Realizable Learning is All You Need
arXiv (Cornell University)(2021)
摘要
The equivalence of realizable and agnostic learnability is a fundamental phenomenon in learning theory. With variants ranging from classical settings like PAC learning and regression to recent trends such as adversarially robust and private learning, it's surprising that we still lack a unified theory; traditional proofs of the equivalence tend to be disparate, and rely on strong model-specific assumptions like uniform convergence and sample compression. In this work, we give the first model-independent framework explaining the equivalence of realizable and agnostic learnability: a three-line blackbox reduction that simplifies, unifies, and extends our understanding across a wide variety of settings. This includes models with no known characterization of learnability such as learning with arbitrary distributional assumptions or general loss, as well as a host of other popular settings such as robust learning, partial learning, fair learning, and the statistical query model. More generally, we argue that the equivalence of realizable and agnostic learning is actually a special case of a broader phenomenon we call property generalization: any desirable property of a learning algorithm (e.g.\ noise tolerance, privacy, stability) that can be satisfied over finite hypothesis classes extends (possibly in some variation) to any learnable hypothesis class.
更多查看译文
关键词
Unsupervised Learning,Active Learning,Representation Learning,Transfer Learning
AI 理解论文
溯源树
样例
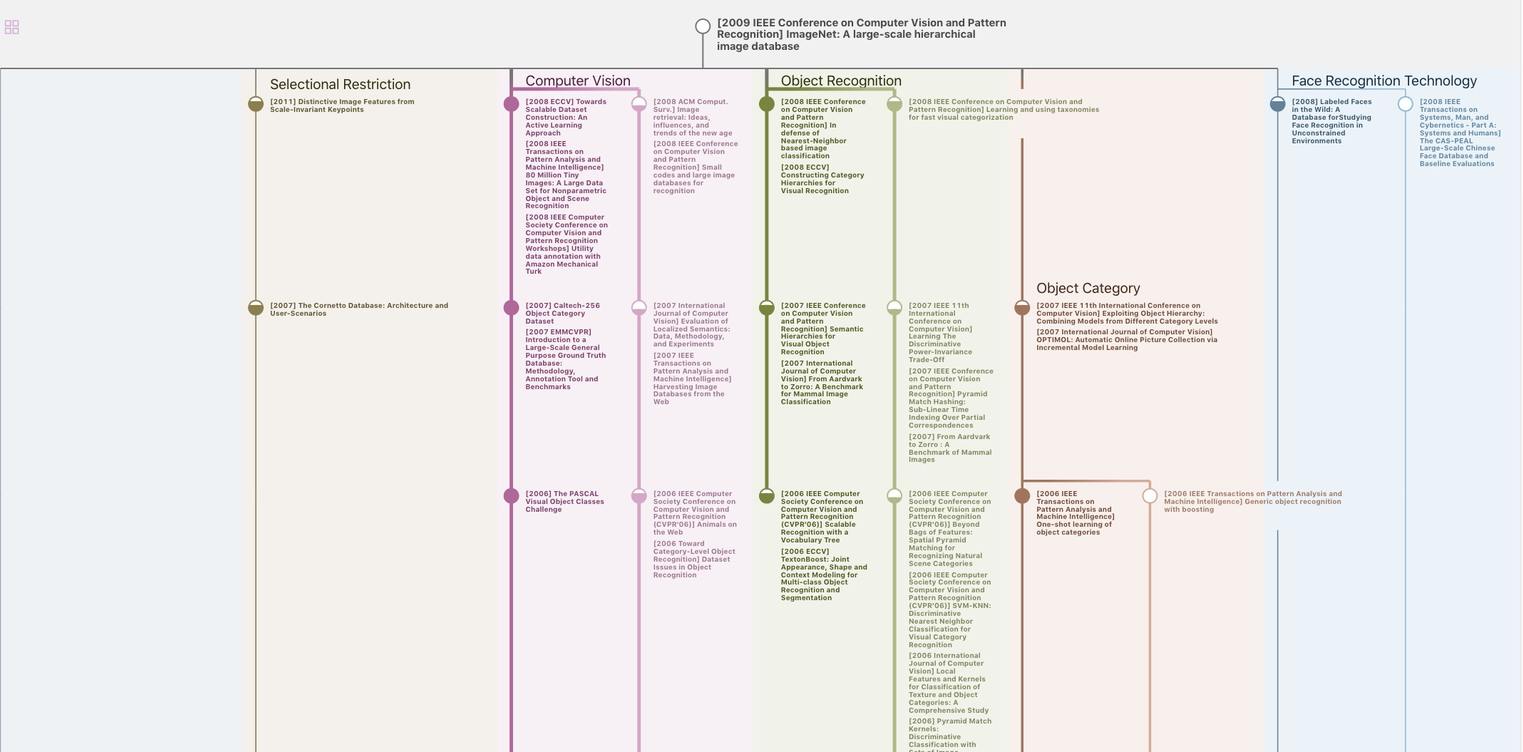
生成溯源树,研究论文发展脉络
Chat Paper
正在生成论文摘要