Deep Reinforcement Model Selection for Communications Resource Allocation in On-Site Medical Care
2022 IEEE Wireless Communications and Networking Conference (WCNC)(2022)
摘要
Greater capabilities of mobile communications technology enable the interconnection of on-site medical care at a scale previously unavailable. However, embedding such critical, demanding tasks into the already complex infrastructure of mobile communications has proven challenging. This paper explores a resource allocation scenario where a scheduler must balance mixed performance metrics among connected users. To fulfill this resource allocation task, we present a scheduler that adaptively switches between different model-based scheduling algorithms. We make use of a deep Q-Network (DQN) to learn the benefit of selecting a scheduling paradigm for a given situation, combining advantages from model-driven and data-driven approaches. The resulting ensemble scheduler is able to combine its constituent algorithms to maximize a sum-utility cost function while ensuring performance on designated high-priority users.
更多查看译文
关键词
Allocation,DQN,adaptive,model-selection,5G,scheduling
AI 理解论文
溯源树
样例
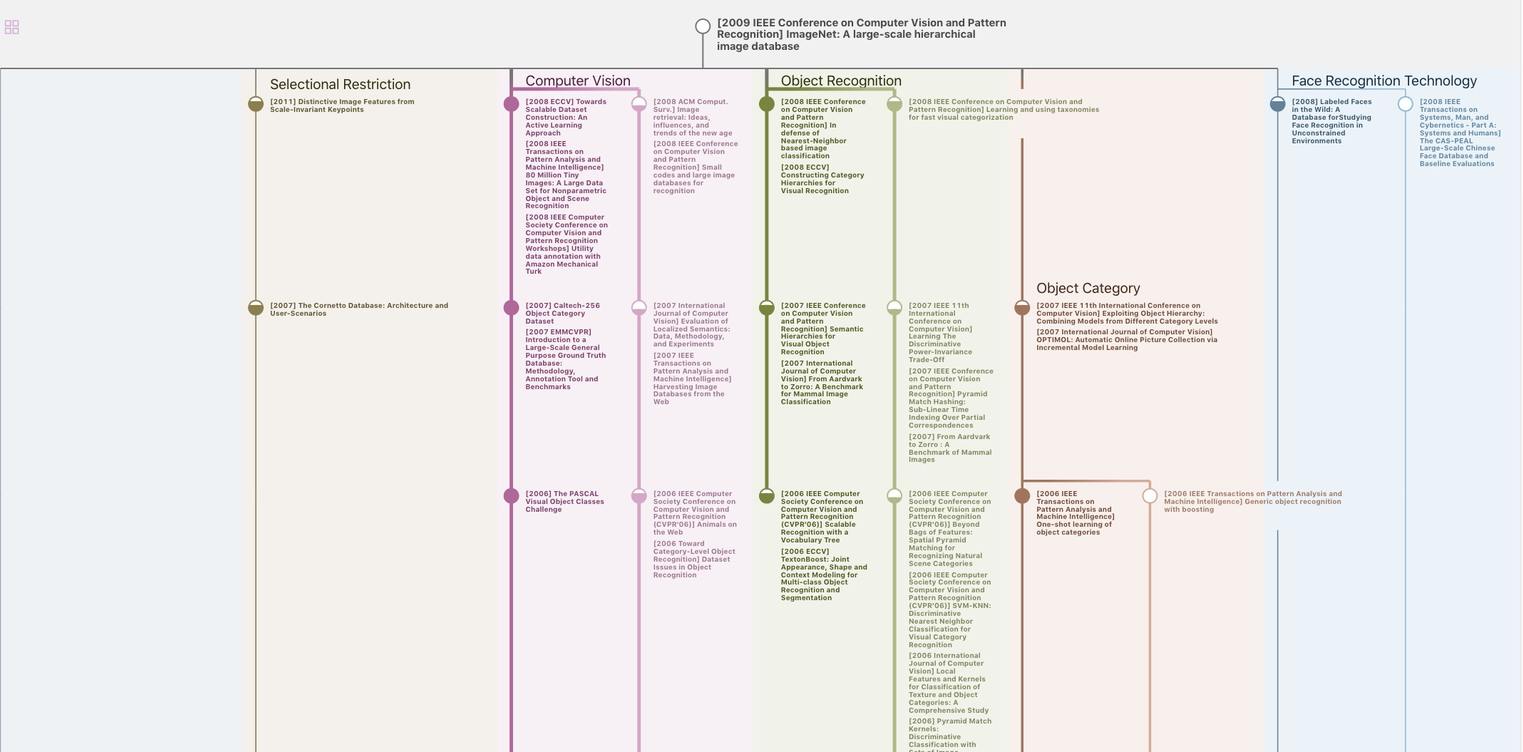
生成溯源树,研究论文发展脉络
Chat Paper
正在生成论文摘要