Auxiliary-task learning for geographic data with autoregressive embeddings
Geographic Information Systems(2021)
摘要
ABSTRACTMachine learning is gaining popularity in a broad range of areas working with geographic data. Here, data often exhibit spatial effects, which can be difficult to learn for neural networks. We propose SXL, a method for embedding information on the autoregressive nature of spatial data directly into the learning process using auxiliary tasks. We utilize the local Moran's I, a measure of local spatial autocorrelation, to "nudge" the model to learn the direction and magnitude of local spatial effects, complementing learning of the primary task. We further introduce a novel expansion of Moran's I to multiple resolutions, capturing spatial interactions over longer and shorter distances simultaneously. The novel multi-resolution Moran's I can be constructed easily and offers seamless integration into existing machine learning frameworks. Over a range of experiments using real-world data, we highlight how our method consistently improves the training of neural networks in unsupervised and supervised learning tasks. In generative spatial modeling experiments, we propose a novel loss for auxiliary task GANs utilizing task uncertainty weights. SXL outperforms domain-specific spatial interpolation benchmarks, highlighting its potential for downstream applications.
更多查看译文
AI 理解论文
溯源树
样例
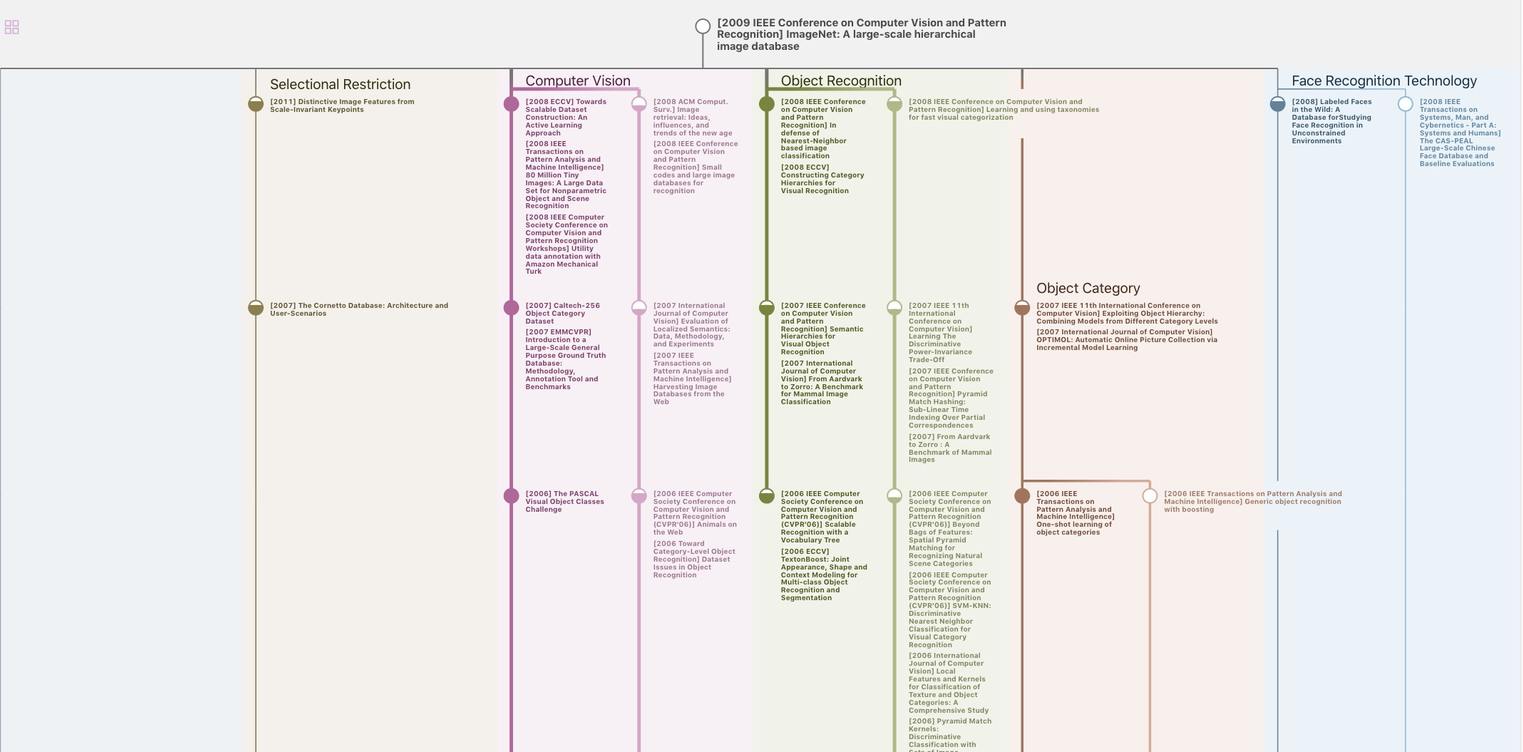
生成溯源树,研究论文发展脉络
Chat Paper
正在生成论文摘要