Weighted Multi-View Common Subspace Learning Method
PATTERN RECOGNITION LETTERS(2021)
摘要
How to use multi-view data effectively has become one of the challenging problems in the computer vision community. The existing multi-view learning methods are mainly based on common subspace learning which aim to explore the discriminative information between multi-view data and find its potential common subspace. Most of the existing multi-view subspace learning methods rely on the within-class scatter matrix and between-class scatter matrix while capturing the discriminative information of multiple views. However, these methods just roughly minimize the within-class distance and maximize the between-class distance, and do not make full use of the intra-view and inter-view information. To address this problem, we propose a weighted common subspace learning method, which can effectively adjust the contribution ratio of between-class and within-class information through a weighted parameter, so that an optimized common subspace can be obtained. And we use the maximum scatter difference criterion as the metric of inter-view and intra-view after projection. Extensive experiments on the public data sets show the superiority of this method . (c) 2021 Elsevier B.V. All rights reserved.
更多查看译文
关键词
Weighted parameter, Multi-view learning, Common subspace learning, Supervised learning
AI 理解论文
溯源树
样例
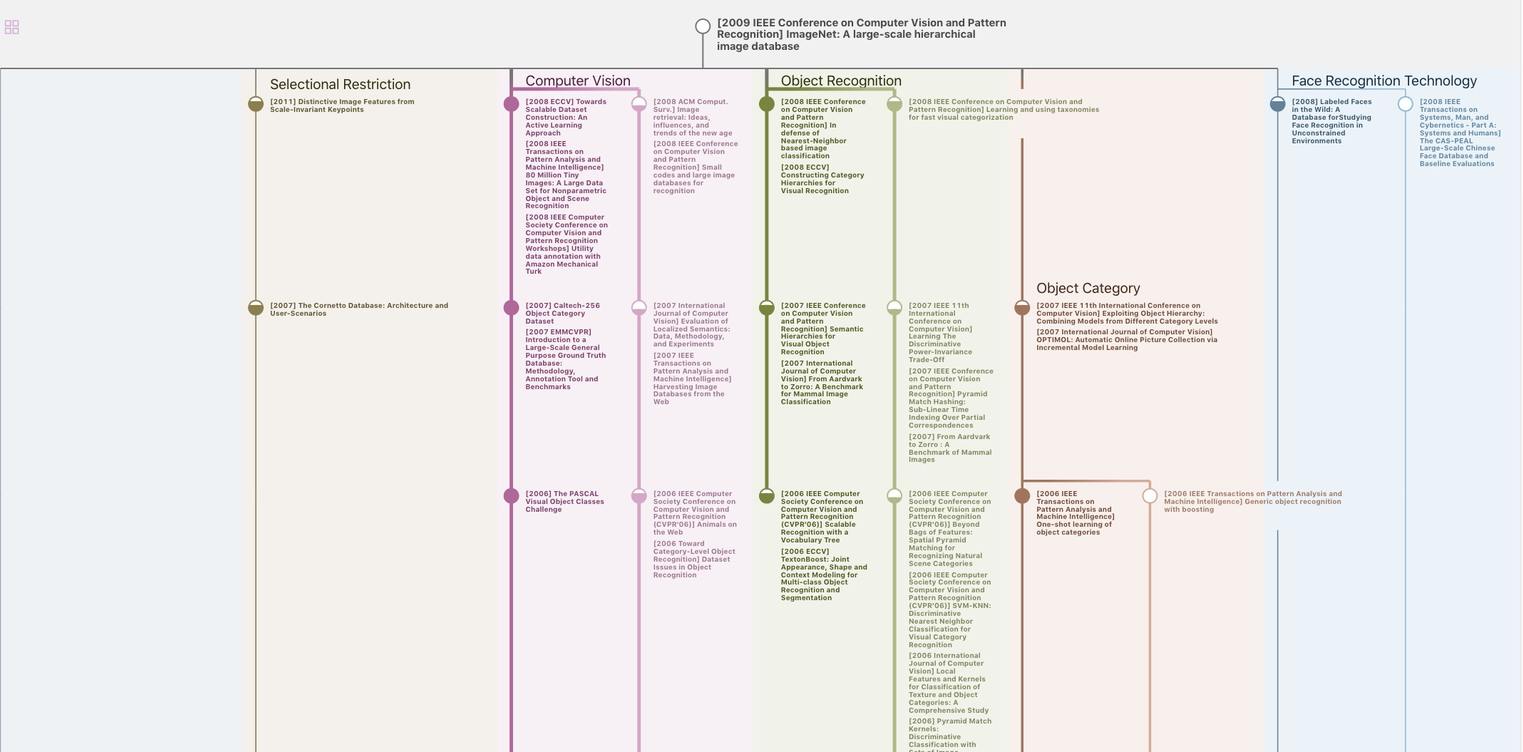
生成溯源树,研究论文发展脉络
Chat Paper
正在生成论文摘要