Self-Normalized Importance Sampling for Neural Language Modeling.
Conference of the International Speech Communication Association (INTERSPEECH)(2022)
摘要
To mitigate the problem of having to traverse over the full vocabulary in the softmax normalization of a neural language model, sampling-based training criteria are proposed and investigated in the context of large vocabulary word-based neural language models. These training criteria typically enjoy the benefit of faster training and testing, at a cost of slightly degraded performance in terms of perplexity and almost no visible drop in word error rate. While noise contrastive estimation is one of the most popular choices, recently we show that other sampling-based criteria can also perform well, as long as an extra correction step is done, where the intended class posterior probability is recovered from the raw model outputs. In this work, we propose self-normalized importance sampling. Compared to our previous work, the criteria considered in this work are self-normalized and there is no need to further conduct a correction step. Compared to noise contrastive estimation, our method is directly comparable in terms of complexity in application. Through self-normalized language model training as well as lattice rescoring experiments, we show that our proposed self-normalized importance sampling is competitive in both research-oriented and production-oriented automatic speech recognition tasks.
更多查看译文
关键词
self-normalization, importance sampling, training criterion, neural network, language modeling
AI 理解论文
溯源树
样例
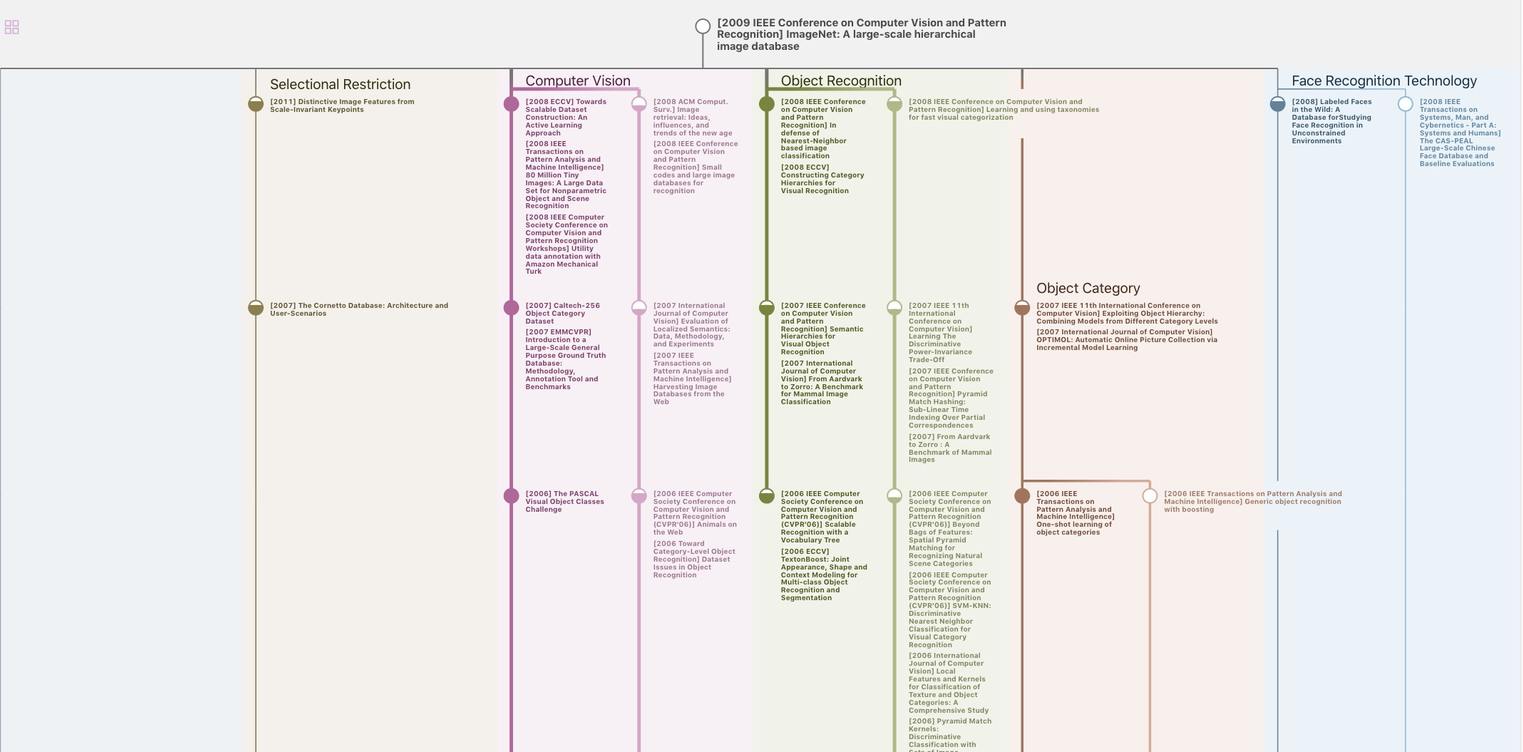
生成溯源树,研究论文发展脉络
Chat Paper
正在生成论文摘要