A Secure and Efficient Federated Learning Framework for NLP.
EMNLP(2021)
摘要
In this work, we consider the problem of designing secure and efficient federated learning (FL) frameworks. Existing solutions either involve a trusted aggregator or require heavyweight cryptographic primitives, which degrades performance significantly. Moreover, many existing secure FL designs work only under the restrictive assumption that none of the clients can be dropped out from the training protocol. To tackle these problems, we propose SEFL, a secure and efficient FL framework that (1) eliminates the need for the trusted entities; (2) achieves similar and even better model accuracy compared with existing FL designs; (3) is resilient to client dropouts. Through extensive experimental studies on natural language processing (NLP) tasks, we demonstrate that the SEFL achieves comparable accuracy compared to existing FL solutions, and the proposed pruning technique can improve runtime performance up to 13.7x.
更多查看译文
关键词
efficient federated learning framework,nlp,secure
AI 理解论文
溯源树
样例
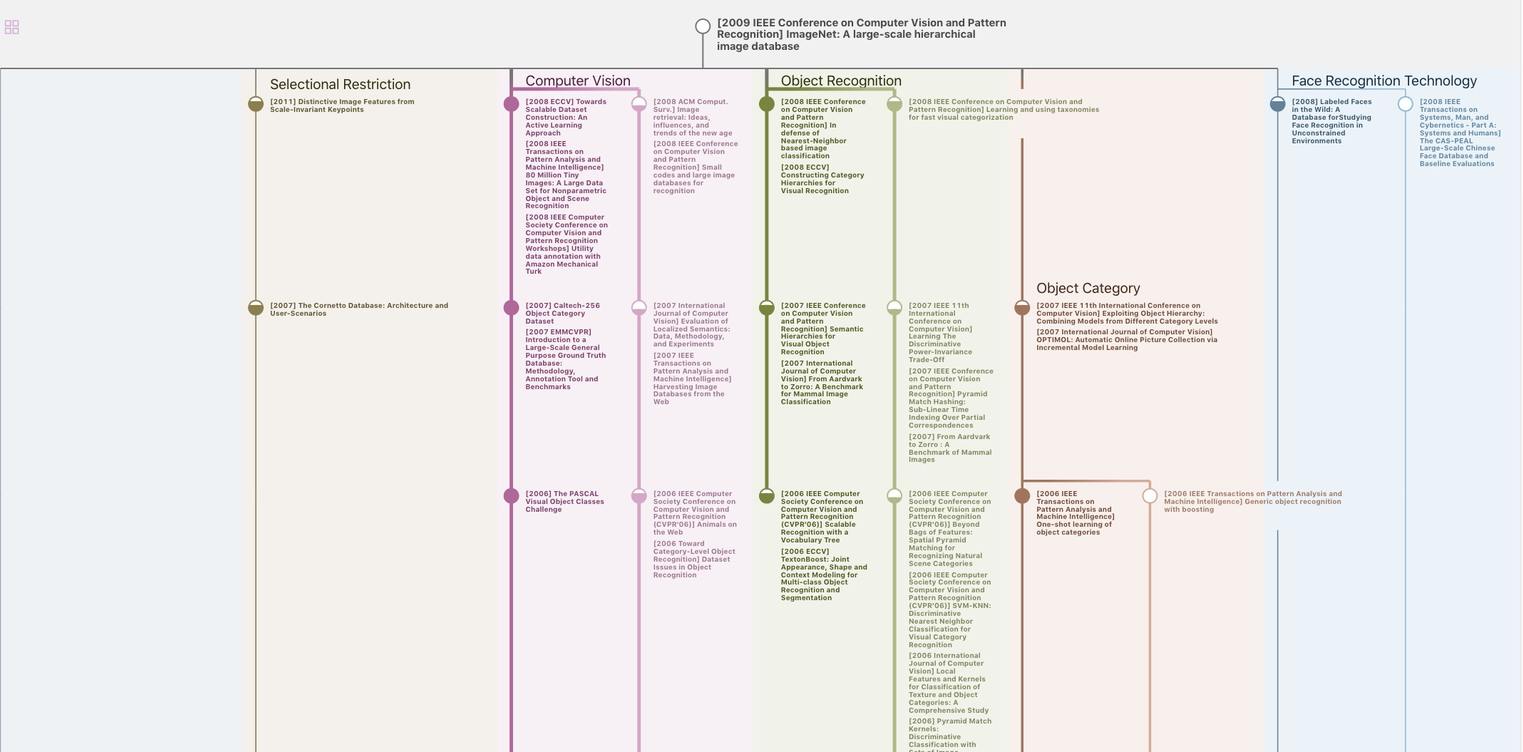
生成溯源树,研究论文发展脉络
Chat Paper
正在生成论文摘要