Progressive anatomically constrained deep neural network for 3D deformable medical image registration
Neurocomputing(2021)
摘要
The 3D deformable image registration is one of the most challenging tasks in medical image analysis. Due to the large and complex deformation in 3D medical images, many deep neural network based methods have been proposed to improve the image similarity after registration, among which recursive cascading network structure is one of the state-of-the-art. However, most existing works rely on the pixel-level image similarities to achieve anatomical rationality and overlook the global-level resemblance between the two structures. Therefore, the resulting registration is not quite clinically valuable. To this end, in this work, we propose a Progressive Anatomically Constrained deep neural Network (PACN) to incorporate the anatomical priors into a progressive cascading registration network to improve the anatomical plausibility as well as the pixel-level similarity of the registration results. Specifically, an Anatomical Constraint Encoder (ACE) network is proposed to encode the global context of the anatomical segmentations and attached to the dense registration network to form a registration unit. Repeated such units forming a cascading framework progressively warps the moving image toward the fixed one, with the output warped image of one unit as the input of the next unit. In this design, the global anatomical priors along with the pixel-level local information are used to guide the model learning process to produce high quality deformation field. Based on this, we explore two frameworks to investigate their registration effectiveness, one attaches the anatomical constraint encoder (ACE) to every dense registration sub-network and the other one attaches ACE only to the last dense registration unit. We test the two frameworks on benchmarks of three liver image datasets SLIVER, LiTS and LSPIG, and one brain dataset LPBA. Our two frameworks have achieved significantly better results in terms of average Dice score than the state-of-the-art baseline method on three liver datasets and comparable on LPBA when both tested with up to three cascades.
更多查看译文
关键词
Deformable medical image registration,Anatomical constraint network,Denoising auto-encoder,Progressive cascading registration network
AI 理解论文
溯源树
样例
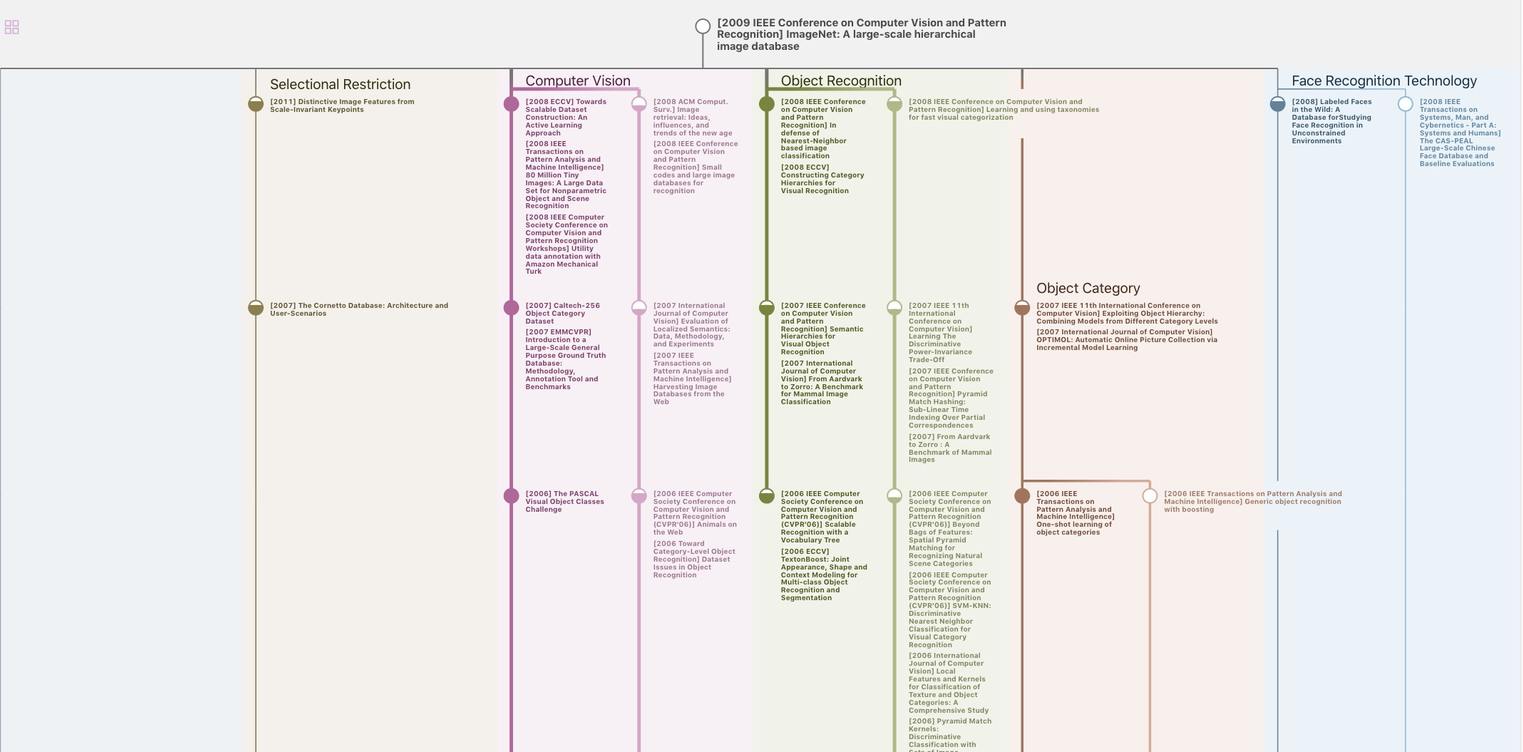
生成溯源树,研究论文发展脉络
Chat Paper
正在生成论文摘要