An Asymmetric Two-Sided Penalty Term for CT-GAN.
MMM (1)(2021)
摘要
Generative Adversarial Networks (GAN) is undoubtedly one of the most outstanding deep generation models in the tasks such as image-to-image translation and image generation. In recent years, many improved algorithms have been proposed for GAN, and Wasserstein GAN with a consistency term (CT-GAN) has been noticed because of excellent stability. However, we find that its penalty term is not only too restrictive, but also ignores the boundary region of model distribution. This makes it challenging to enforce 1-Lipschitz constraint required by CT-GAN. In this paper, we propose a more efficient and less restrictive penalty term, named asymmetric two-sided penalty, to enforce the 1-Lipischtz constraint. As a application, we introduce Wasserstein GAN with Asymmetric Two-sided Penalty (WGAN-AP). In addition, we use Spectral Normalization enforcing generator to enhance the stability of our model. Through experiments on image generation task, our WGAN-AP shows stability and superiority compared to the state-of-the-art methods in terms of Inception Score and Fréchet Inception Distance. In particular, The Inception Score of our WGAN-AP achieves 9.14 and 8.41 separately on CIFAR-10 dataset in supervised and unsupervised tasks, which exceeds that of state-of-the-art models.
更多查看译文
关键词
two-sided,ct-gan
AI 理解论文
溯源树
样例
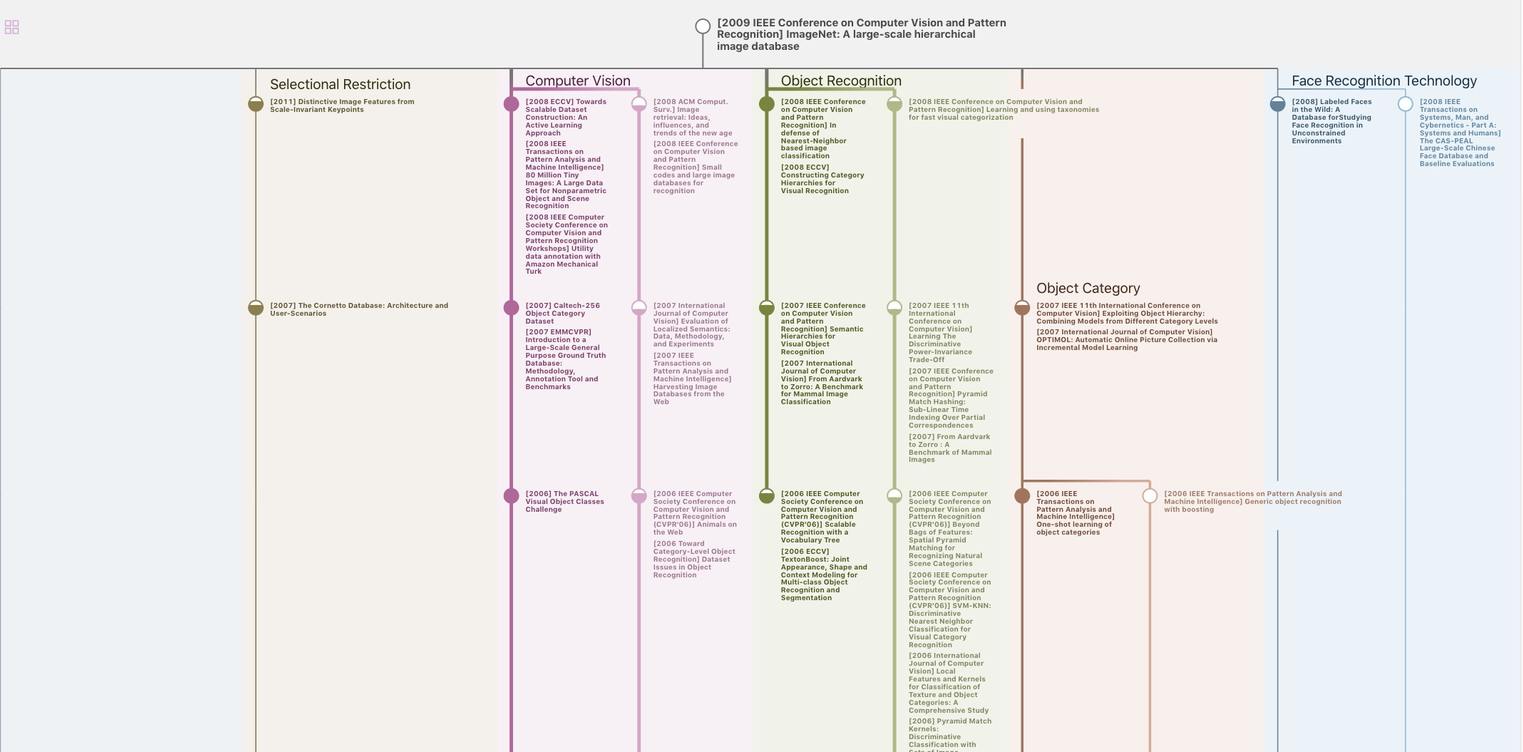
生成溯源树,研究论文发展脉络
Chat Paper
正在生成论文摘要