Towards Robust Autonomous Coverage Navigation for Carlike Robots
IEEE ROBOTICS AND AUTOMATION LETTERS(2021)
Abstract
Thanks to their high carrying capacity and strong maneuverability, carlike robots which move with non-holonomic constraints, are frequently utilized in numerous coverage operation fields. In such fields, the robots need to complete the coverage task via autonomous path planning and tracking, which is referred to as a "Coverage Navigation for Carlike Robots" (CNCR) problem. When it comes to CNCR, two folds of challenges are put forward as follows. On one hand, the local path planner should not only consider the non-holonomic constraints of carlike robots but also should meet the high coverage rate required by the coverage task. On the other hand, during CNCR, there must be many U-turns in narrow areas. As a result, Reeds-Shepp-type paths will be planned inevitably. Tracking this sort of multi-segment paths stably is also an urgent problem to be solved in CNCR. To this end, this letter introduces a reference line constraint and a heuristic initialization strategy along with a cubic parametric path smoother to the time elastic band planner and designs a multi-segment-supported path tracker. Experimental results show that the proposed path planner largely reduces the average deviation off the reference line and obtains a high success rate even in complex environments. Besides, the designed path tracker achieves stable and high-precision tracking for multi-segment paths. Real-world experiments reveal that the proposed path planner and tracker are fully competent for CNCR, rendering our sweeping robot to attain an actual average coverage rate of 92.21%. To make our results fully reproducible, relevant codes and data are made available online at https://github.com/csLinZhang/cncr.git.
MoreTranslated text
Key words
Robots, Tracking, Planning, Optimization, Navigation, Task analysis, Location awareness, Autonomous vehicle navigation, motion control, motion and path planning
AI Read Science
Must-Reading Tree
Example
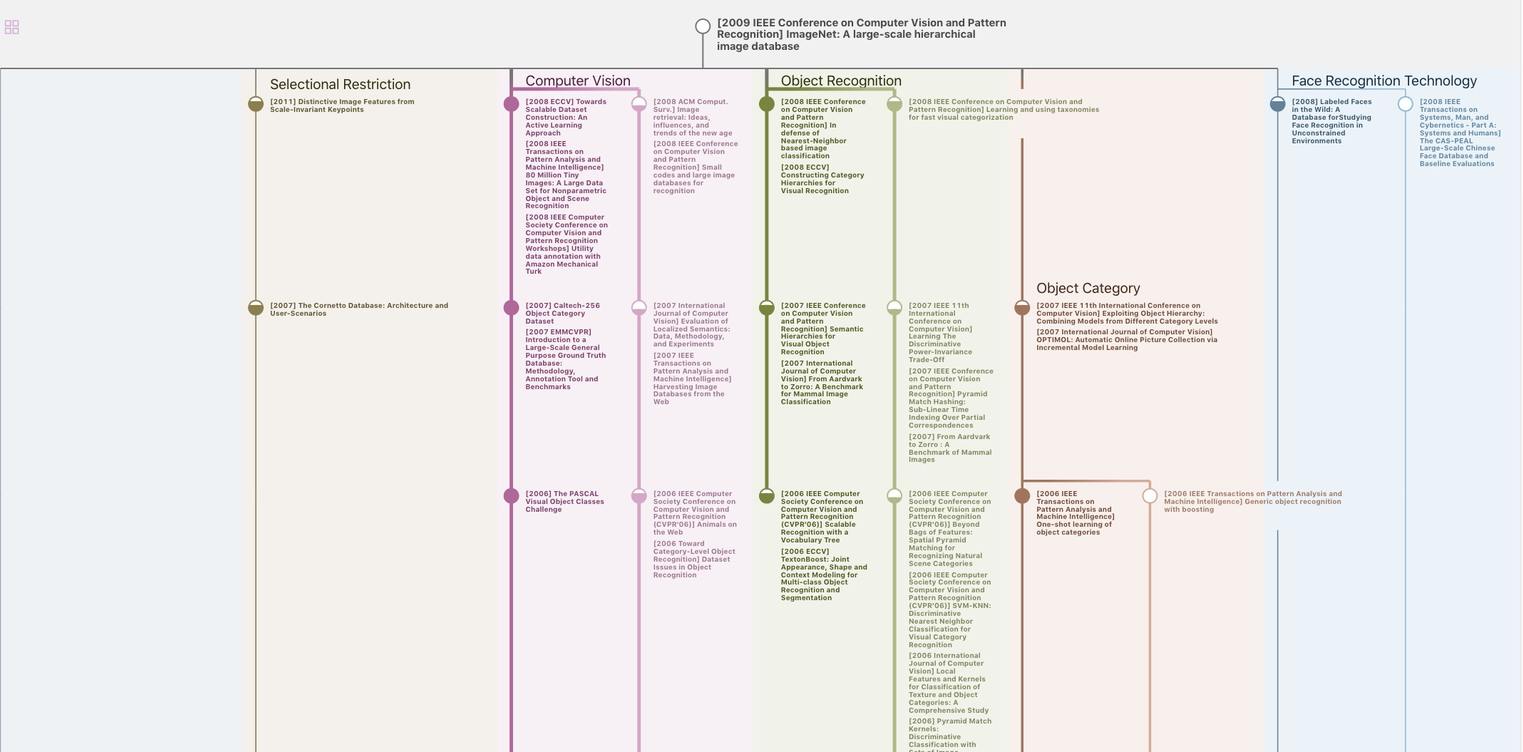
Generate MRT to find the research sequence of this paper
Chat Paper
Summary is being generated by the instructions you defined