Vicinity-Based Abstraction: VA-DGCNN Architecture for Noisy 3D Indoor Object Classification.
ICCS (3)(2021)
摘要
One of the outstanding benchmark architectures for point cloud processing with graph-based structures is Dynamic Graph Convolutional Neural Network (DGCNN). Though it works well for classification of nearly perfectly described digital models, it leaves much to be desired for real-life cases burdened with noise and 3D scanning shadows. Therefore we propose a novel, feature-preserving vicinity abstraction (VA) layer for the EdgeConv module. This allowed for enriching the global feature vector with the local context provided by the k -NN graph. Rather than processing a point together with its neighbours at once, local information is aggregated before further processing, unlike in the original DGCNN. Such an approach enabled a model to learn accumulated information instead of max-pooling features from local context at the end of each EdgeConv module. Thanks to this strategy mean- and overall classification accuracy increased by 9.4pp and 4.4pp, respectively. Furthermore, thanks to processing aggregated information rather than the entire vicinity, the new VA-DGCNN model converges significantly faster than the original DGCNN.
更多查看译文
关键词
3D object classification, Point clouds, DGCNN
AI 理解论文
溯源树
样例
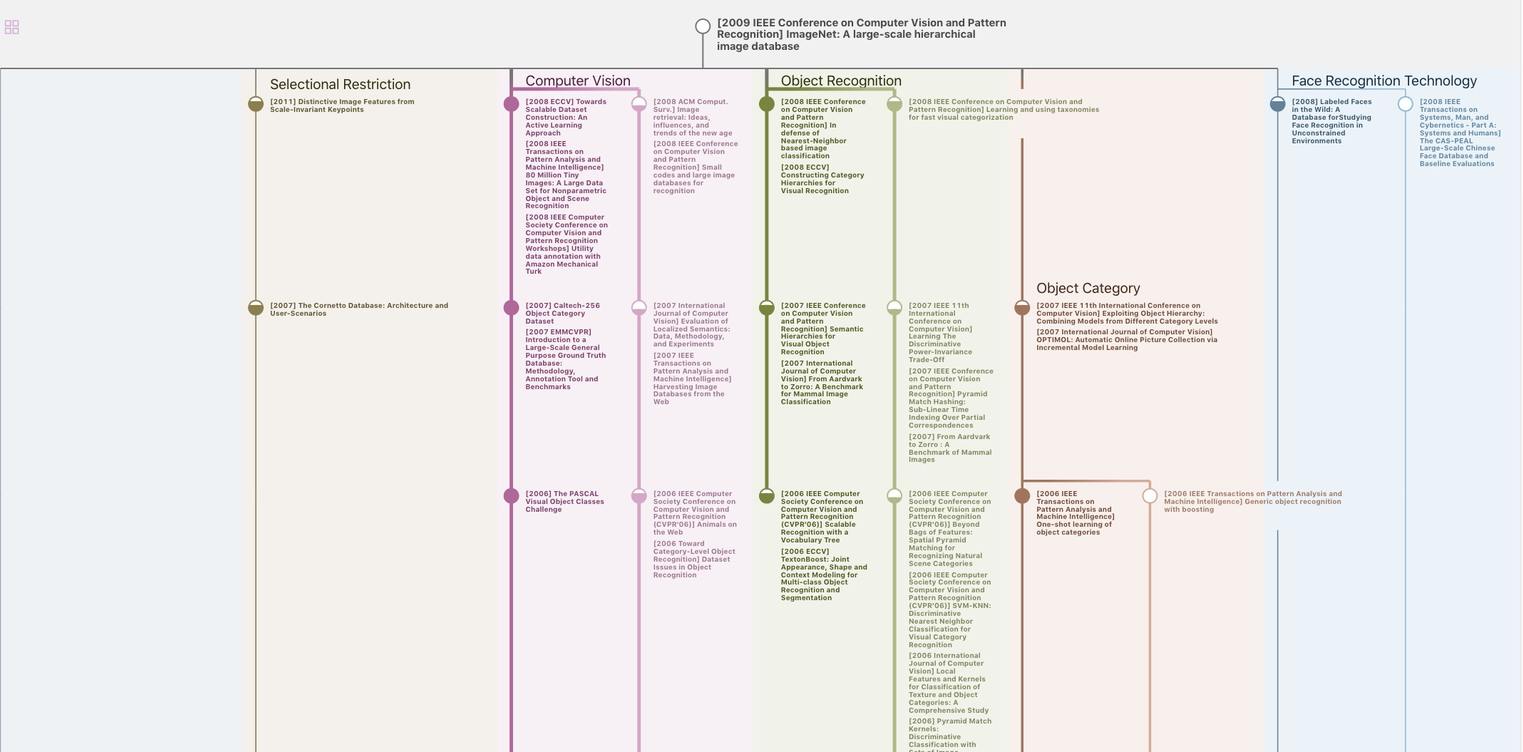
生成溯源树,研究论文发展脉络
Chat Paper
正在生成论文摘要