On the non-recursive implementation of multistage sampling without replacement
METHODSX(2021)
摘要
Variance estimation in multistage sampling without replacement usually requires considerable computational effort. One option is to implement explicit formulas on a computer, at least for some specific sampling designs. This approach becomes quite cumbersome to handle beyond two stages, both from the formulation and computer implementation points of view. Another option is to provide a general method to compute variance estimates for any number of stages. Such an approach may involve data structures and estimators which are recursively defined. The solution we present in this article is intended to be both general and computationally efficient by relying on a full-iterative implementation. The definition of the estimators remains implicit as in the recursive approach, but is expressed in terms of recurrence relations translated into iterative algorithms. These algorithms rely only on (dense) array data structures. Moreover, most of the necessary computer memory is only used during preliminary steps and is not required when performing the statistical calculations. (C) 2021 The Author(s). Published by Elsevier B.V.
更多查看译文
关键词
Multistage sampling, Horvitz-Thompson estimator, Expansion estimator, Sen-Yates-Grundy variance, Approximate variance estimator, Self-weighted design, Array data structures, Non-recursive algorithms
AI 理解论文
溯源树
样例
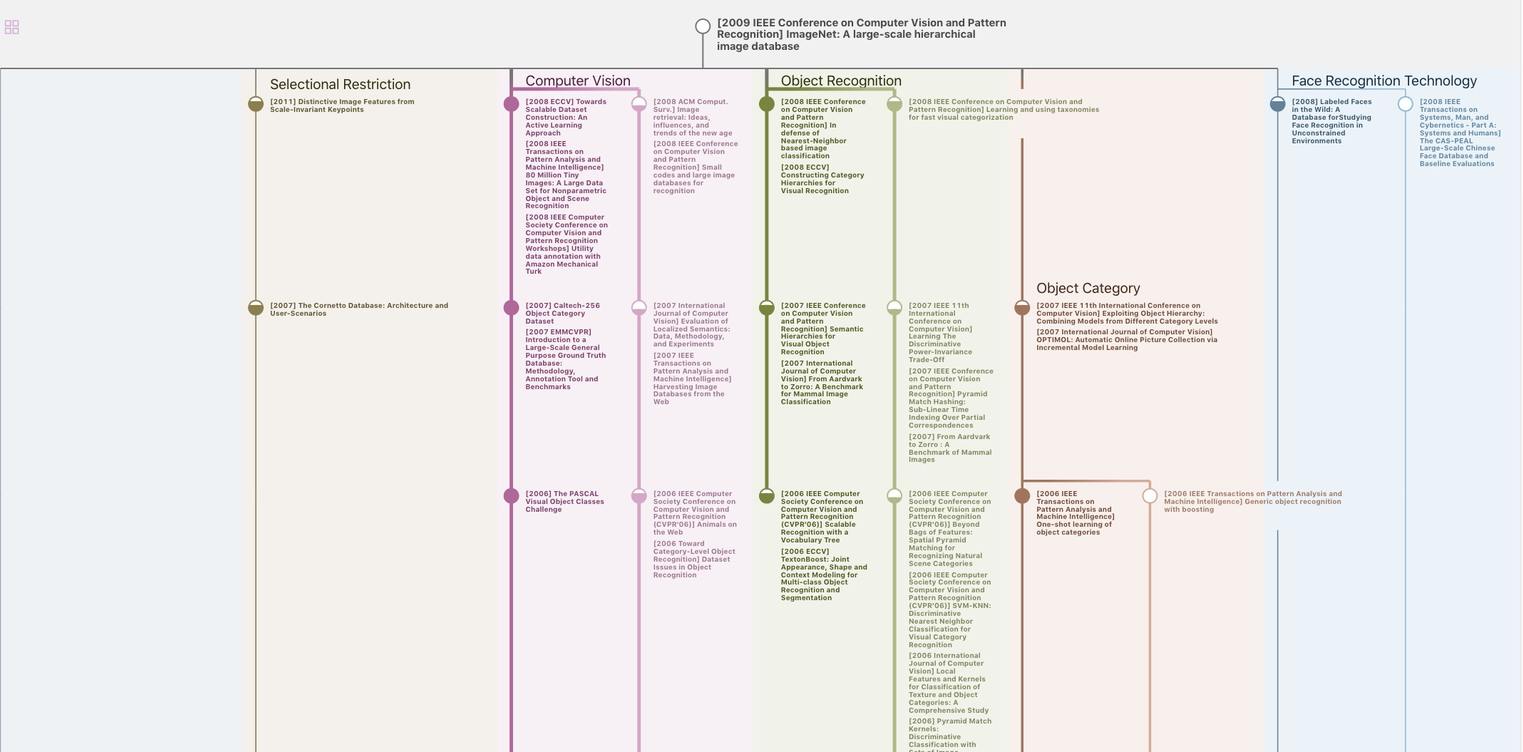
生成溯源树,研究论文发展脉络
Chat Paper
正在生成论文摘要