A Deep Learning Approach For Imbalanced Crash Data In Predicting Highway-Rail Grade Crossings Accidents
RELIABILITY ENGINEERING & SYSTEM SAFETY(2021)
摘要
Accurate accident prediction for highway-rail grade crossings (HRGCs) is critically important for assisting at-grade safety improvement decision making. Numerous machine-learning methods were developed focusing on predicting accidents and identifying contributing physical and operational characteristics. A more advanced deep learning-based model is explored as a more accurate means of predicting HRGC crashes compared to machine learning-based approaches. In particular, the prediction performance of the convolution neural network (CNN) model is compared to the most commonly used machine learning methods, such as decision tree (DT) and random forests (RF). A 19-year HRGCs data in North Dakota (ND) is used in this study. Training a machine learning model on an imbalanced data (e.g., unequal distribution of labeled data in accident and no-accident classes) introduce unique challenges for accurate prediction especially for minority class. In this paper, a resampling approach was used to address the imbalanced data issue. Various performance measurements are used to compare the models' prediction performance. The results indicate that resampling the imbalanced dataset significantly improves the recall rate. The results also show that the proposed deep learning-based approach which deepens the layer levels and adapts to the training dataset has better prediction performance compared to other machine learning-based methods.
更多查看译文
关键词
Deep learning, Machine learning, Safety, Prediction accuracy, Imbalanced data, Highway rail grade crossing
AI 理解论文
溯源树
样例
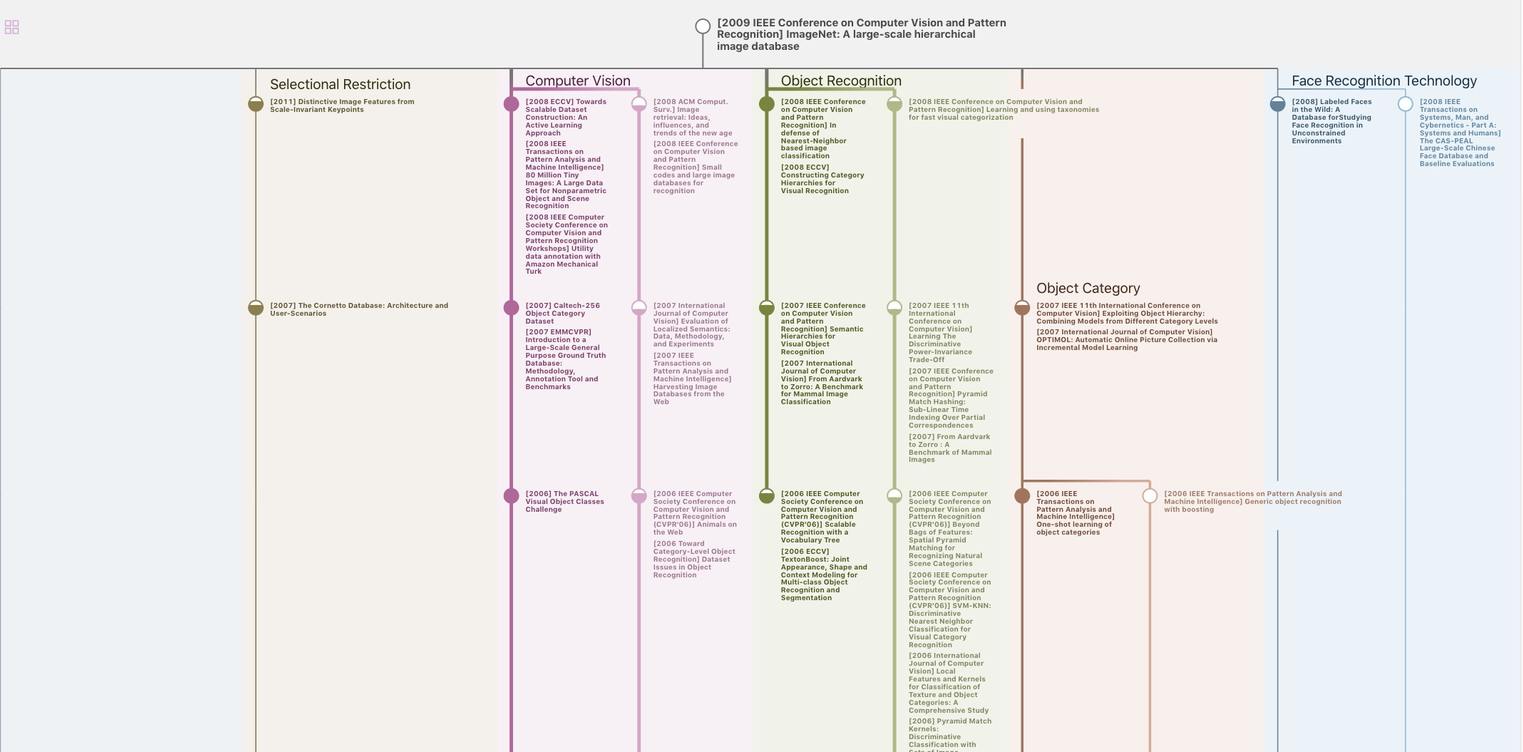
生成溯源树,研究论文发展脉络
Chat Paper
正在生成论文摘要