Deep Reinforcement Learning based control algorithms: Training and validation using the ROS Framework in CARLA Simulator for Self-Driving applications
2021 32ND IEEE INTELLIGENT VEHICLES SYMPOSIUM (IV)(2021)
摘要
This paper presents a Deep Reinforcement Learning (DRL) framework adapted and trained for Autonomous Vehicles (AVs) purposes. To do that, we propose a novel software architecture for training and validating DRL based control algorithms that exploits the concepts of standard communication in robotics using the Robot Operating System (ROS), the Docker approach to provide the system with portability, isolation and flexibility, and CARLA (CAR Learning to Act) as our hyper-realistic open-source simulation platform. First, the algorithm is introduced in the context of Self-Driving and DRL tasks. Second, we highlight the steps to merge the proposed algorithm with ROS, Docker and the CARLA simulator, as well as how the training stage is carried out to generate our own model, specifically designed for the AV paradigm. Finally, regarding our proposed validation architecture, the paper compares the trained model with other state-of-the-art traditional control approaches, demonstrating the full strength of our DL based control algorithm, as a preliminary stage before implementing it in our real-world autonomous electric car.
更多查看译文
关键词
Docker approach,DRL tasks,CARLA simulator,validation architecture,real-world autonomous electric car,ROS framework,self-driving applications,autonomous vehicles,software architecture,robot operating system,hyper-realistic open-source simulation,deep reinforcement learning
AI 理解论文
溯源树
样例
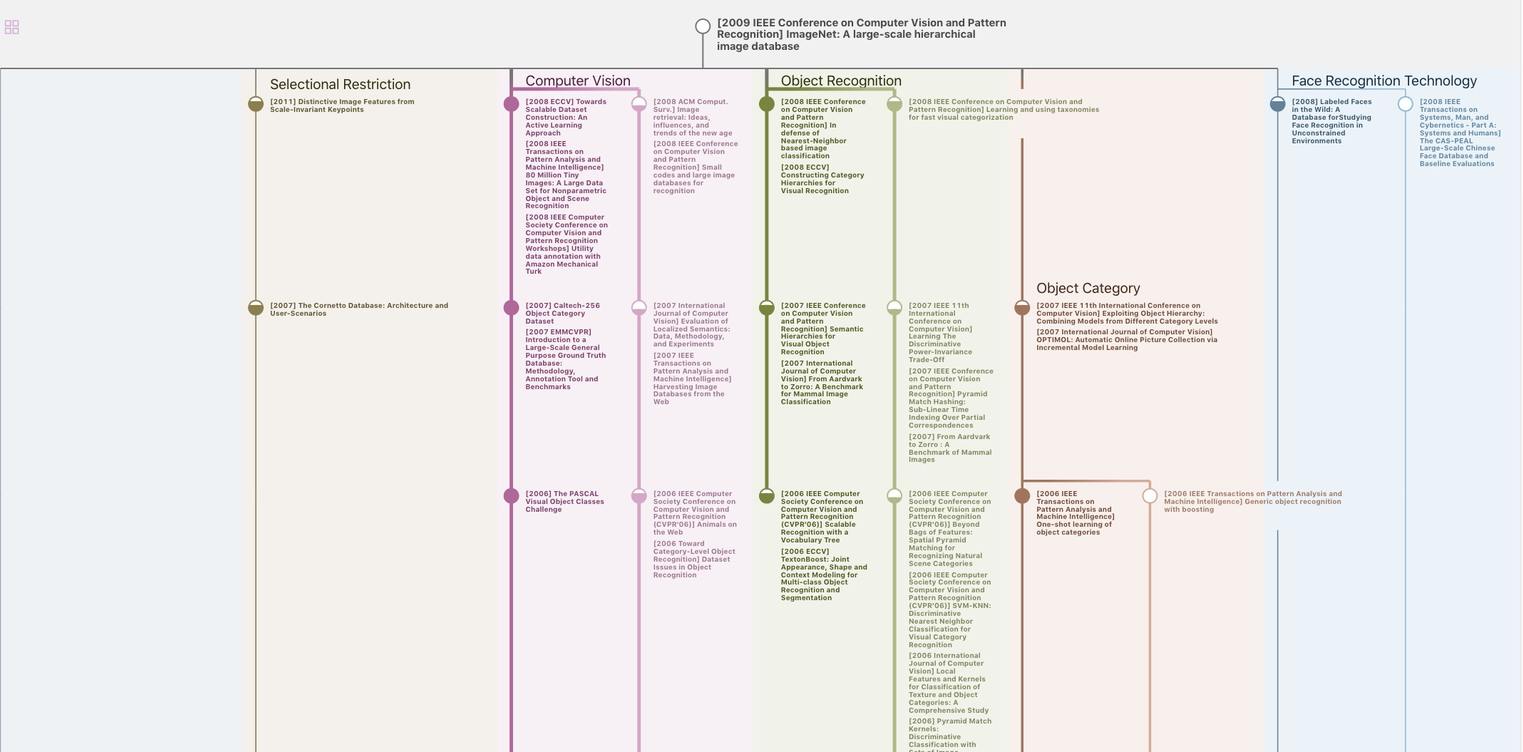
生成溯源树,研究论文发展脉络
Chat Paper
正在生成论文摘要