QA4PRF: A Question Answering Based Framework for Pseudo Relevance Feedback
IEEE ACCESS(2021)
Abstract
Pseudo relevance feedback (PRF) automatically performs query expansion based on top-retrieved documents to better represent the user's information need so as to improve the search results. Previous PRF methods mainly select expansion terms with high occurrence frequency in top-retrieved documents or with high semantic similarity with the original query. However, existing PRF methods hardly try to understand the content of documents, which is very important in performing effective query expansion to reveal the user's information need. In this paper, we propose a QA-based framework for PRF called QA4PRF to utilize contextual information in documents. In such a framework, we formulate PRF as a QA task, where the query and each top-retrieved document play the roles of question and context in the corresponding QA system, while the objective is to find some proper terms to expand the original query by utilizing contextual information, which are similar answers in QA task. Besides, an attention-based pointer network is built on understanding the content of top-retrieved documents and selecting the terms to represent the original query better. We also show that incorporating the traditional supervised learning methods, such as LambdaRank, to integrate PRF information will further improve the performance of QA4PRF. Extensive experiments on three real-world datasets demonstrate that QA4PRF significantly outperforms the state-of-the-art methods.
MoreTranslated text
Key words
Task analysis,Semantics,Supervised learning,Knowledge discovery,Licenses,Text analysis,Support vector machines,Pseudo relevance feedback,question answering,query expansion
AI Read Science
Must-Reading Tree
Example
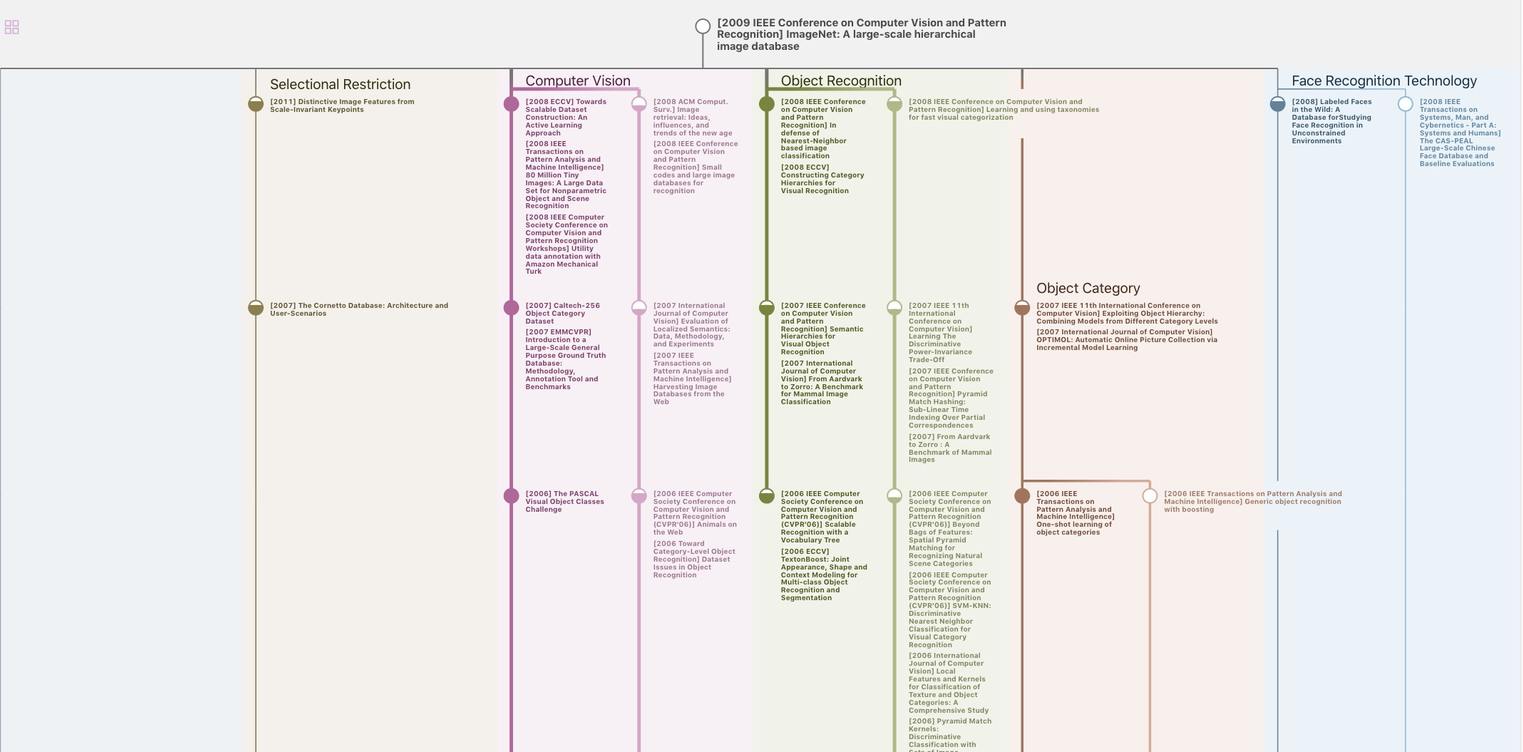
Generate MRT to find the research sequence of this paper
Chat Paper
Summary is being generated by the instructions you defined