Feature Fused Human Activity Recognition Network (FFHAR-Net).
HCI (38)(2021)
Abstract
With the advances in smart home technology and Internet of Things (IoT), there has been keen research interest in human activity recognition to allow service systems to understand human intentions. Recognizing human objectives by these systems without user intervention, results in better service, which is crucial to improve the user experience. Existing research approaches have focused primarily on probabilistic methods like Bayesian networks (for instance, the CRAFFT algorithm). Though quite versatile, these probabilistic models may be unable to successfully capture the possibly complex relationships between the input variables. To the best of our knowledge, a statistical study of features in a human activity recognition task, their relationships, etc., has not yet been attempted. To this end, we study the domain of human activity recognition to improve the state-of-the-art and present a novel neural network architecture for the task. It employs early fusion on different types of minimalistic features such as time and location to make extremely accurate predictions with a maximum micro F1-score of 0.98 on the Aruba CASAS dataset. We also accompany the model with a comprehensive study of the features. Using feature selection techniques like Leave-One-Out, we rank the features according to the information they add to deep learning models and make further inferences using the ranking obtained. Our empirical results show that the feature Previous Activity Performed is the most useful of all, surprisingly even more than time (the basis of activity scheduling in most societies). We use three Activities of Daily Living (ADL) datasets in different settings to empirically demonstrate the utility of our architecture. We share our findings along with the models and the source code.
MoreTranslated text
Key words
Human activity recognition,Activities of Daily Living,Deep learning
AI Read Science
Must-Reading Tree
Example
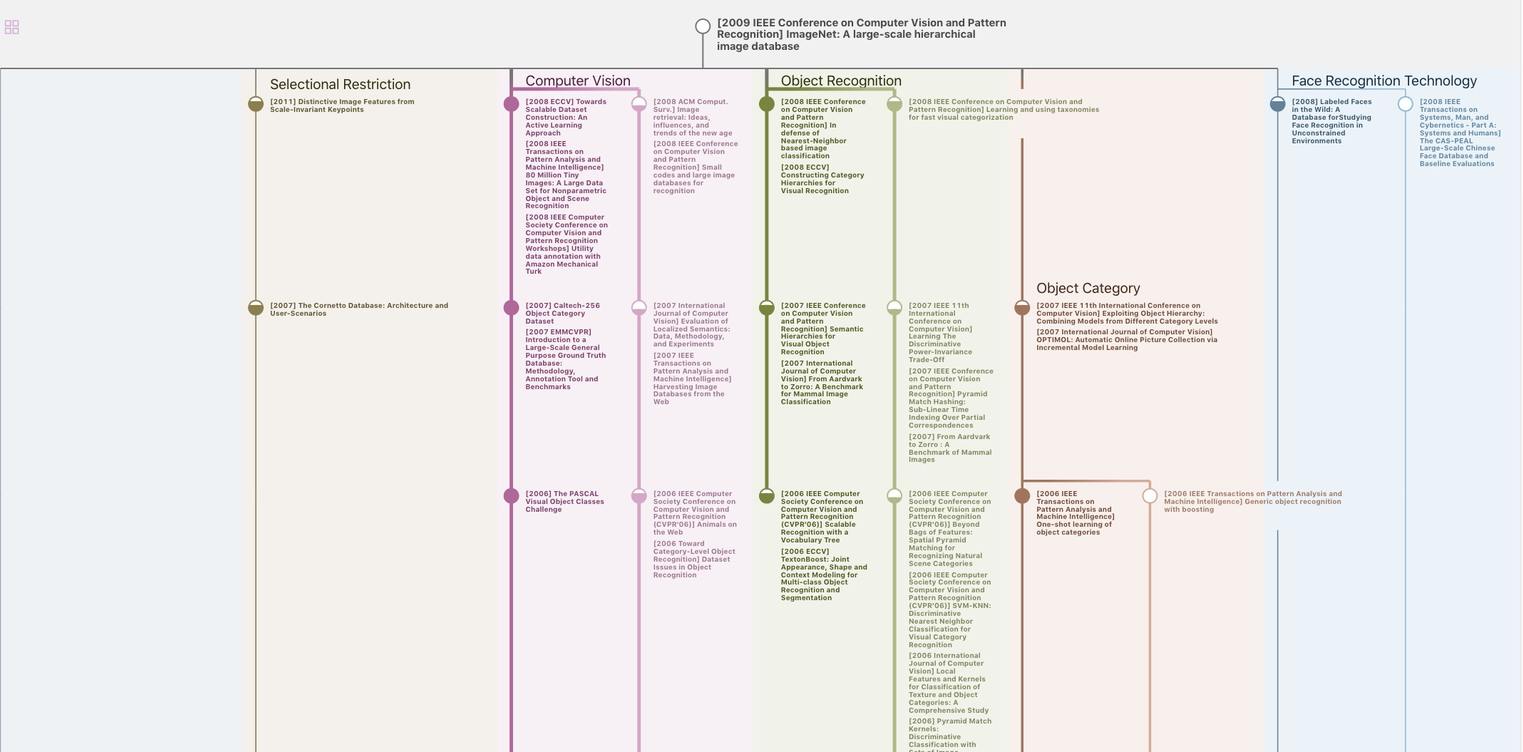
Generate MRT to find the research sequence of this paper
Chat Paper
Summary is being generated by the instructions you defined