ACR-Net: Attention Integrated and Cross-Spatial Feature Fused Rotation Network for Tubular Solder Joint Detection
IEEE TRANSACTIONS ON INSTRUMENTATION AND MEASUREMENT(2021)
摘要
Tubular solder joint detection is an important and challenging issue in the industry, due to the illegible objects, rarely collected datasets and requiring high-precision and real-time performance for positioning and angle estimation. In this article, we propose an Attention integrated and Cross-spatial feature fused Rotation Network (ACR-Net) for tubular solder joint detection, which consists of a new feature extraction network named ECA-CSPDarknet44, a cross-spatial feature fusion network (CFFN), and a bin-based rotation detection network (BRDN). The proposed network can efficiently detect oriented tubular solder joints with high-precision and real-time performance. ECA-CSPDarknet44 with attention mechanism was presented, which can adaptively guide the network to learn important features of tubular solder joints, significantly improving the ability of feature extraction. By integrating multiscale global features and multiscale local region features, CFFN can enhance the network's ability to express the characteristics of tubular solder joints. Meanwhile, BRDN is proposed for oriented bounding box regression through a decoupling approach, which regresses target parameters efficiently and accurately with little increasing of model complexity. Finally, we establish a tubular solder joint dataset and conduct sufficient experiments to verify the effectiveness of our method. Our proposed ACR-Net achieves 98.8% mAP with 31.3 frames per second (FPSs) on the dataset, meeting the high-precision and real-time requirements of industrial systems.
更多查看译文
关键词
Deep learning,defect detection,object detection,tubular solder joint detection
AI 理解论文
溯源树
样例
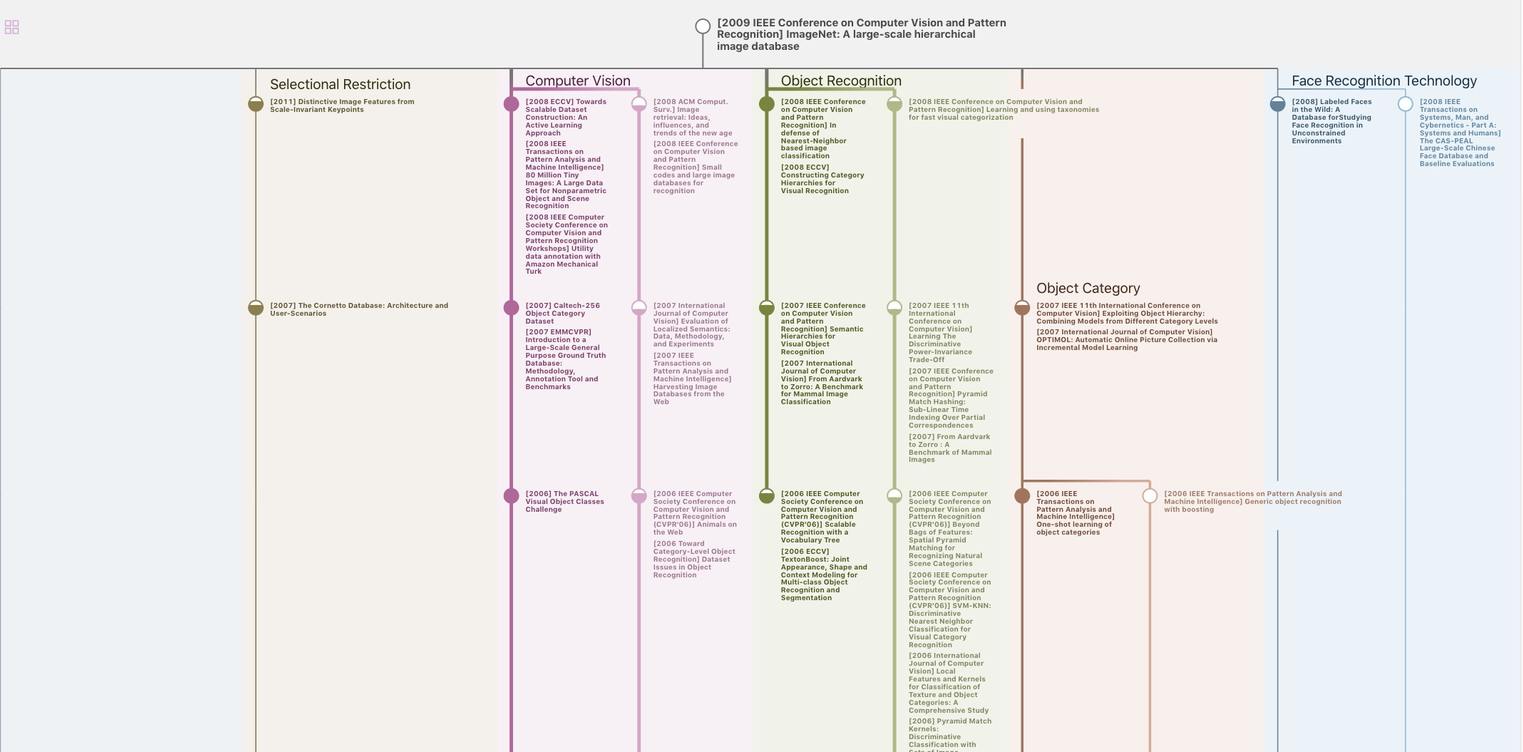
生成溯源树,研究论文发展脉络
Chat Paper
正在生成论文摘要