Deep-learning-based bright-field image generation from a single hologram using an unpaired dataset
OPTICS LETTERS(2021)
Abstract
We adopted an unpaired neural network training technique, namely CycleGAN, to generate bright-field microscope-like images from hologram reconstructions. The motivation for unpaired training in microscope applications is that the construction of paired/parallel datasets is cumbersome or sometimes not even feasible, for example, lensless or flow-through holographic measuring setups. Our results show that the proposed method is applicable in these cases and provides comparable results to the paired training. Furthermore, it has some favorable properties even though its metric scores are lower. The CycleGAN training results in sharper and-from this point of view-more realistic object reconstructions compared to the baseline paired setting. Finally, we show that a lower metric score of the unpaired training does not necessarily imply a worse image generation but a correct object synthesis, yet with a different focal representation. (C) 2021 Optical Society of America
MoreTranslated text
Key words
single hologram,deep-learning-based,bright-field
AI Read Science
Must-Reading Tree
Example
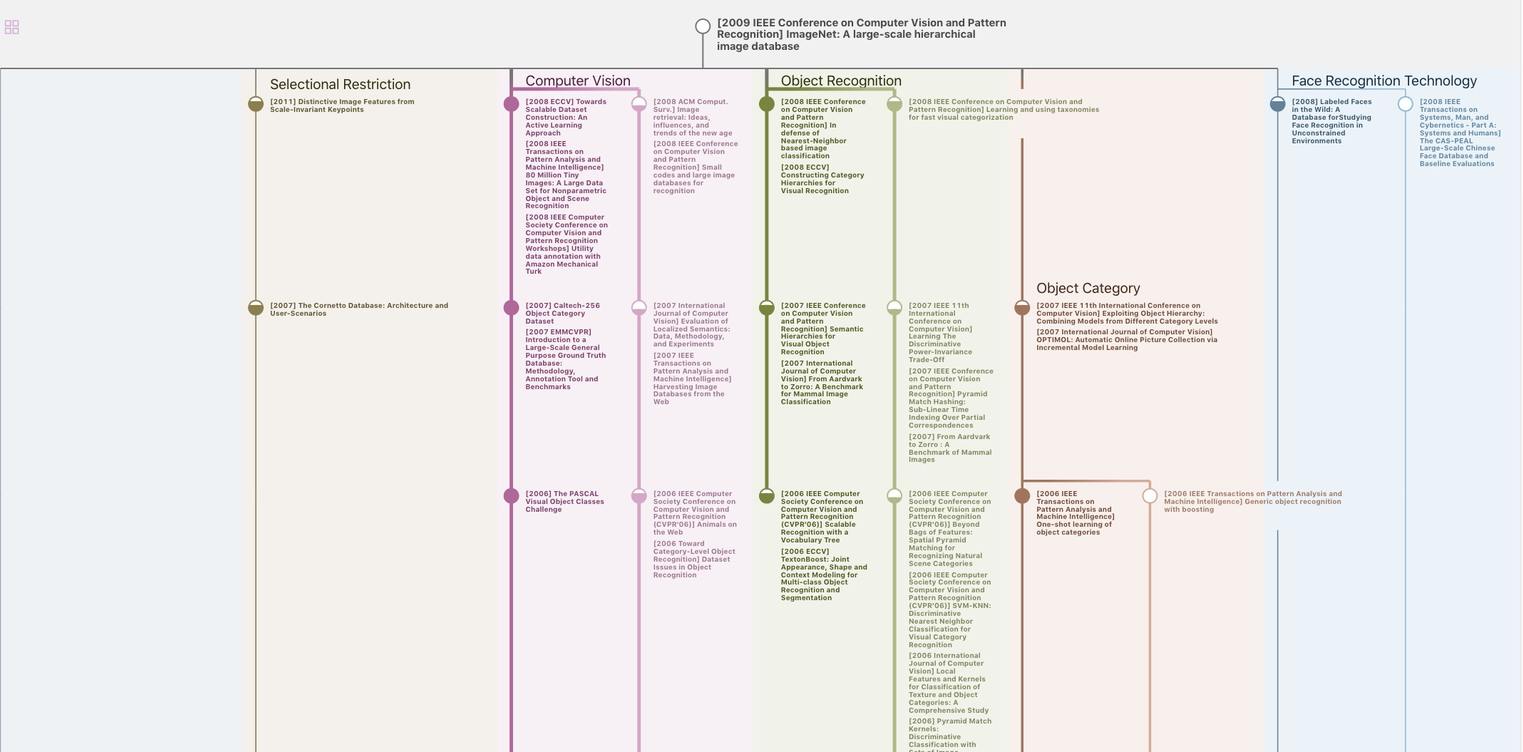
Generate MRT to find the research sequence of this paper
Chat Paper
Summary is being generated by the instructions you defined