A Blockchain-Enabled Federated Learning Model for Privacy Preservation: System Design
INFORMATION SECURITY AND PRIVACY, ACISP 2021(2021)
Abstract
Information Silo is a common problem in most industries, while Federated Learning (FL) as an emerging privacy-preservation technique aims to facilitate data sharing to solve the problem. It avoids data leakage by sharing the model gradient instead of the raw data. However, there are some challenges of FL, such as Single Point of Failure (SPoF), gradient privacy, and trust issues. This paper proposes a Homomorphic-integrated and blockchain-based FL model to address the above issues. It provides gradient privacy protection by employing Homomorphic, and uses a smart contract-based reputation scheme and an on/off-chain storage strategy to respectively solve FL trust and blockchain storage issues. In the end, it evaluates the proposed model by providing a qualitative privacy analysis and conducting preliminary experiments on model performance.
MoreTranslated text
Key words
Blockchain, Smart contract, Homomorphic encryption, Federated learning, Privacy protection, Data sharing
AI Read Science
Must-Reading Tree
Example
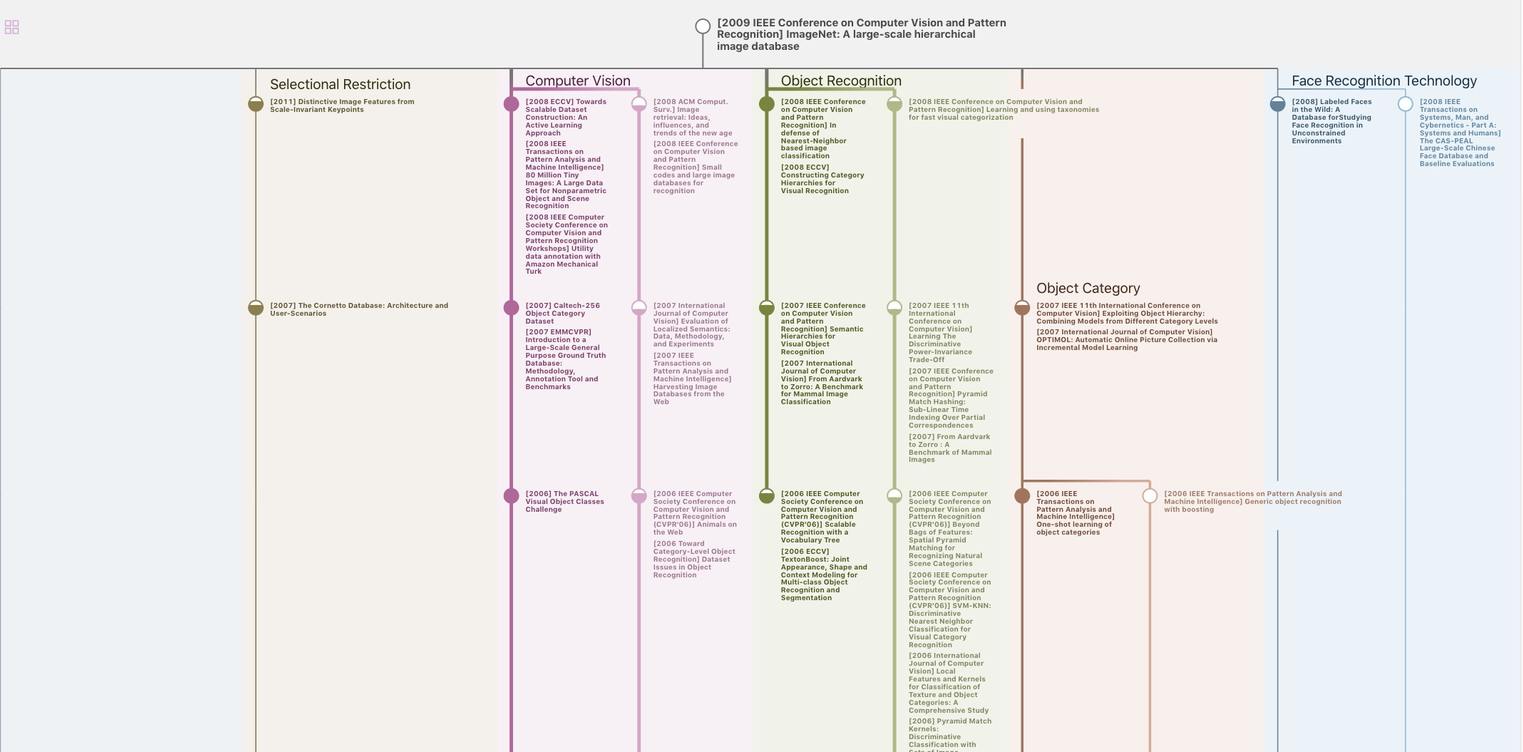
Generate MRT to find the research sequence of this paper
Chat Paper
Summary is being generated by the instructions you defined