Non-separable Spatio-temporal Graph Kernels via SPDEs
INTERNATIONAL CONFERENCE ON ARTIFICIAL INTELLIGENCE AND STATISTICS, VOL 151(2022)
摘要
Gaussian processes (GPs) provide a principled and direct approach for inference and learning on graphs. However, the lack of justified graph kernels for spatio-temporal modelling has held back their use in graph problems. We leverage an explicit link between stochastic partial di.erential equations (SPDEs) and GPs on graphs, introduce a framework for deriving graph kernels via SPDEs, and derive non-separable spatio-temporal graph kernels that capture interaction across space and time. We formulate the graph kernels for the stochastic heat equation and wave equation. We show that by providing novel tools for spatio-temporal GP modelling on graphs, we outperform pre-existing graph kernels in realworld applications that feature di.usion, oscillation, and other complicated interactions.
更多查看译文
关键词
spdes,kernels,graph,non-separable,spatio-temporal
AI 理解论文
溯源树
样例
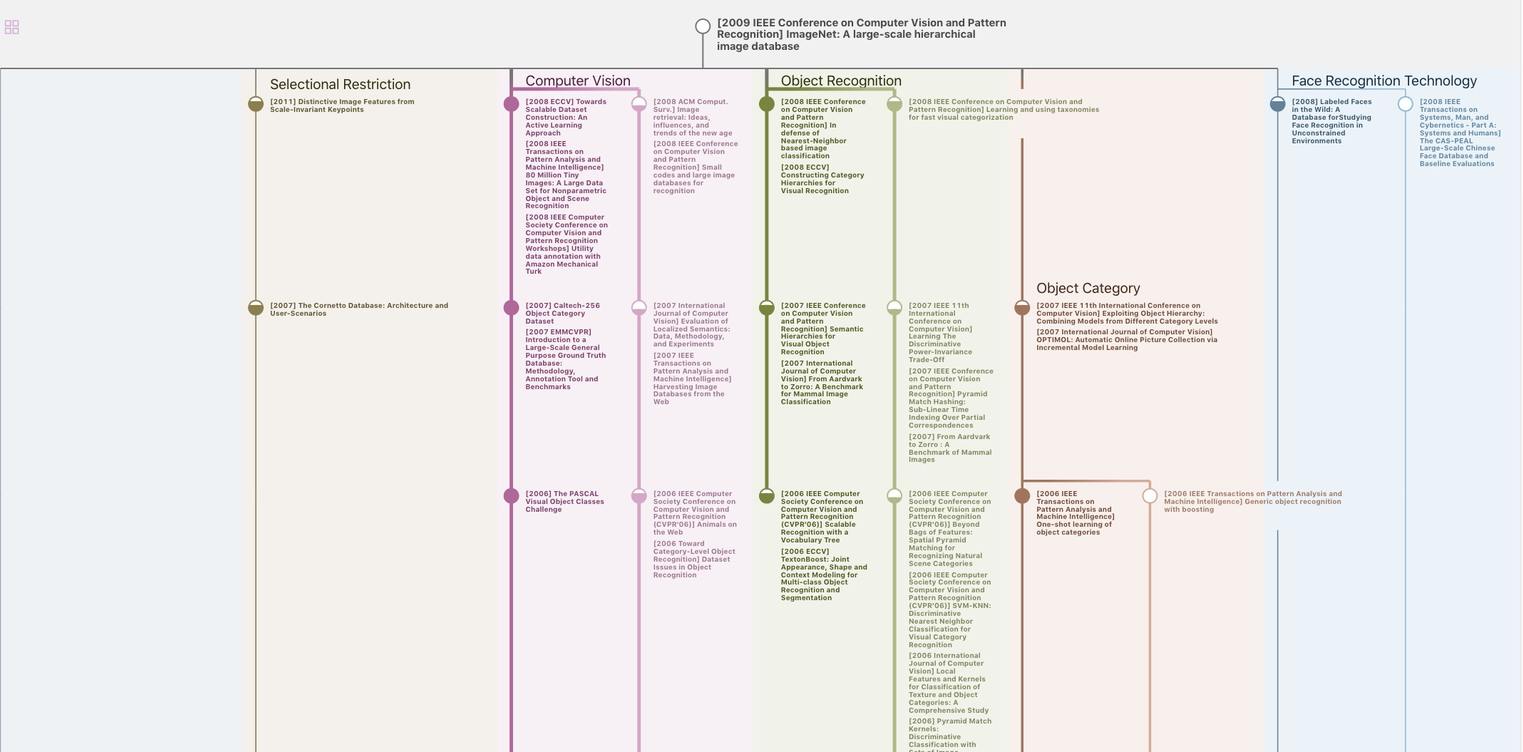
生成溯源树,研究论文发展脉络
Chat Paper
正在生成论文摘要