Early Prediction of student’s Performance in Higher Education: A Case Study
WorldCIST (1)(2021)
摘要
This work aims to contribute to the reduction of academic failure at higher education, by using machine learning techniques to identify students at risk of failure at an early stage of their academic path, so that strategies to support them can be put into place. A dataset from a higher education institution is used to build classification models to predict academic performance of students. The dataset includes information known at the time of student’s enrollment – academic path, demographics and social-economic factors. The problem is formulated as a three category classification task, in which there’s a strong imbalance towards one of the classes. Algorithms to promote class balancing with synthetic oversampling are tested, and classification models are trained and evaluated, both with standard machine learning algorithms and state of the art boosting algorithms. Our results show that boosting algorithms respond better to the specific classification task than standard methods. However, even these state of the art algorithms fall short in correctly identifying the majority of cases in one of the minority classes. Future directions of this study include the addition of information regarding student’s first year performance, such as academic grades from the first academic semesters.
更多查看译文
关键词
Academic performance,Machine learning in education,Imbalanced classes,Multi-class classification,Boosting methods
AI 理解论文
溯源树
样例
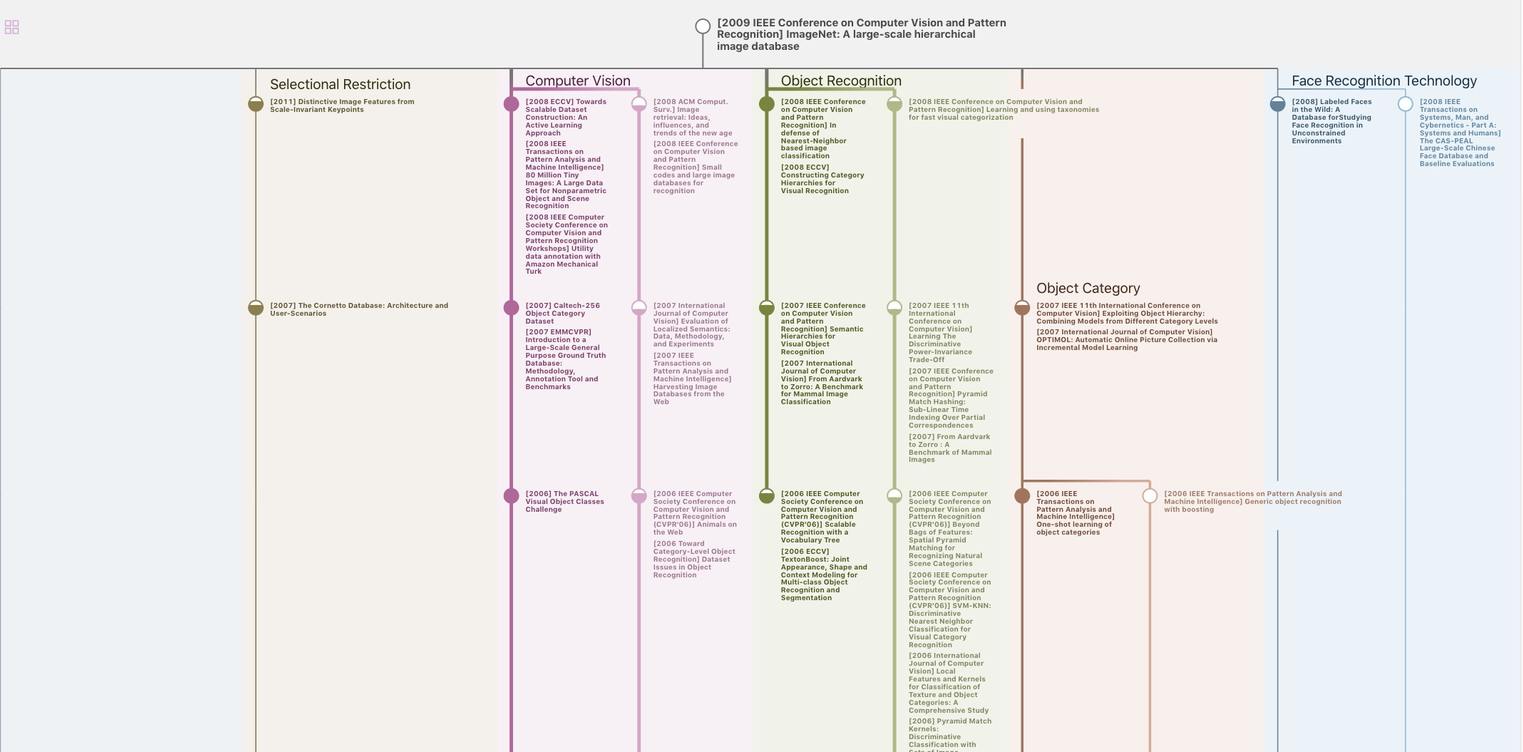
生成溯源树,研究论文发展脉络
Chat Paper
正在生成论文摘要