Anomaly Detection for Hyperspectral Images Based on Improved Low-Rank and Sparse Representation and Joint Gaussian Mixture Distribution
IEEE JOURNAL OF SELECTED TOPICS IN APPLIED EARTH OBSERVATIONS AND REMOTE SENSING(2021)
摘要
The background dictionary used in the hyperspectral images anomaly detection based on low-rank and sparse representation (LRASR) contains both target information and background information which will result in low detection accuracy. In response to this problem, this article proposes an improved hyperspectral anomaly detection algorithm that is based on low-rank and sparse representation and joint Gaussian mixture distribution (MOG-LRASR). Modeling the sparse components as a mixture of Gaussian (MOG) distribution in the traditional low-rank and sparse decomposition model can get a purer low-rank background component. Then using the low-rank background component as the input of the dictionary learning to get the sparse matrix to be detected. Since the distribution in the anomalous part is usually sparse and complex, Manhattan distance is used to evaluate anomalous pixels in this article. Using Wilcoxon rank-sum test, the experimental results show that the algorithm proposed in this article has the highest score, which proves the MOG-LRASR has higher detection stability than other algorithms. Also, it has achieved better detection results on other data sets indicated by the experiments.
更多查看译文
关键词
Sparse matrices,Dictionaries,Anomaly detection,Matrix decomposition,Hyperspectral imaging,Machine learning,Sun,Anomaly detection,dictionary construction,hyperspectral image (HSI),low-rank and sparse representation (LRASR),mixture of Gaussian (MOG)
AI 理解论文
溯源树
样例
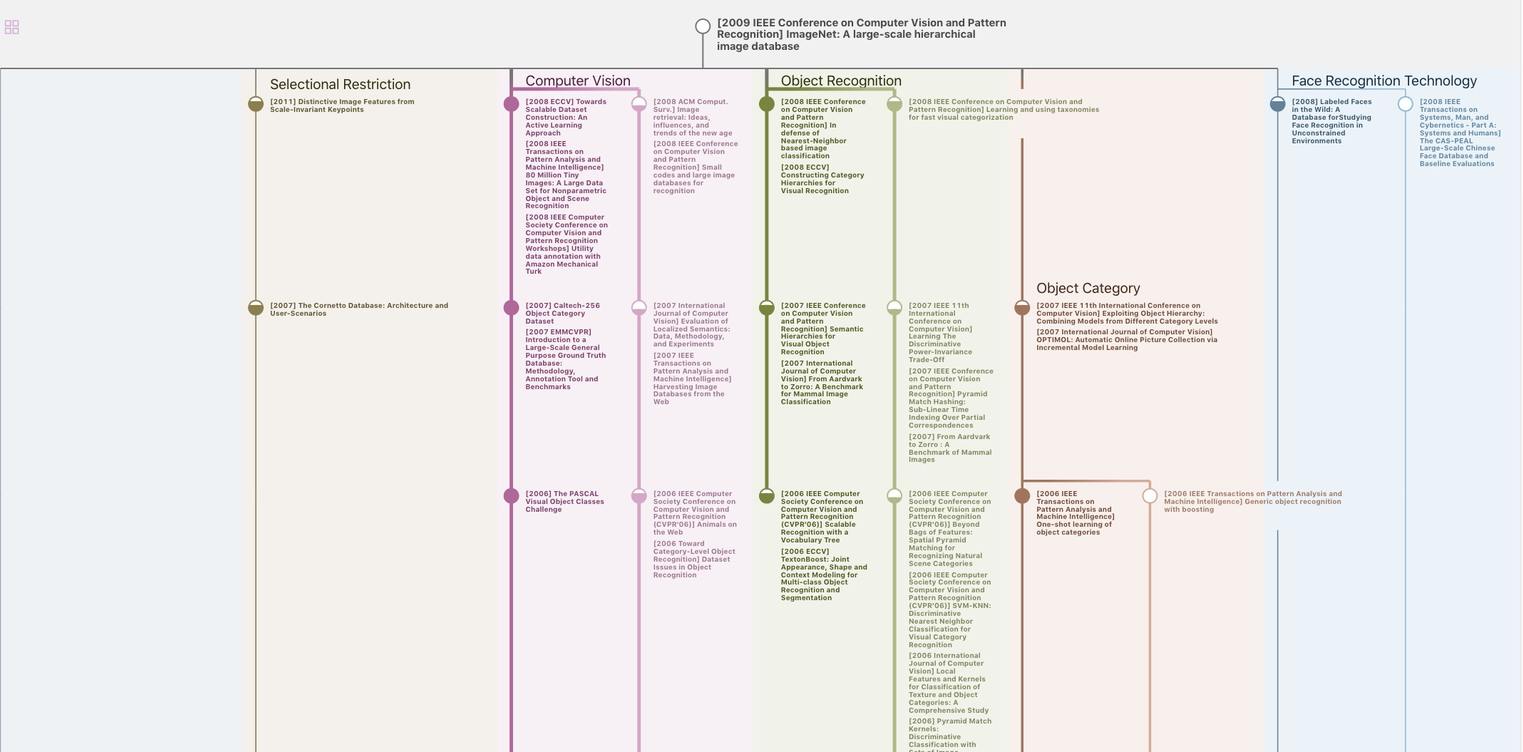
生成溯源树,研究论文发展脉络
Chat Paper
正在生成论文摘要