TrajNet: Outlier Detection in Vehicle Trajectory Data using Capsule Network based One-Shot Learning
Geographic Information Systems(2021)
摘要
ABSTRACTDiscovering anomalous trajectory becomes an essential task in various research and industrial domains in recent years. Unsupervised learning techniques have been used frequently to find an anomaly in trajectory. These methods fail to detect an outlier in highly correlated trajectory data. In general, the supervised approach is found to be more efficient compared to unsupervised learning in many domains. In this article, to detect outliers in vehicle trajectory data, a supervised learning technique is proposed called TrajNet. TrajNet work with a limited number of labelled trajectories exploiting capsule network-based one-shot learning. The experiments are conducted with a publicly available Geolife GPS trajectory dataset, and the preliminary results are very encouraging.
更多查看译文
AI 理解论文
溯源树
样例
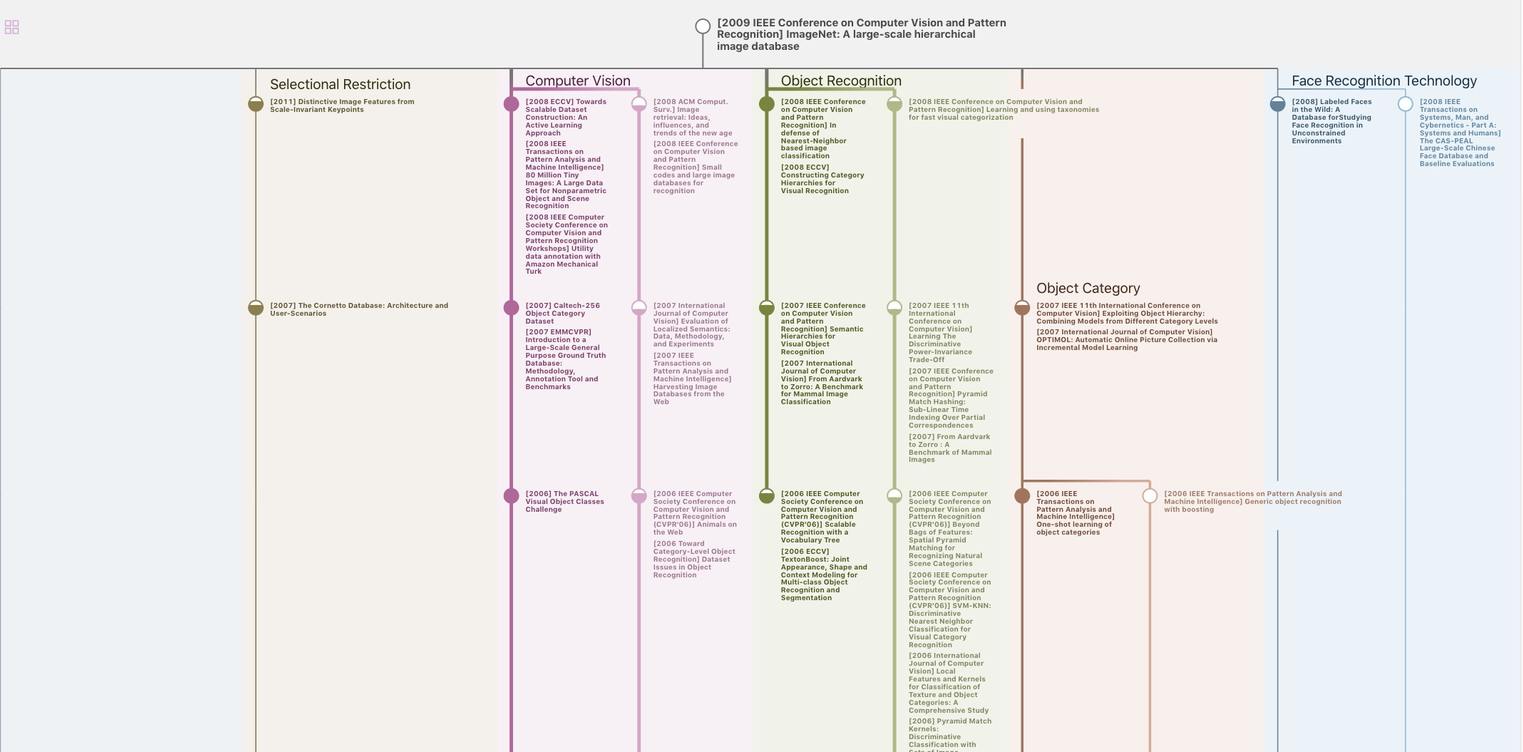
生成溯源树,研究论文发展脉络
Chat Paper
正在生成论文摘要