Contrastive Feature Loss for Image Prediction.
ICCVW(2021)
摘要
Training supervised image synthesis models requires a critic to compare two images: the ground truth to the result. Yet, this basic functionality remains an open problem. A popular line of approaches uses the L1 (mean absolute error) loss, either in the pixel or the feature space of pretrained deep networks. However, we observe that these losses tend to produce overly blurry and grey images, and other techniques such as GANs need to be employed to fight these artifacts. In this work, we introduce an information theory based approach to measuring similarity between two images. We argue that a good reconstruction should have high mutual information with the ground truth. This view enables learning a lightweight critic to "calibrate" a feature space in a contrastive manner, such that reconstructions of corresponding spatial patches are brought together, while other patches are repulsed. We show that our formulation immediately boosts the perceptual realism of output images when used as a drop-in replacement for the L1 loss, with or without an additional GAN loss.
更多查看译文
关键词
contrastive feature loss,image prediction,supervised image synthesis models,ground truth,basic functionality,open problem,L1 loss,mean absolute error,feature space,pretrained deep networks,overly blurry images,grey images,information theory,high mutual information,lightweight critic,contrastive manner,GAN loss,spatial patches
AI 理解论文
溯源树
样例
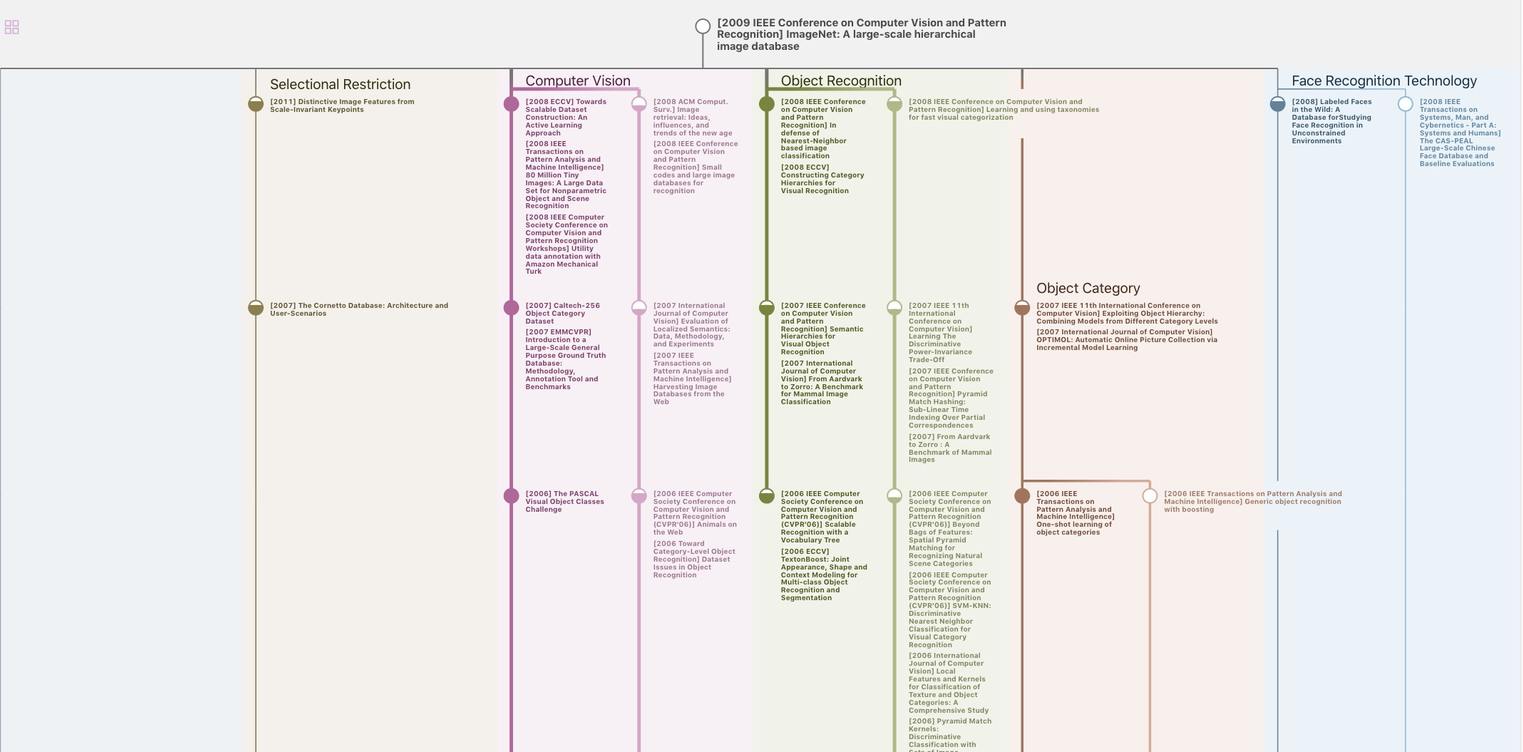
生成溯源树,研究论文发展脉络
Chat Paper
正在生成论文摘要