Kernel recursive least square tracker and long-short term memory ensemble based battery health prognostic model
iScience(2021)
摘要
A data-driven approach is developed to predict the future capacity of lithium-ion batteries (LIBs) in this work. The empirical mode decomposition (EMD), kernel recursive least square tracker (KRLST), and long short-term memory (LSTM) are used to derive the proposed approach. First, the LIB capacity data is split into local regeneration and monotonic global degradation using the EMD approach. Next, the KRLST is used to track the decomposed intrinsic mode functions, and the residual signal is predicted using the LSTM sub-model. Finally, all the predicted intrinsic mode functions and the residual are ensembled to get the future capacity. The experimental and comparative analysis validates the high accuracy (RMSE of 0.00103) of the proposed ensemble approach compared to Gaussian process regression and LSTM fused model. Furthermore, two times lesser error than other fused models makes this approach an efficient tool for battery health prognostics.
更多查看译文
关键词
Computer systems organization,Energy engineering,Energy systems
AI 理解论文
溯源树
样例
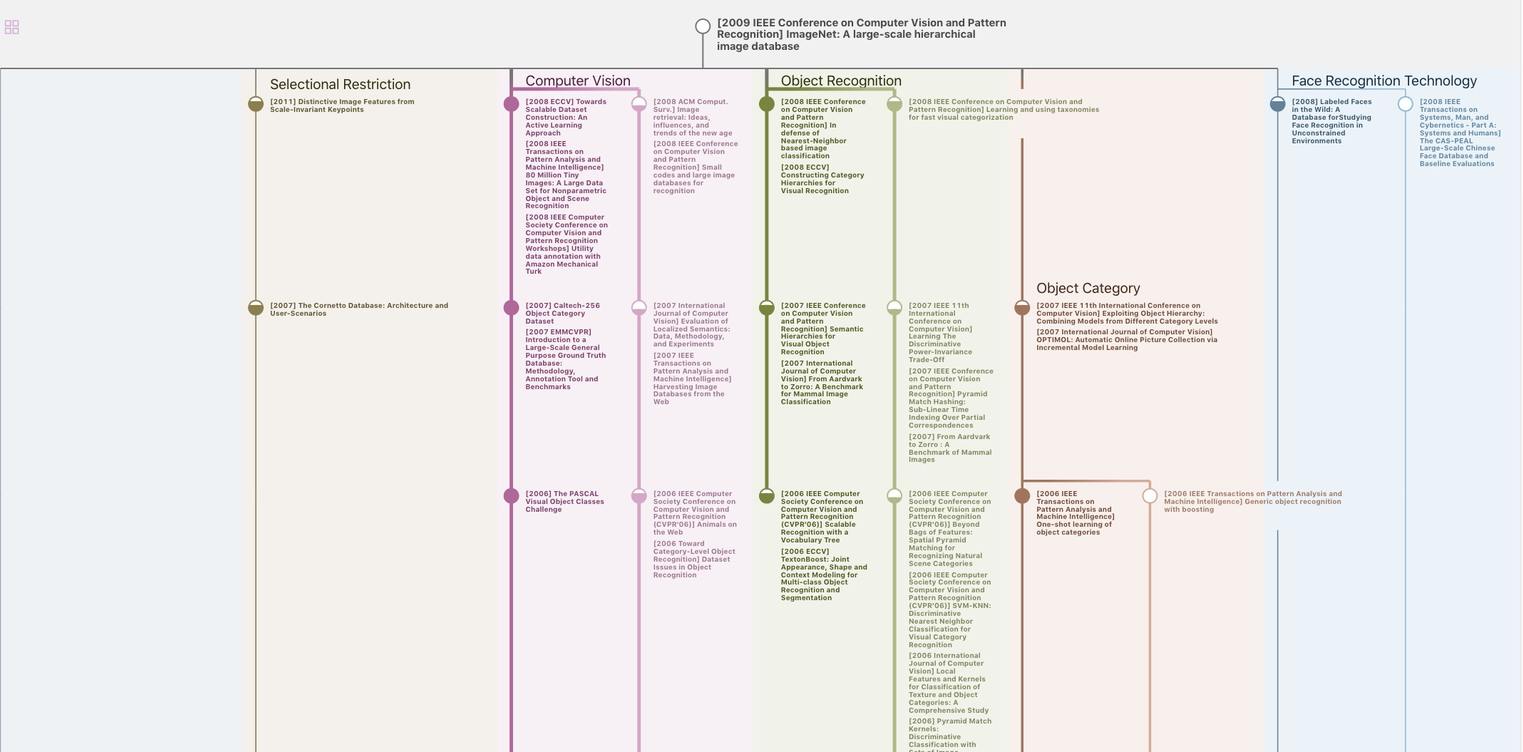
生成溯源树,研究论文发展脉络
Chat Paper
正在生成论文摘要