Training Generative Adversarial Networks with Adaptive Composite Gradient
DATA INTELLIGENCE(2024)
Abstract
The wide applications of Generative adversarial networks benefit from the successful training methods, guaranteeing that an object function converges to the local minimum. Nevertheless, designing an efficient and competitive training method is still a challenging task due to the cyclic behaviors of some gradient-based ways and the expensive computational cost of acquiring the Hessian matrix. To address this problem, we proposed the Adaptive Composite Gradients(ACG) method, linearly convergent in bilinear games under suitable settings. Theory analysis and toy-function experiments both suggest that our approach alleviates the cyclic behaviors and converges faster than recently proposed SOTA algorithms. The convergence speed of the ACG is improved by 33% than other methods. Our ACG method is a novel Semi-Gradient-Free algorithm that can reduce the computational cost of gradient and Hessian by utilizing the predictive information in future iterations. The mixture of Gaussians experiments and real-world digital image generative experiments show that our ACG method outperforms several existing technologies, illustrating the superiority and efficacy of our method.
MoreTranslated text
Key words
Generative adversarial networks,adaptive composite gradient,semi-gradient free,game theory,bilinear game
AI Read Science
Must-Reading Tree
Example
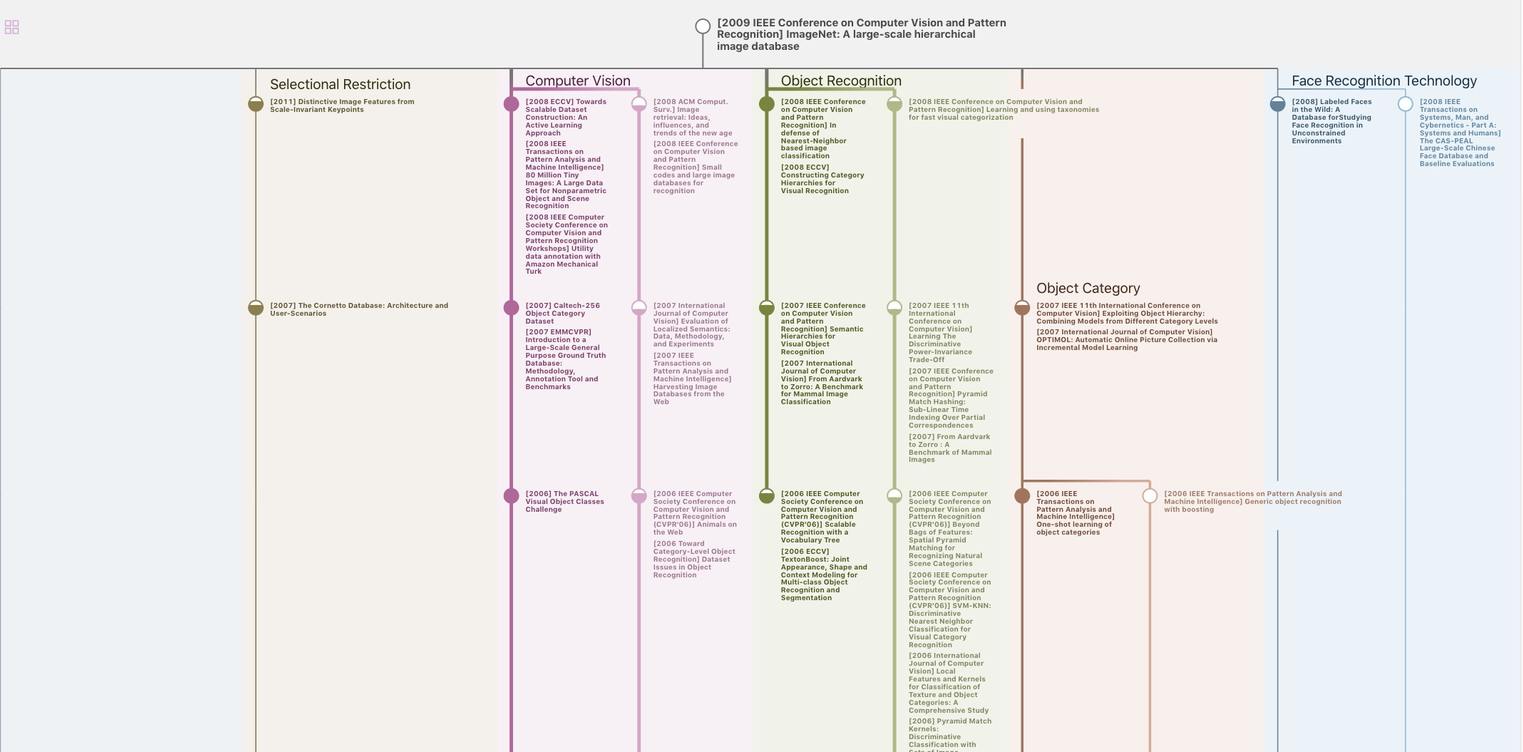
Generate MRT to find the research sequence of this paper
Chat Paper
Summary is being generated by the instructions you defined