Hyperspectral Remote Sensing Image Classification Using Deep Convolutional Capsule Network
IEEE JOURNAL OF SELECTED TOPICS IN APPLIED EARTH OBSERVATIONS AND REMOTE SENSING(2021)
Abstract
Deep learning models have shown excellent performance in the hyperspectral remote sensing image (HSI) classification. In particular, convolutional neural networks (CNNs) have received widespread attention because of their powerful feature-extraction ability. Recently, a capsule network (CapsNet) was introduced to boost the performance of CNNs, marking a remarkable progress in the field of HSI classification. In this article, we propose a novel deep convolutional capsule neural network (DC-CapsNet) based on spectral-spatial features to improve the performance of CapsNet in the HSI classification while significantly reducing the computation cost of the model. Specifically, a convolutional capsule layer based on the extension of dynamic routing using 3-D convolution is used to reduce the number of parameters and enhance the robustness of the learned spectral-spatial features. Furthermore, a lighter and stronger decoder network composed of deconvolutional layers as a better regularization term and capable of acquiring more spatial relationships is used to further improve the HSI classification accuracy with low computation cost. In this study, we tested the performance of the proposed model on four widely used HSI datasets: the Kennedy Space Center, Indian Pines, Pavia University, and Salinas datasets. We found that the DC-CapsNet achieved high classification accuracy with limited training samples and effectively reduced the computation cost.
MoreTranslated text
Key words
Three-dimensional displays,Feature extraction,Convolution,Computational modeling,Routing,Training,Solid modeling,Capsule neural network,convolutional neural network (CNN),hyperspectral image classification
AI Read Science
Must-Reading Tree
Example
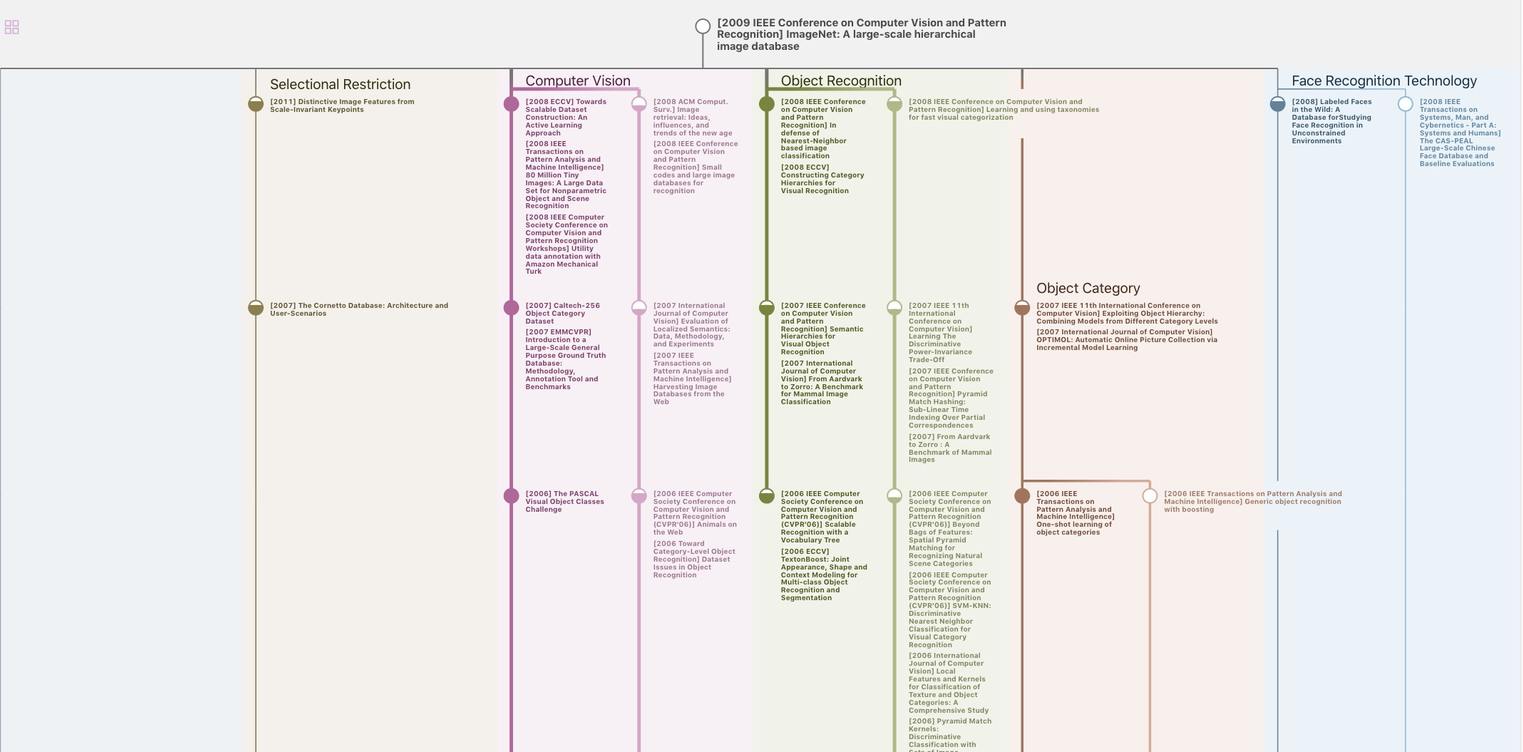
Generate MRT to find the research sequence of this paper
Chat Paper
Summary is being generated by the instructions you defined