Fine-grained Information Extraction from Biomedical Literature based on Knowledge-enriched Abstract Meaning Representation
Annual Meeting of the Association for Computational Linguistics(2021)
摘要
Event Detection (ED) aims to recognize mentions of events (i.e., event triggers) and their types in text. Recently, several ED datasets in various domains have been proposed. However, the major limitation of these resources is the lack of enough training data for individual event types which hinders the efficient training of data-hungry deep learning models. To overcome this issue, we propose to exploit the powerful pre-trained language model GPT-2 to generate training samples for ED. To prevent the noises inevitable in automatically generated data from hampering training process, we propose to exploit a teacher-student architecture in which the teacher is supposed to learn anchor knowledge from the original data. The student is then trained on combination of the original and GPT-generated data while being led by the anchor knowledge from the teacher. Optimal transport is introduced to facilitate the anchor knowledge-based guidance between the two networks. We evaluate the proposed model on multiple ED benchmark datasets, gaining consistent improvement and establishing state-of-the-art results for ED.
更多查看译文
关键词
information extraction,abstract meaning representation,biomedical literature,fine-grained,knowledge-enriched
AI 理解论文
溯源树
样例
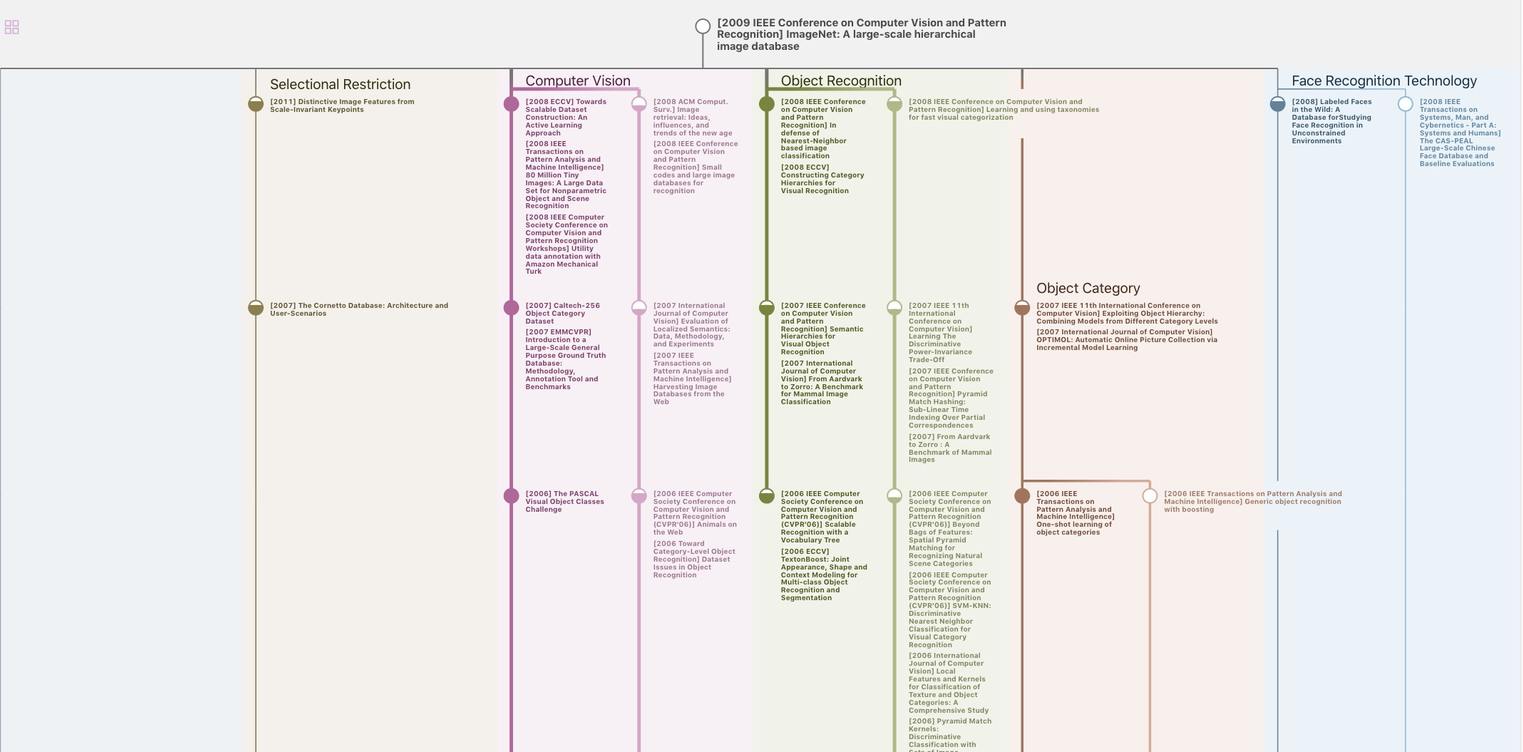
生成溯源树,研究论文发展脉络
Chat Paper
正在生成论文摘要