Optimizing Model Training in Interactive Learning Scenarios.
WorldCIST (1)(2021)
摘要
In the last years, developments in data collection, storing, processing and analysis technologies resulted in an unprecedented use of data by organizations. The volume and variety of data, combined with the velocity at which decisions must now be taken and the dynamism of business environments, pose new challenges to Machine Learning. Namely, algorithms must now deal with streaming data, concept drift, distributed datasets, among others. One common task nowadays is to update or re-train models when data changes, as opposed to traditional one-shot batch systems, in which the model is trained only once. This paper addresses the issue of when to update or re-train a model, by proposing an approach to predict the performance metrics of the model if it were trained at a given moment, with a specific set of data. We validate the proposed approach in an interactive Machine Learning system in the domain of fraud detection.
更多查看译文
关键词
interactive learning scenarios,interactive learning,model training
AI 理解论文
溯源树
样例
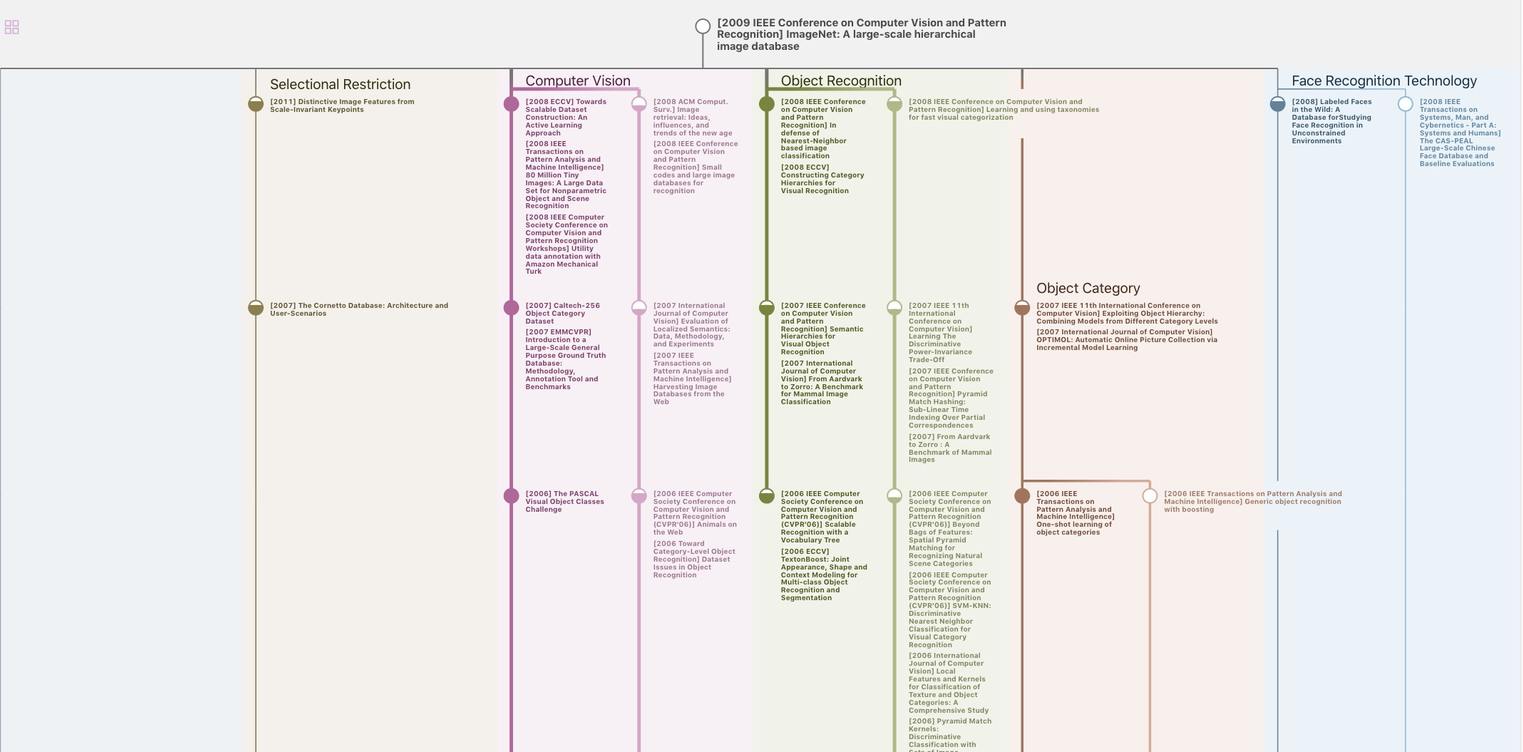
生成溯源树,研究论文发展脉络
Chat Paper
正在生成论文摘要