HackRL: Reinforcement learning with hierarchical attention for cross-graph knowledge fusion and collaborative reasoning
Knowledge-Based Systems(2021)
摘要
Reasoning aiming at inferring implicit facts over knowledge graphs (KGs) is a critical and fundamental task for various intelligent knowledge-based services. With multiple distributed and complementary KGs, the effective and efficient capture and fusion of knowledge from different KGs is becoming an increasingly important topic, which has not been well studied. To fill this gap, we propose to explore cross-KG relation paths with the anchor links identified by entity alignment for the knowledge fusion and collaborative reasoning of multiple KGs. To address the heterogeneity of different KGs, this paper proposes a novel reasoning model named HackRL based on the reinforcement learning framework, which incorporates the long short-term memory and hierarchical graph attention in the policy network to infer indicative cross-KG relation paths from the history trajectory and the heterogeneous environment for predicting corresponding relations. Meanwhile, an entity alignment-oriented representation learning method is utilized to embed different KGs into a unified vector space based on the anchor links to reduce the impact of distinct vector spaces, and two training mechanisms, action mask and retrain with sampled paths, are proposed to optimize the training process to learn more successful indicative paths. The proposed HackRL is validated on three cross-lingual datasets built from DBpedia on the link prediction and fact prediction tasks. Experimental results demonstrate that HackRL achieves better performance on most tasks than existing methods. This work provides an industrially-applicable framework for fusing distributed KGs to make better decisions.
更多查看译文
关键词
Knowledge fusion,Knowledge reasoning,Decision-making,Hierarchical graph attention,Reinforcement learning
AI 理解论文
溯源树
样例
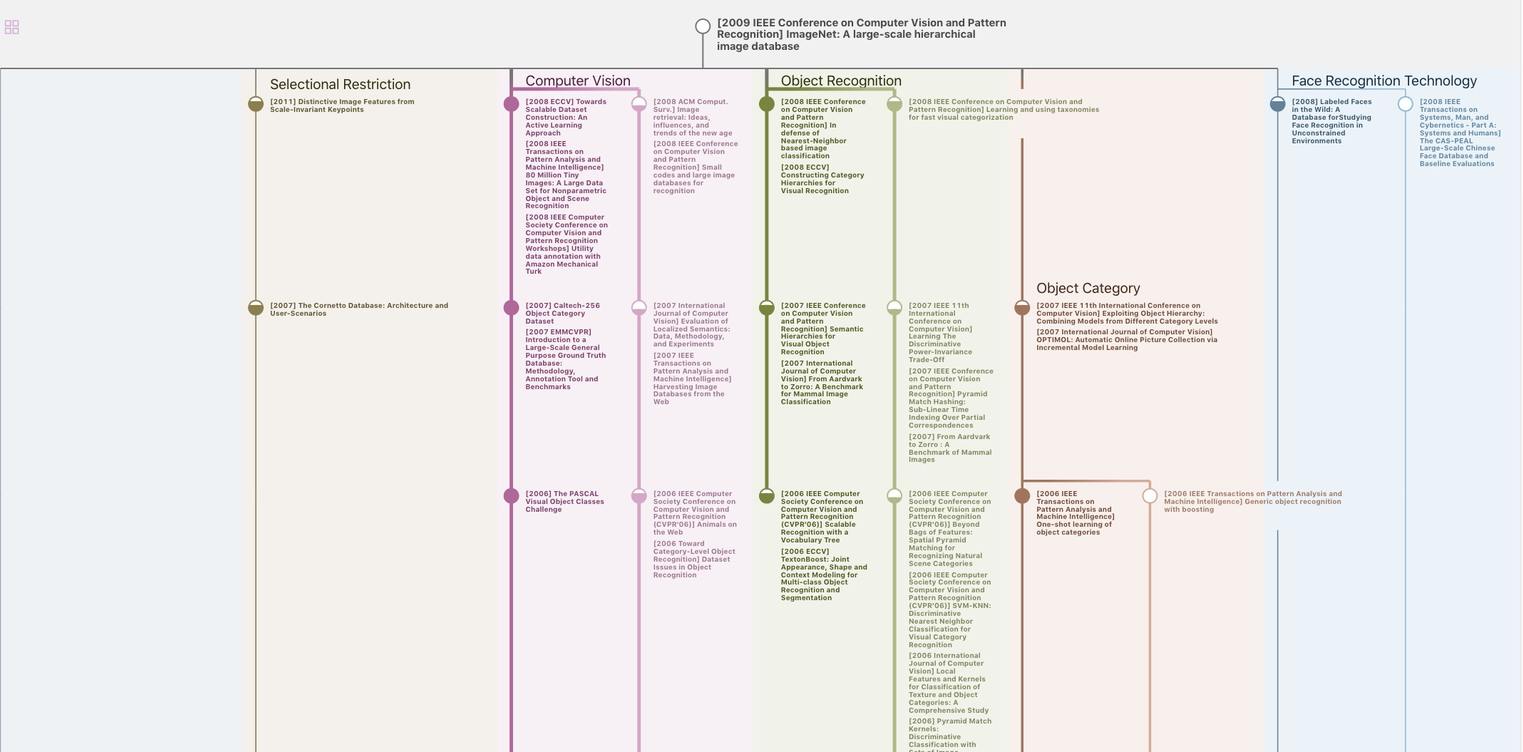
生成溯源树,研究论文发展脉络
Chat Paper
正在生成论文摘要