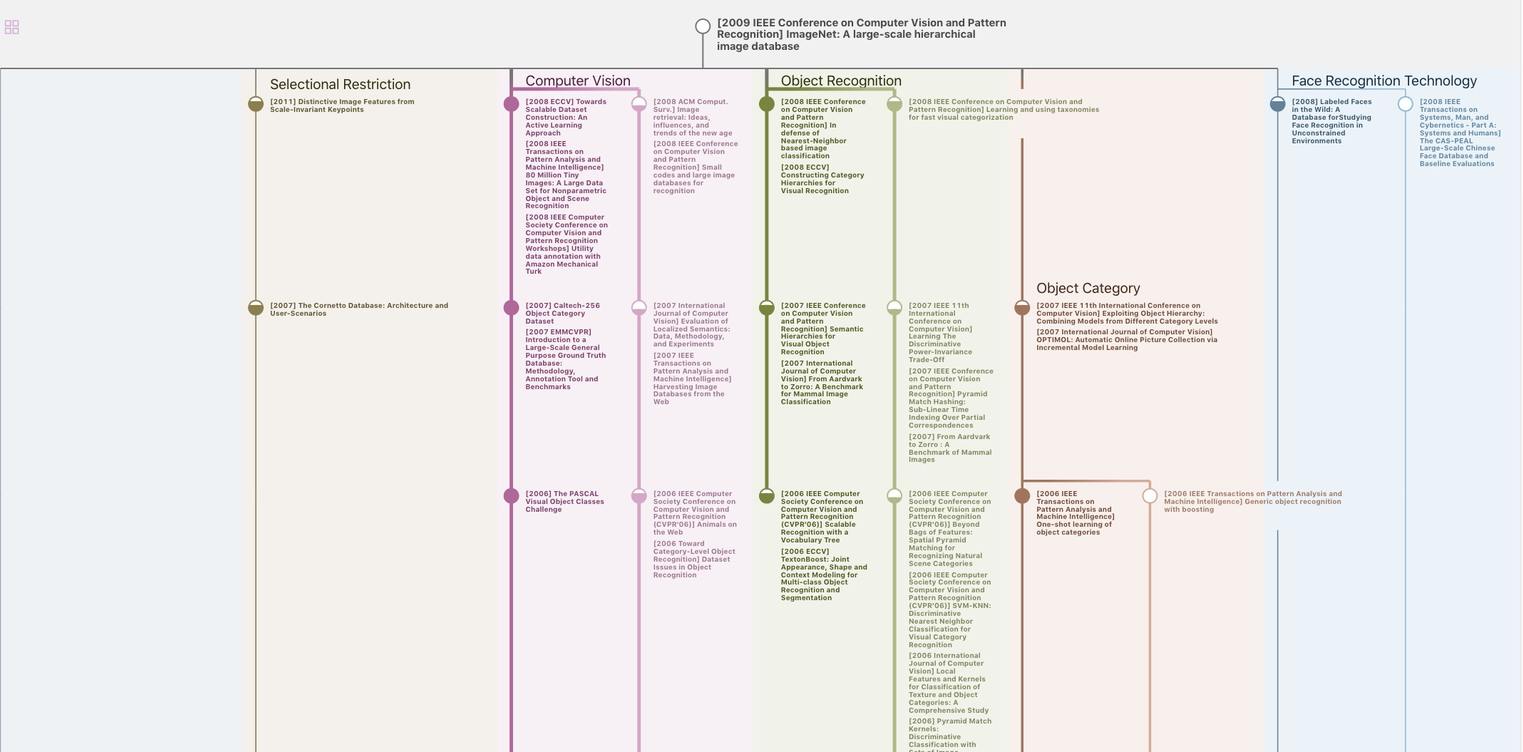
生成溯源树,研究论文发展脉络
Chat Paper
正在生成论文摘要