Unsupervised Spiking Instance Segmentation on Event Data Using STDP Features
arxiv(2022)
摘要
Spiking Neural Networks (SNN) and the field of Neuromorphic Engineering has brought about a paradigm shift in how to approach Machine Learning (ML) and Computer Vision (CV) problem. This paradigm shift comes from the adaption of event-based sensing and processing. An event-based vision sensor allows for sparse and asynchronous events to be produced that are dynamically related to the scene. Allowing not only the spatial information but a high-fidelity of temporal information to be captured. Meanwhile avoiding the extra overhead and redundancy of conventional high frame rate approaches. However, with this change in paradigm, many techniques from traditional CV and ML are not applicable to these event-based spatial-temporal visual streams. As such a limited number of recognition, detection and segmentation approaches exist. In this paper, we present a novel approach that can perform instance segmentation using just the weights of a Spike Time Dependent Plasticity trained Spiking Convolutional Neural Network that was trained for object recognition. This exploits the spatial and temporal aspects of the SpikeSEG network's internal feature representations adding this new discriminative capability. We highlight the new capability by successfully transforming a single class unsupervised network for face detection into a multi-person face recognition and instance segmentation network.
更多查看译文
关键词
Neuromorphic engineering,neuromorphic algorithms,SNN,STDP,computer vision,unsuperivsed learning,instance segmenation
AI 理解论文
溯源树
样例
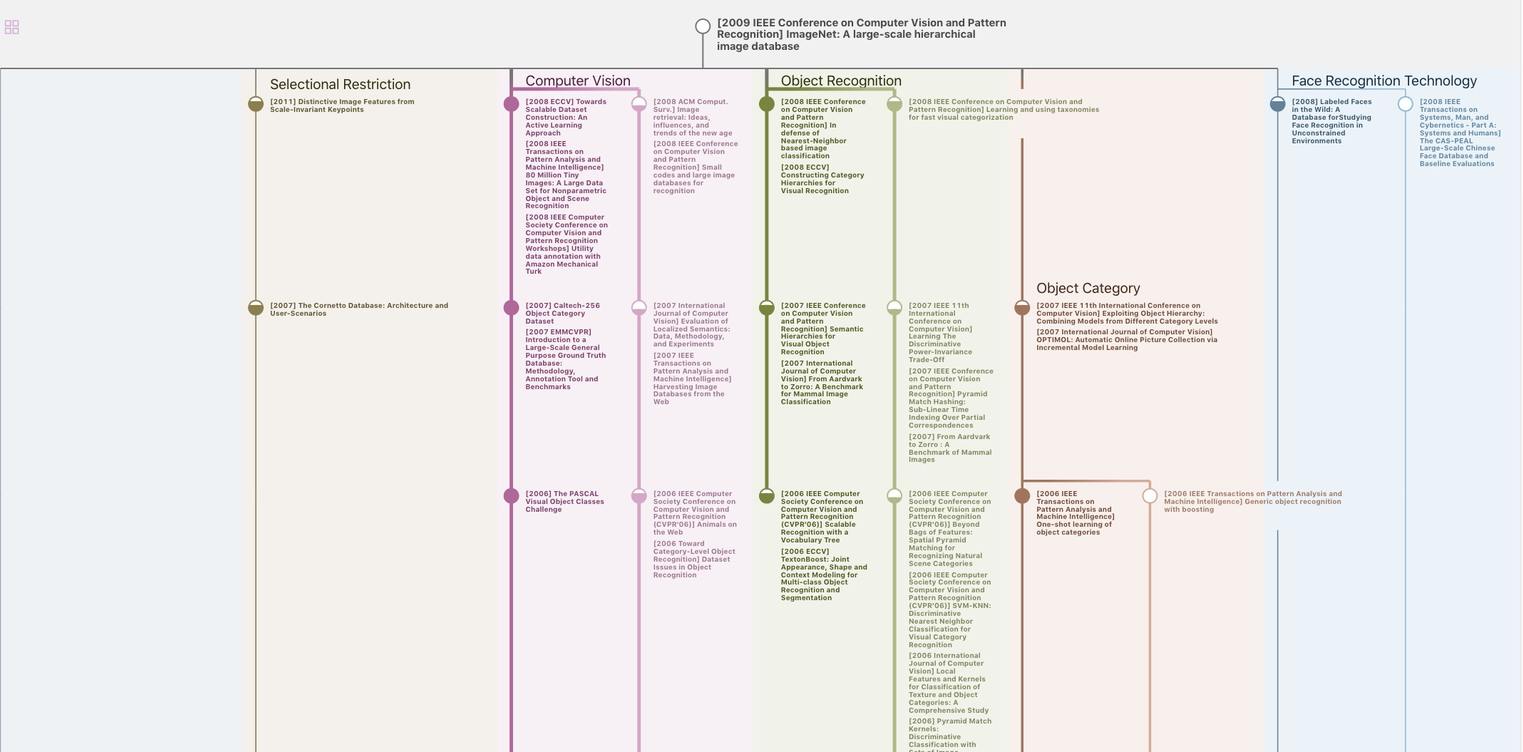
生成溯源树,研究论文发展脉络
Chat Paper
正在生成论文摘要