Leveraging Capsule Routing to Associate Knowledge with Medical Literature Hierarchically
2021 CONFERENCE ON EMPIRICAL METHODS IN NATURAL LANGUAGE PROCESSING (EMNLP 2021)(2021)
摘要
Integrating knowledge into text is a promising way to enrich text representation, especially in the medical field. However, undifferentiated knowledge not only confuses the text representation but also imports unexpected noises. In this paper, to alleviate this problem, we propose leveraging capsule routing to associate knowledge with medical literature hierarchically (called HiCapsRKL). Firstly, HiCapsRKL extracts two empirically designed text fragments from medical literature and encodes them into fragment representations respectively. Secondly, the capsule routing algorithm is applied to two fragment representations. Through the capsule computing and dynamic routing, each representation is processed into a new representation (denoted as caps-representation), and we integrate the caps-representations as information gain to associate knowledge with medical literature hierarchically. Finally, HiCapsRKL are validated on relevance prediction and medical literature retrieval test sets. The experimental results and analyses show that HiCapsRKL can more accurately associate knowledge with medical literature than the mainstream methods. In summary, HiCapsRKL can efficiently help selecting the most relevant knowledge to the medical literature, which may be an alternative attempt to improve knowledge-based text representation. Source code is released on GitHub(1).
更多查看译文
AI 理解论文
溯源树
样例
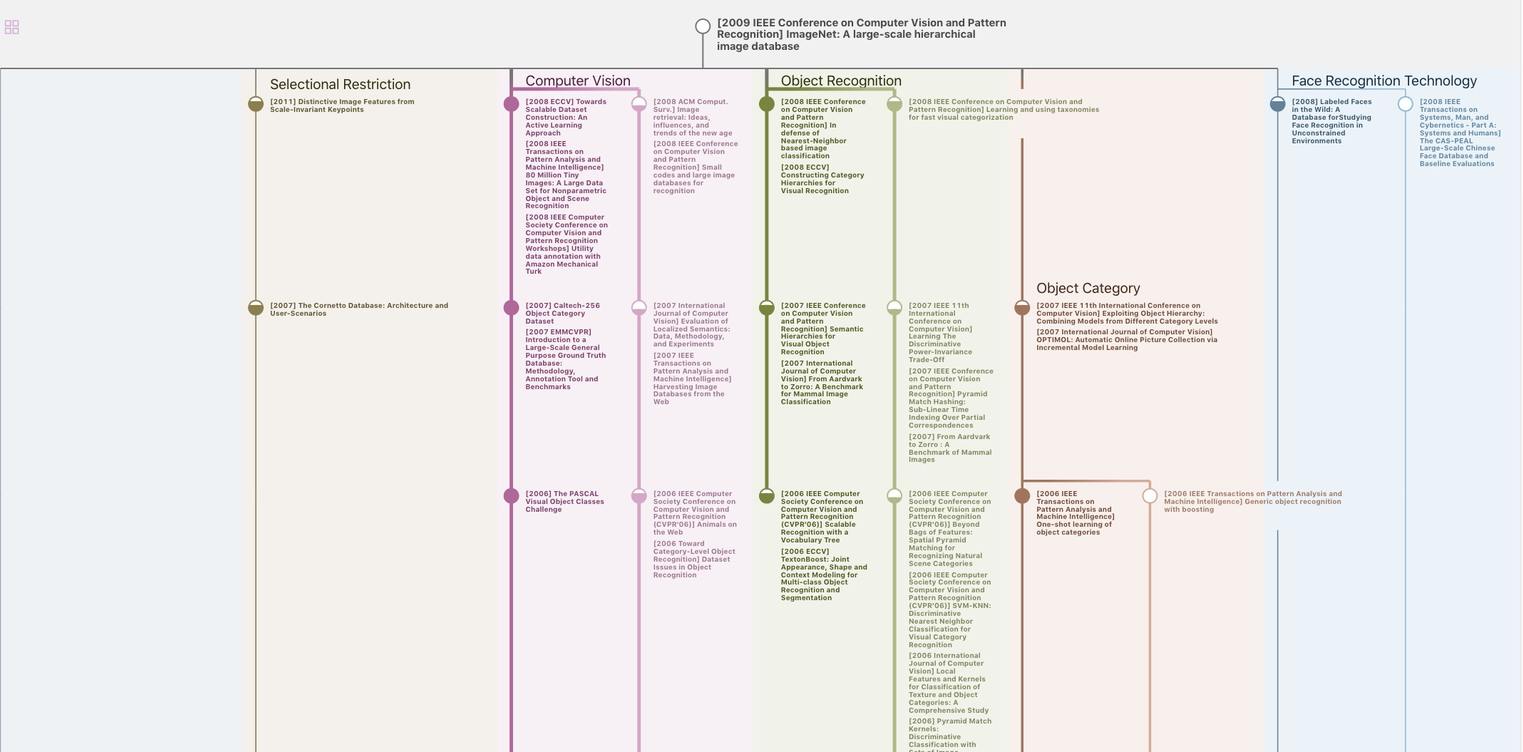
生成溯源树,研究论文发展脉络
Chat Paper
正在生成论文摘要