A Sequence-to-Sequence Model With Attention and Monotonicity Loss for Tool Wear Monitoring and Prediction
IEEE TRANSACTIONS ON INSTRUMENTATION AND MEASUREMENT(2021)
摘要
Recently, deep learning has been successfully applied in tool wear monitoring systems. However, since the tool wear accumulates in the cutting process, the state of the cutting tool shows a degradation trend, which has not been fully exploited by the current deep learning models. In this article, an end-to-end deep learning model, named sequence-to-sequence model with attention and monotonicity loss (SMAML) is proposed to simultaneously monitor and predict the tool wear. In the proposed SMAML, the encoder is utilized to extract features from signals collected by different sensors using the group convolution, while the decoder is designed to produce sequential outputs, including the results of tool wear monitoring and multistep-ahead prediction. Besides, a monotonicity loss function is proposed to capture the degeneration characters in these sequential outputs. The experiments are conducted on real-world datasets, which were collected from a high-speed CNC machine. The experimental results demonstrate the effectiveness of the proposed model which outperforms other conventional machine learning and deep learning models.
更多查看译文
关键词
Attention mechanism,deep learning,monotonicity loss,sequence-to-sequence learning,tool wear monitoring and prediction
AI 理解论文
溯源树
样例
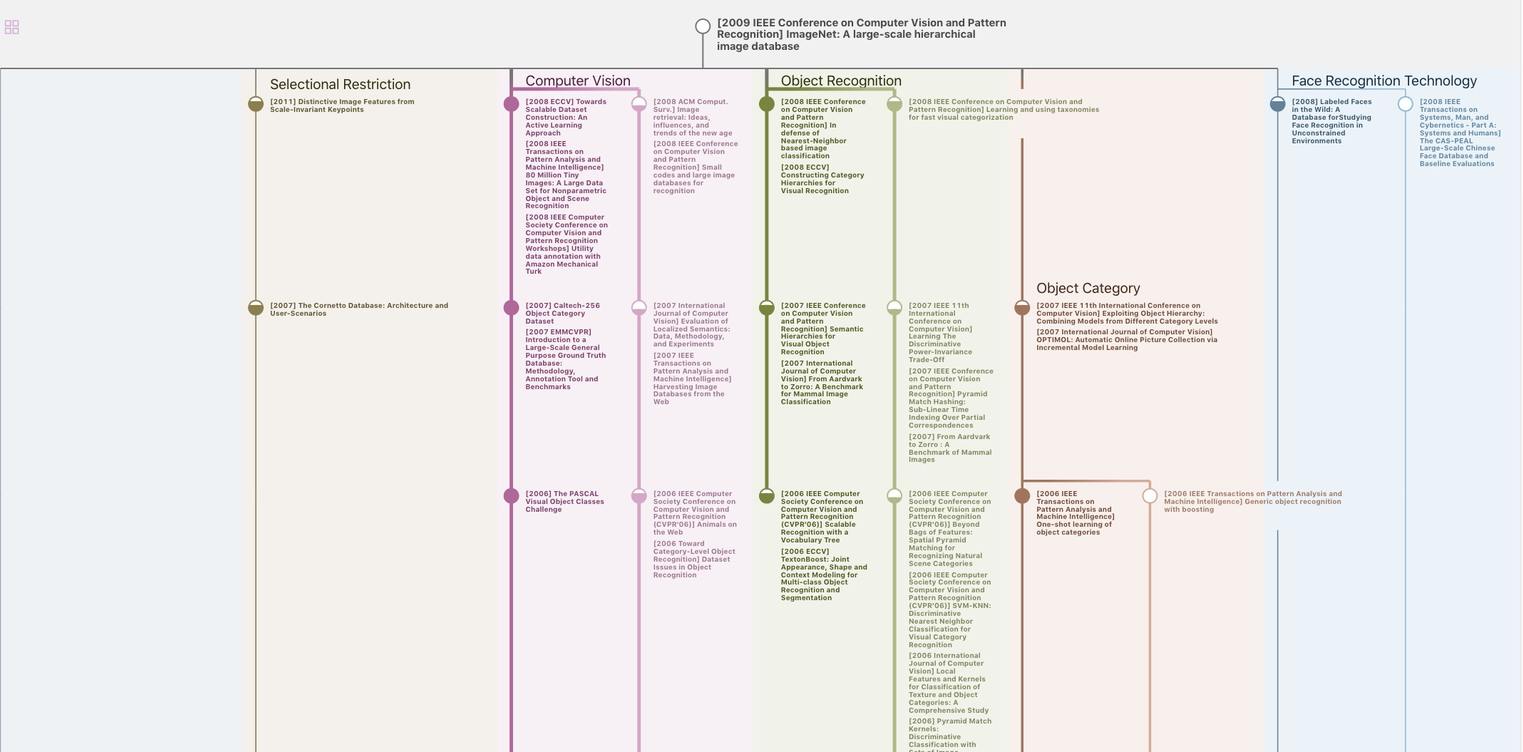
生成溯源树,研究论文发展脉络
Chat Paper
正在生成论文摘要