TomoSLAM: Factor Graph Optimization for Rotation Angle Refinement in Microtomography
FOURTEENTH INTERNATIONAL CONFERENCE ON MACHINE VISION (ICMV 2021)(2022)
摘要
In computed tomography (CT), the relative trajectories of a sample, a detector, and a signal source are traditionally considered to be known, since they are caused by the intentional preprogrammed movement of the instrument parts. However, due to the mechanical backlashes, rotation sensor measurement errors, thermal deformations real trajectory differs from desired ones. This negatively affects the resulting quality of tomographic reconstruction. Neither the calibration nor preliminary adjustments of the device completely eliminates the inaccuracy of the trajectory but significantly increase the cost of instrument maintenance. A number of approaches to this problem are based on an automatic refinement of the source and sensor position estimate relative to the sample for each projection (at each time step) during the reconstruction process. A similar problem of position refinement while observing different images of an object from different angles is well known in robotics (particularly, in mobile robots and self-driving vehicles) and is called Simultaneous Localization And Mapping (SLAM). The scientific novelty of this work is to consider the problem of trajectory refinement in microtomography as a SLAM problem. This is achieved by extracting Speeded Up Robust Features (SURF) features from X-ray projections, filtering matches with Random Sample Consensus (RANSAC), calculating angles between projections, and using them in factor graph in combination with stepper motor control signals in order to refine rotation angles.
更多查看译文
关键词
rotation angle estimation, computed tomography, SLAM, factor graph, digital X-ray imaging
AI 理解论文
溯源树
样例
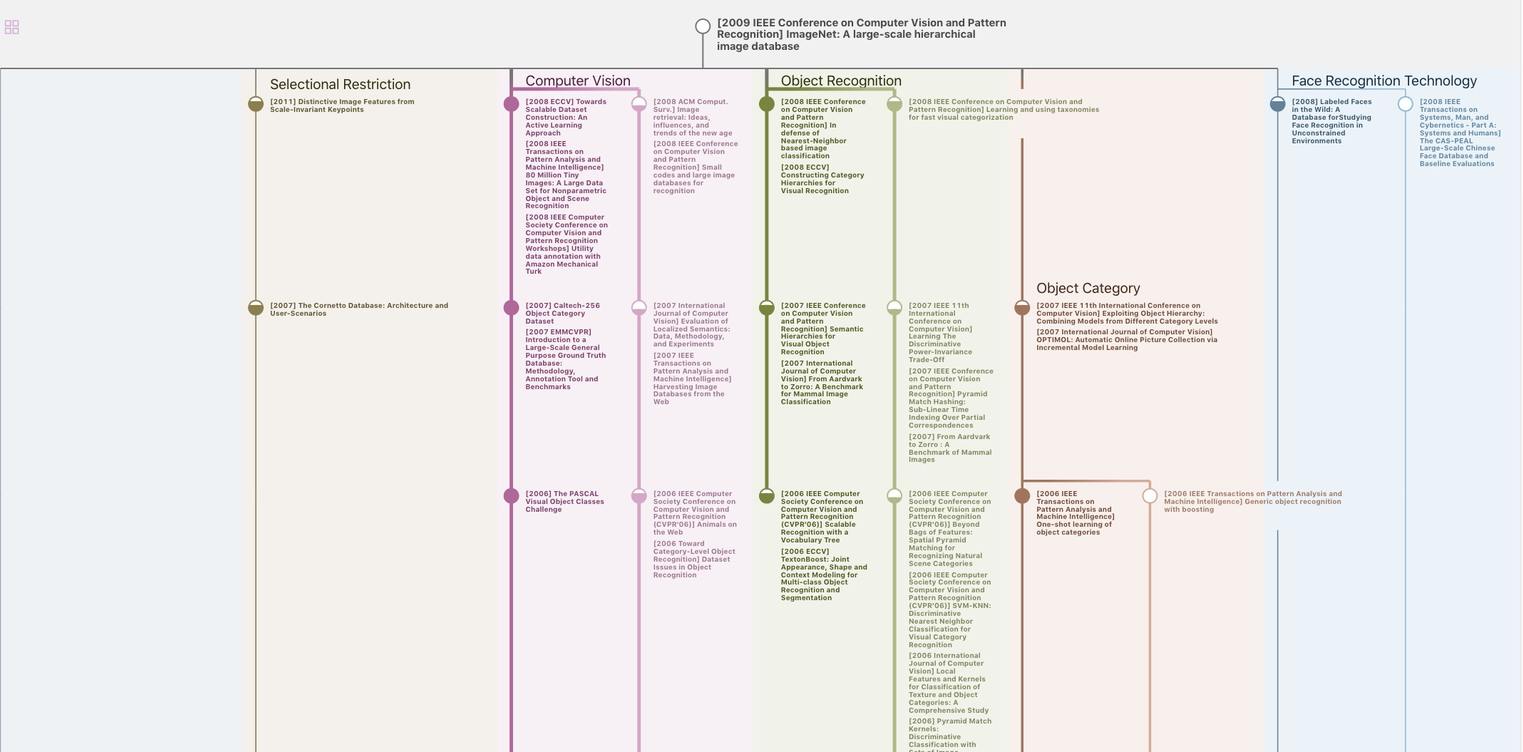
生成溯源树,研究论文发展脉络
Chat Paper
正在生成论文摘要