A Label Similarity Probability Filter for Hyperspectral Image Postclassification
IEEE JOURNAL OF SELECTED TOPICS IN APPLIED EARTH OBSERVATIONS AND REMOTE SENSING(2021)
摘要
This article presents a label similarity probability filter (LSPF) to make hyperspectral image postclassification. The LSPF is inspired by the first law of geography and proposes a class label probability function to quantify the probability of both centered and its neighboring pixels belonging to the same class. It first classifies the hyperspectral data using the regular support vector machine classifier. Then, it binarizes the posterior classification result to obtain the binary label maps of each class. After that, it traverses all spatial windows centered by each pixel and calculates the cumulative probability of all pixels in each class. Finally, the cumulative probabilities are used to make reclassification to obtain the refined classification map. The experiments on Indian Pines, Pavia University, and ZY1-02D Yellow River Estuary data show that LSPF greatly improves the classification accuracy of spectral signatures and outperforms other state-of-the-art spectral-spatial methods.
更多查看译文
关键词
Hyperspectral imaging,Support vector machines,Probability,Feature extraction,Information filters,Geography,Degradation,Hyperspectral,label similarity probability filter (LSPF),postclassification,spectral-spatial methods
AI 理解论文
溯源树
样例
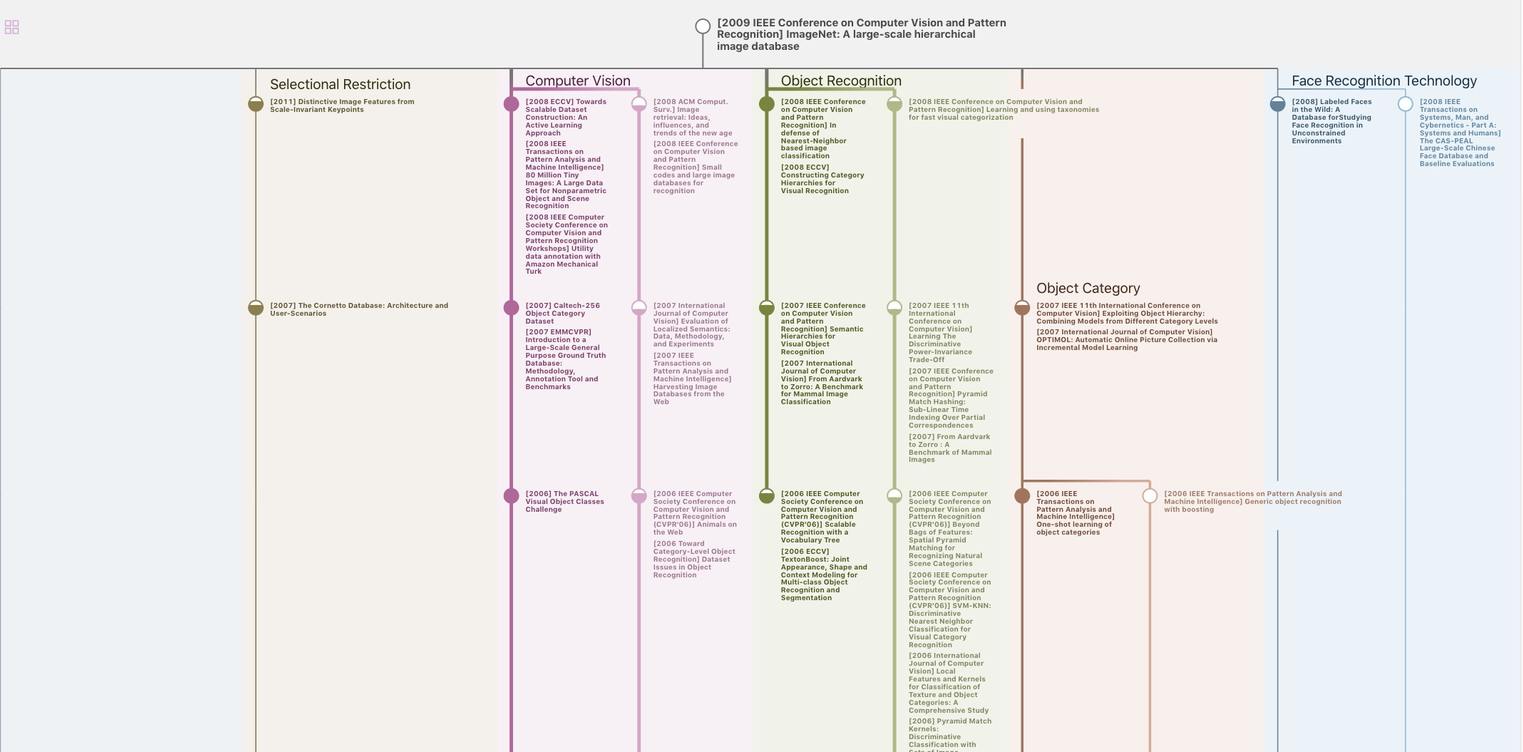
生成溯源树,研究论文发展脉络
Chat Paper
正在生成论文摘要