Normalizing flows for atomic solids
MACHINE LEARNING-SCIENCE AND TECHNOLOGY(2022)
摘要
We present a machine-learning approach, based on normalizing flows, for modelling atomic solids. Our model transforms an analytically tractable base distribution into the target solid without requiring ground-truth samples for training. We report Helmholtz free energy estimates for cubic and hexagonal ice modelled as monatomic water as well as for a truncated and shifted Lennard-Jones system, and find them to be in excellent agreement with literature values and with estimates from established baseline methods. We further investigate structural properties and show that the model samples are nearly indistinguishable from the ones obtained with molecular dynamics. Our results thus demonstrate that normalizing flows can provide high-quality samples and free energy estimates without the need for multi-staging.
更多查看译文
关键词
normalizing flows, atomic solids, free energy estimation
AI 理解论文
溯源树
样例
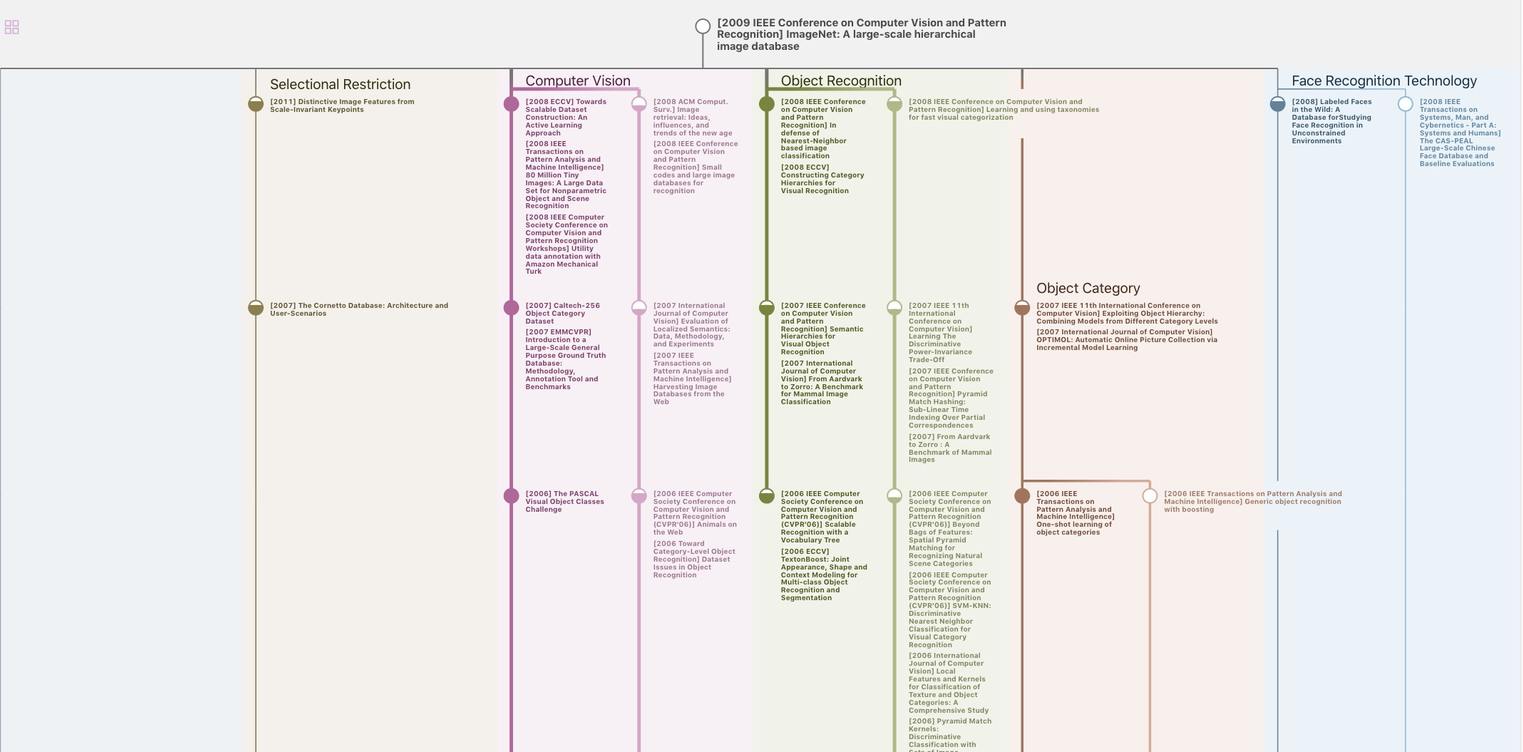
生成溯源树,研究论文发展脉络
Chat Paper
正在生成论文摘要