Fast and credible likelihood-free cosmology with truncated marginal neural ratio estimation
JOURNAL OF COSMOLOGY AND ASTROPARTICLE PHYSICS(2022)
摘要
Sampling-based inference techniques are central to modern cosmological data analysis; these methods, however, scale poorly with dimensionality and typically require approximate or intractable likelihoods. In this paper we describe how Truncated Marginal Neural Ratio Estimation (TMNRE) (a new approach in so-called simulation-based inference) naturally evades these issues, improving the (i) efficiency, (ii) scalability, and (iii) trustworthiness of the inference. Using measurements of the Cosmic Microwave Background (CMB), we show that TMNRE can achieve converged posteriors using orders of magnitude fewer simulator calls than conventional Markov Chain Monte Carlo (MLML) methods. Remarkably, in these examples the required number of samples is effectively independent of the number of nuisance parameters. In addition, a property called local amortization allows the performance of rigorous statistical consistency checks that are not accessible to sampling-based methods. TMNRE promises to become a powerful tool for cosmological data analysis, particularly in the context of extended cosmologies, where the timescale required for conventional sampling-based inference methods to converge can greatly exceed that of simple cosmological models such as ACDM. To perform these computations, we use an implementation of TMNRE via the open-source code swyft.1
更多查看译文
关键词
Bayesian reasoning, Machine learning, Statistical sampling techniques, cosmo-logical parameters from CMBR
AI 理解论文
溯源树
样例
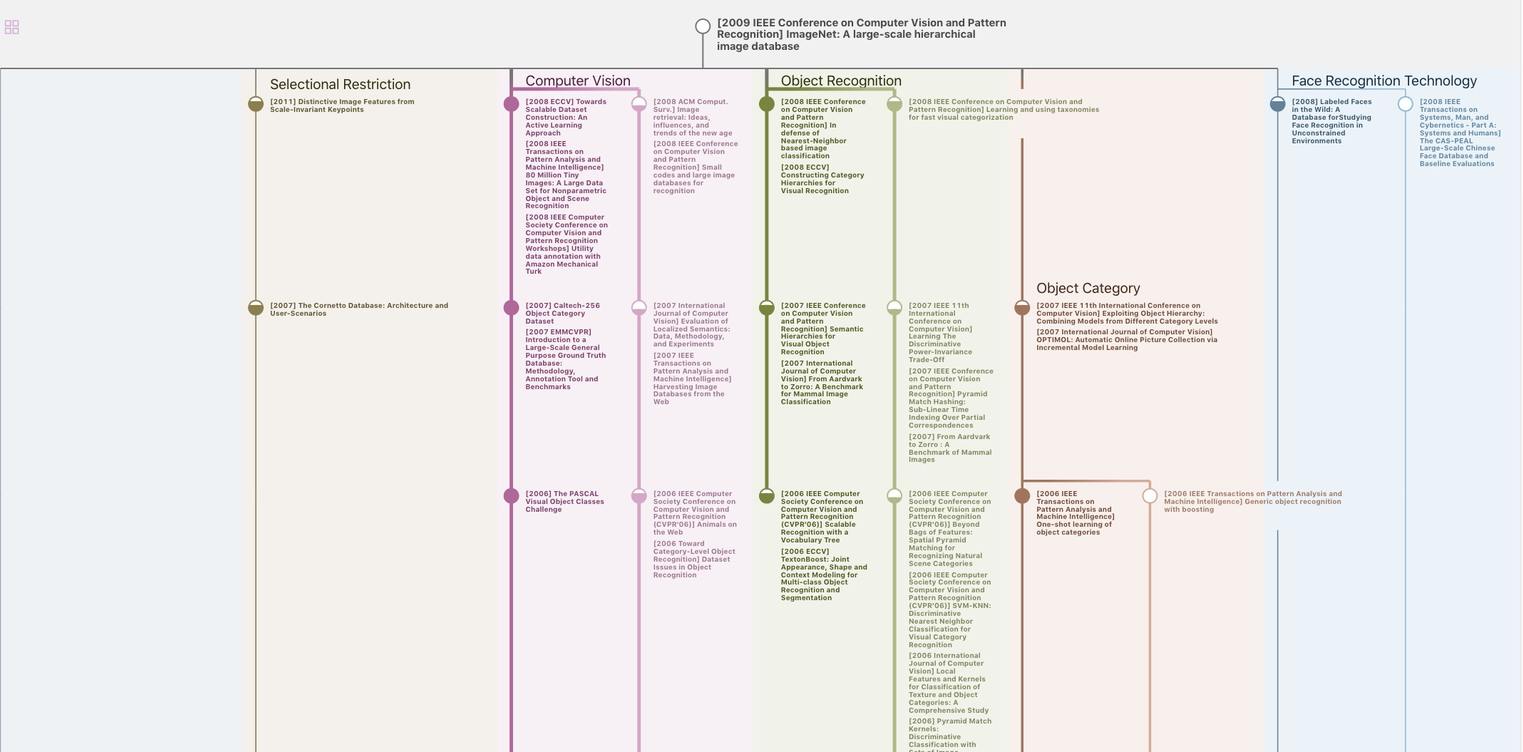
生成溯源树,研究论文发展脉络
Chat Paper
正在生成论文摘要