Disentangling physical parameters for anomalous sound detection under domain shifts
2022 30th European Signal Processing Conference (EUSIPCO)(2022)
摘要
To develop a sound-monitoring system for machines, a method for detecting anomalous sound under domain shifts is proposed. A domain shift occurs when a machine's physical parameters change. Because a domain shift changes the distribution of normal sound data, conventional unsupervised anomaly detection methods can output false positives. To solve this problem, the proposed method constrains some latent variables of a normalizing flows (NF) model to represent physical parameters, which enables disentanglement of the factors of domain shifts and learning of a latent space that is invariant with respect to these domain shifts. Anomaly scores calculated from this domain-shift-invariant latent space are unaffected by such shifts, which reduces false positives and improves the detection performance. Experiments were conducted with sound data from a slide rail under different operation velocities. The results show that the proposed method disentangled the velocity to obtain a latent space that was invariant with respect to domain shifts, which improved the AUC by 13.2% for Glow with a single block and 2.6% for Glow with multiple blocks.
更多查看译文
关键词
Machine health monitoring,Anomalous sound detection,Anomaly detection,Semi-supervised disentanglement learning,Normalizing flows
AI 理解论文
溯源树
样例
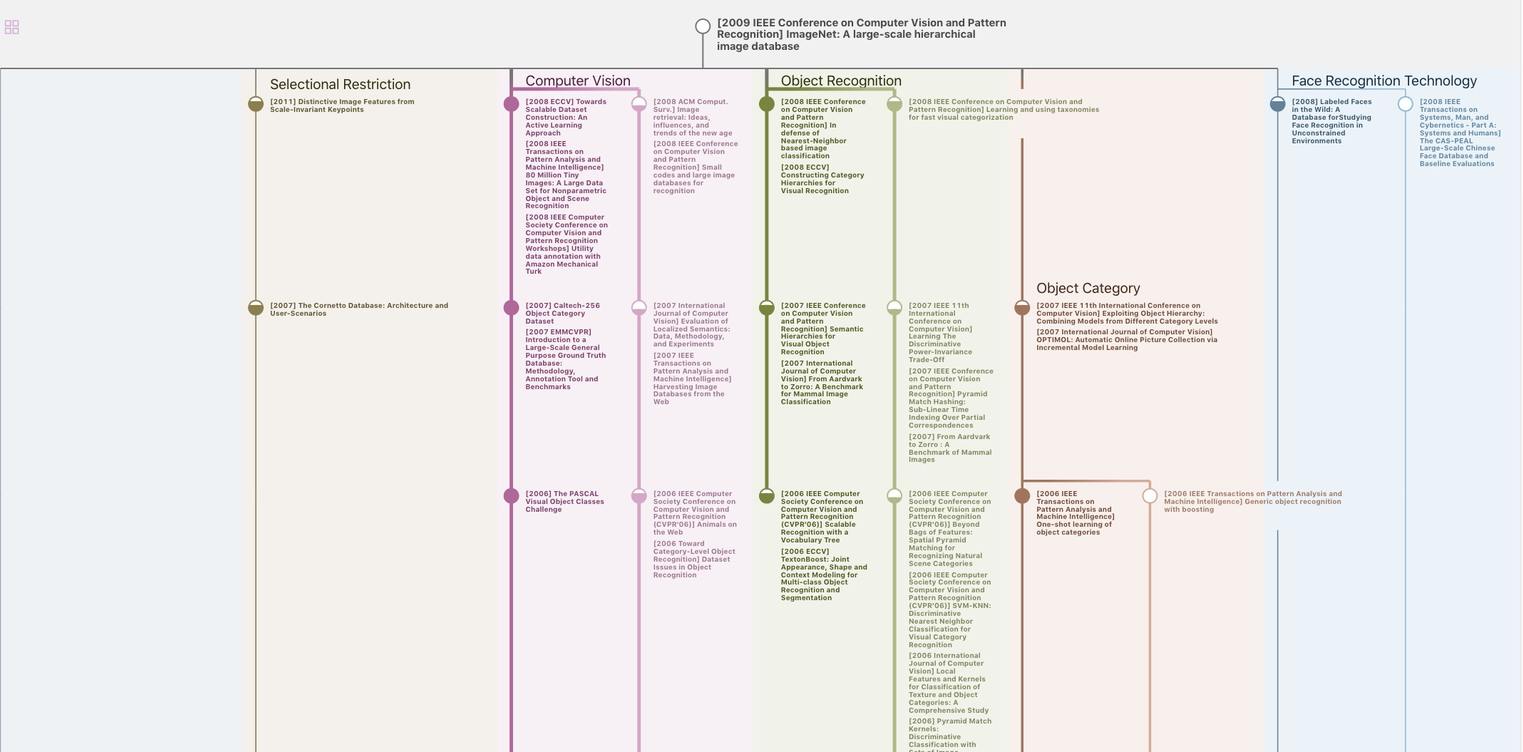
生成溯源树,研究论文发展脉络
Chat Paper
正在生成论文摘要